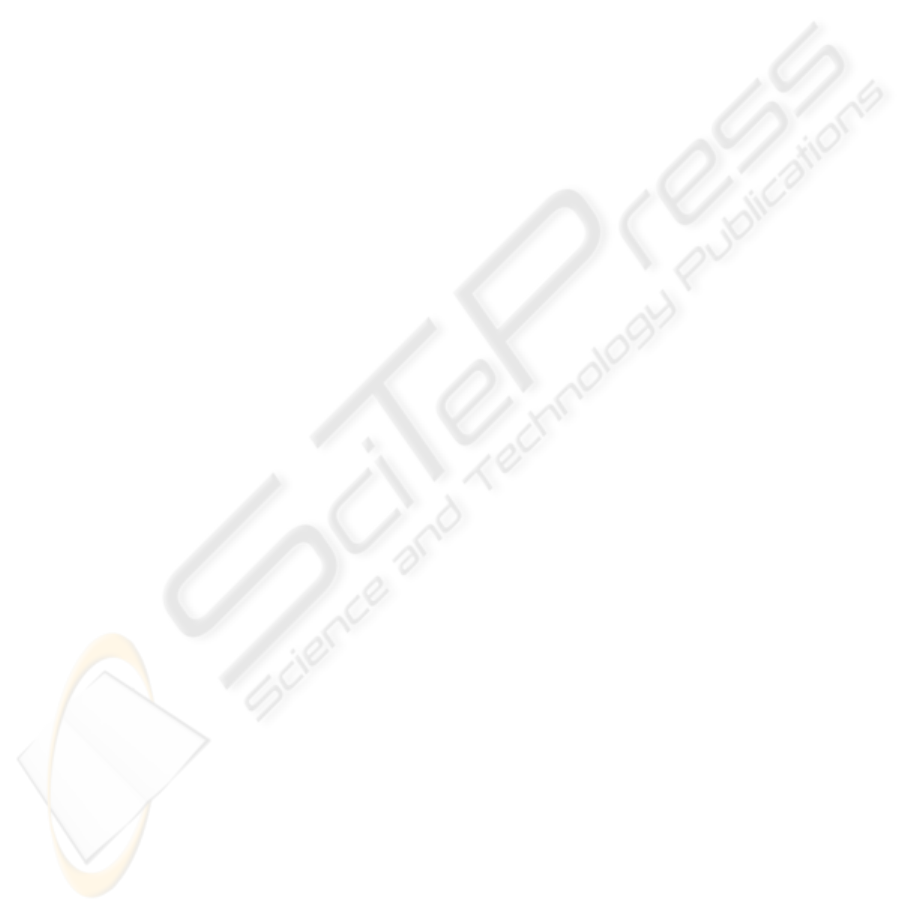
high-pass reconstruction error was decreased 1%–2%
with simulated data, and 5%–11% with real applica-
tion data depending on the reconstruction method. In
general, Wiener filtering improved the reconstruction
result for all the methods and data sets.
4 CONCLUSIONS
In this paper, surface reconstruction techniques were
studied in the context of surfaces with high frequency
variation. The effect of imaging noise to gradient
fields was also evaluated and a denoising approach
was proposed.
The experiments demonstrated that the Wiener fil-
tering based denoising of the gradient fields is useful
and applicable, if the power spectrum of the noise
is known. Minimization based surface reconstruc-
tion techniques, such as Poisson solver and Frankot-
Chellappa, are more robust against the noise with sim-
ulated and real application data compared to Hans-
son and Symmetric, which perform the integration
in Fourier domain with Wiener filtering. Hansson’s
method applies only one gradient field and was found
to be more sensitive to correct Wiener filter parame-
ters than Symmetric using two gradient fields. Pois-
son solvers with Neumann and periodic boundary
conditions provided the best results in total and high
frequency scale reconstruction, respectively. Neu-
mann boundary conditions provided correct surface
boundaries, whereas periodic boundary conditions
deteriorated the surface boundary with a non-periodic
pattern.
High frequency surfaces reconstructed using min-
imization based methods are is still not adequate for
some real applications, as they smoothen the surface
making, for example, roughness measurements in-
valid. Frankot-Chellappa and Poisson solvers provide
a robust and parameter free surface reconstruction in
many cases, but the methods have to developed fur-
ther for high frequencycontaining data. Recent devel-
opments in surface reconstruction, such as α surfaces,
M-estimators, regularization and diffusion, seem not
to provide improvement for these problems, as their
main effect is additional adaptive smoothing of the
surface, as noted in (Agrawal et al., 2006). Thus,
one problem in the reconstruction of high frequency
surfaces is in the definition of the function to be min-
imized. In future work, minimization constraints for
the surface reconstruction methodsneed to be studied.
Another approach would be to model the imaging sys-
tem more accurately, for example, using Wiener filter-
ing with SNR and PSF. In this work, only the effect of
the SNR was evaluated, and in future works also the
PSF has to be studied.
ACKNOWLEDGEMENTS
The authors gratefully appreciate the provided fund-
ing from European Regional Development Fund
(ERDF), Finnish Funding Agency for Technology
and Innovation (TEKES), Stora Enso, UPM, Metso,
and Future Printing Center (FPC).
REFERENCES
Agrawal, A., Raskar, R., and Chellappa, R. (2006). What
is the range of surface reconstructions from a gradient
field? In Proc. ECCV, pages 578–591.
Drbohlav, O. and Chantler, M. (2005). On optimal light
configurations in photometric stereo. In Proc. IEEE
ICCV.
Frankot, R. and Chellappa, R. (1988). A method for enforc-
ing integrability in shape from shading algorithms.
IEEE PAMI, 10(4):435–446.
Gonzalez, R. and Woods, R. (2002). Digital Image Process-
ing. Prentice Hall.
Hansson, P. and Johansson, P.-A. (2000). Topography and
reflectance analysis of paper surfaces using a photo-
metric stereo method. Opt. Eng., 39(9):2555–2561.
Horn, B. (1990). Height and gradient from shading. Int. J.
Comput. Vision, 5(1):37–75.
Karacali, B. and Snyder, W. (2004). Noise reduction in sur-
face reconstruction from a given gradient field. Inter-
national Journal of Computer Vision, 60:25–44.
Kuparinen, T., Kyrki, V., Mielikainen, J., and Toivanen,
P. (2007). On surface reconstruction from gradient
fields. In Proc. of IEEE ICIP, pages II 545–548.
Lambert, J. (2001). Photometry, or, on the measure and gra-
dations of light, colors, and shade: translation from
the Latin of photometria, sive, de mensura et gradibus
luminis, colorum et umbrae. Illuminating Engineering
Society of North America.
McGunnigle, G. and Chantler, M. (2003). Rough surface
description using photometric stereo. Measurement
Science and Technology, 14:699–709.
Noakes, L. and Kozera, R. (2003). Nonlinearities and noise
reduction in 3-source photometric stereo. Journal of
Mathematical Imaging and Vision, 18:119–127.
Simchony, T., Chellappa, R., and Shao, M. (1990). Direct
analytical methods for solving Poisson equations in
computer vision problems. IEEE PAMI, 12(5):435–
446.
Wei, T. and Klette, R. (2003). Depth recovery from noisy
gradient vector fields using regularization. In Proc. of
CAIP, pages 116–123.
Woodham, R. (1978). Photometric stereo. MIT A.I. Labo-
ratory Memo. No. 479.
VISAPP 2008 - International Conference on Computer Vision Theory and Applications
576