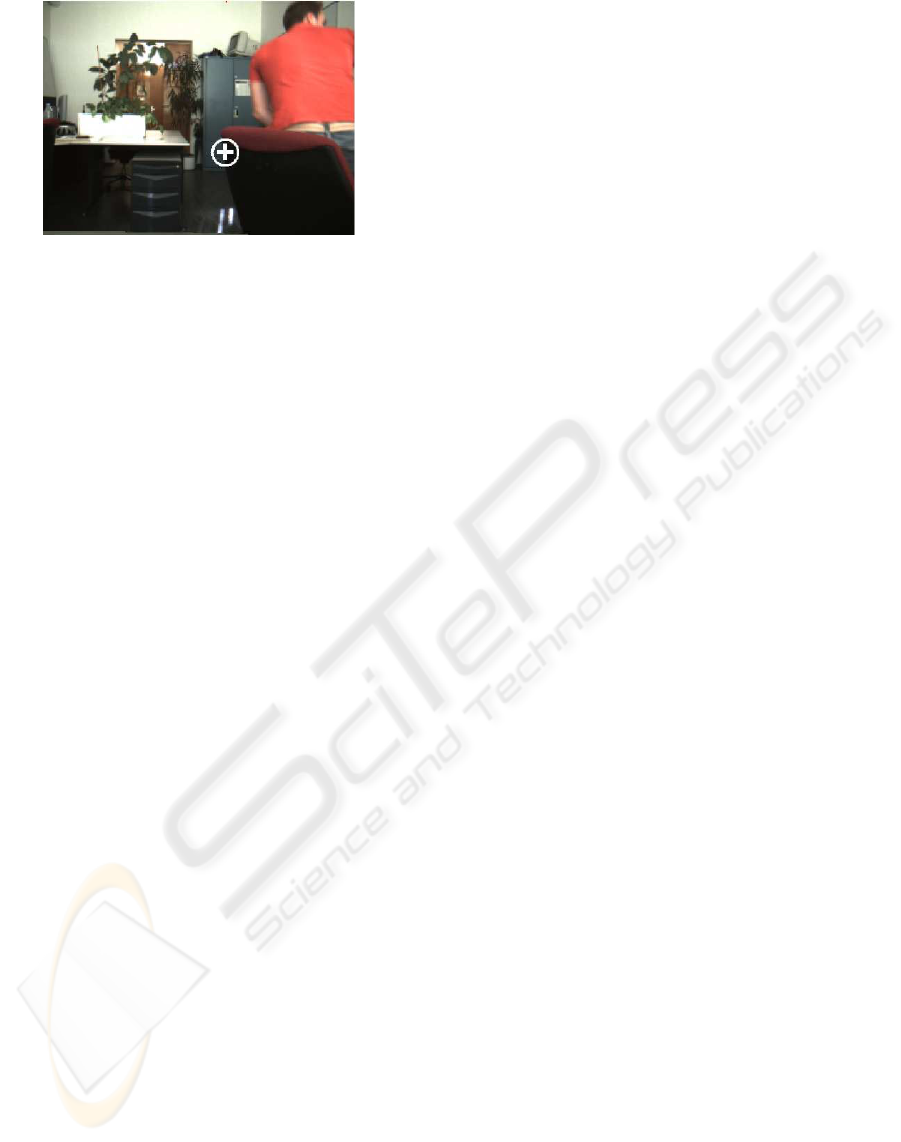
Figure 7: The detected FOA (indicated by a cross sur-
rounded by a cirle) is the closest object from the camera.
are promising, knowing that the computation of dis-
parity is a challenging task, specially in real time.
Some optimizations on the distance function, the
computation of the conspicuity map from the depth
gradient feature map, and the combination of the dif-
ferent feature maps will likely lead to better results.
5 CONCLUSIONS AND
PERSPECTIVES
In order to develop a mobility aid for blind people,
we have presented in this article a new approach to
detect salient parts in videos, using a depth based
FOA mechanism. Depth gradient is introduced as a
new feature map in an already known visual attention
model. We have proved that this feature map allows
for a better detection of objects of interest in video
sequences when using depth only, as proposed in pre-
vious works (Ouerhani and H¨ugli, 2000; Jost et al.,
2004). We have also proposed a specific distance
function, in order to take into account both hardware
limitations and user’s choices ; this allows the user
to decide if objects closer than his/her cane should be
detected or not. The results we obtained with this sim-
ple framework are promising, and some optimizations
such as a more realistic distance function or the deter-
mination of optimal coefficients in Equation 4, should
lead to even better results.
Ongoing and future work concerns the following.
First, we will integrate some usual feature maps like
colour opposition, flicker, or motion, to ensure that
the depth gradient brings useful information in a vi-
sual attention model. The presented method will then
be integrated in the See ColOr framework. It is partic-
ularly important to decide how the salient area will be
sonified ; we do not want the user to be confused by
similar sounds meaning completely different things.
Once this will be done, the system will finally be eval-
uated by blind and blindfolded users.
ACKNOWLEDGEMENTS
We gratefully acknowledge the financial support of
the Swiss Hasler Foundation.
REFERENCES
Bay, H., Tuytelaars, T., and Gool, L. V. (2006). Surf:
Speeded up robust features. In Proceedings of the 9th
European Conference on Computer Vision, pages 7–
13.
Bologna, G., Deville, B., Pun, T., and Vinckenbosch, M.
(2007a). Identifying major components of pictures by
audio encoding of colors. In IWINAC2007, 2nd. In-
ternational Work-conference on the Interplay between
Natural and Artificial Computation.
Bologna, G., Deville, B., Pun, T., and Vinckenbosch, M.
(2007b). Transforming 3d coloured pixels into mu-
sical instrument notes for vision substitution applica-
tions. EURASIP Journal on Image and Video Process-
ing.
Hoffman, D. and Singh, M. (1997). Salience of visual parts.
Cognition, 63:29–78.
Itti, L., Koch, C., and Niebur, E. (1998). A model of
saliency-based visual attention for rapid scene anal-
ysis. IEEE Transactions on Pattern Analysis and
Machcine Intelligence, 20(11):1254–1259.
Jost, T., Ouerhani, N., von Wartburg, R., M¨uri, R., and
H¨ugli, H. (2004). Contribution of depth to visual at-
tention: comparison of a computer model and human.
In Early cognitive vision workshop, Isle of Skye, Scot-
land.
Kadir, T. and Brady, M. (2001). Scale, saliency and im-
age description. International Journal of Computer
Vision, 45(2):83–105.
Landragin, F. (2004). Saillance physique et saillance cogni-
tive. Cognition, Reprsentation, Langage, 2(2).
Lowe, D. (1999). Object recognition from local scale-
invariant features. In Seventh International Confer-
ence on Computer Vision (ICCV’99), volume 2.
Maki, A., Nordlund, P., and Eklundh, J. (1996). A computa-
tional model of depth-based attention. In Proceedings
of the International Conference on Pattern Recogni-
tion (ICPR ’96).
Milanese, R., Gil, S., and Pun, T. (1995). Attentive mech-
anism for dynamic and static scene analysis. Optical
Engineering, 34(8):2428–2434.
Ouerhani, N. and H¨ugli, H. (2000). Computing visual at-
tention from scene depth. In Proceedings of the 15th
International Conference on Pattern Recognition, vol-
ume 1, pages 375–378.
DEPTH-BASED DETECTION OF SALIENT MOVING OBJECTS IN SONIFIED VIDEOS FOR BLIND USERS
439