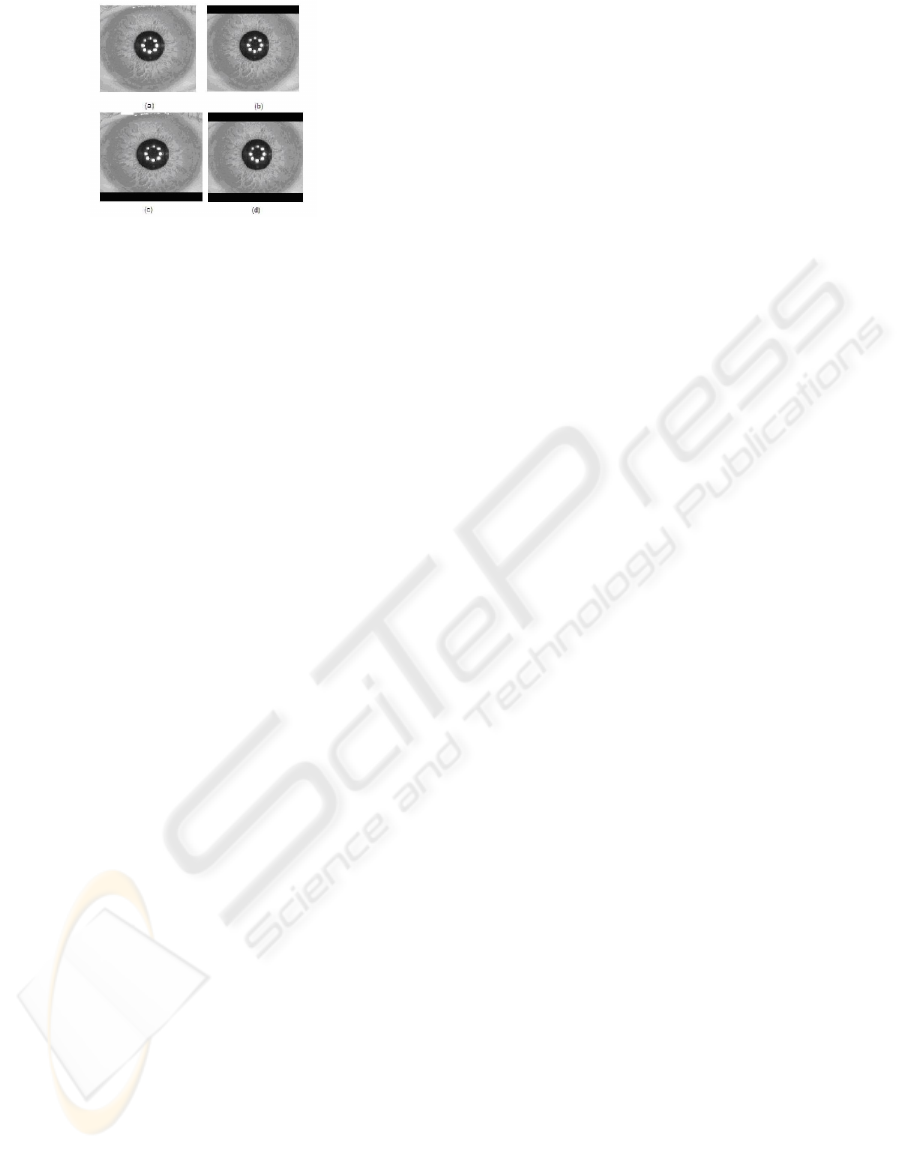
Figure 2: Iris images (a) without occlusions and with
occlusions on (b) top (c) bottom and (d) top and bottom
regions.
In the second set of experiments, the iris images
are occluded from the top region of the iris. The
recognition rates for various occlusion percentages
are presented in Table 2. The data on this table
shows that some of the partitioned PCA approaches
slightly increase the performance of the holistic
PCA. The recognition rate of the proposed approach
is significantly increased compared to holistic
counterparts.
The third and the fourth set of experiments also
demonstrate that the proposed approach by
combining the outputs of individual regions achieves
better performance compared to the performance of
the holistic PCA and partitioned PCA methods.
In general, the experiments using holistic PCA,
partitioned PCA and the proposed approach without
occlusions demonstrate that the proposed approach
achieves a significant improvement for the
recognition of iris images (from 84% to 96%). On
the other hand, for the iris images with occlusions on
either top or bottom of the iris, the recognition rates
demonstrate that the proposed approach is not
sensitive up to 5% occlusions. The results obtained
by the proposed approach with 5% occlusions on
either top or bottom of the irises are equivalent to
the results obtained by holistic PCA without
occlusions.
5 CONCLUSIONS
A simple and efficient approach for reducing the
effect of partial occlusions and improving the
recognition performance of the iris images is
presented. The experiments performed using holistic
PCA, partitioned PCA and the proposed approach
without occlusions demonstrate that the proposed
approach achieves a significant improvement for the
recognition of iris images. It can also be stated that
the proposed approach is not sensitive up to 5%
occlusions on top or bottom regions of the iris
images compared to the holistic PCA method
without occlusions.
ACKNOWLEDGEMENTS
Portions of the research in this paper use CASIA
IrisV3 collected by the Chinese Academy of
Sciences’ Institute of Automation (CASIA).
REFERENCES
CASIA-IrisV3, http://www.cbsr.ia.ac.cn/IrisDatabase.htm
Chu, C.T., Chen, C.H., 2005. High Performance Iris
Recognition Based on LDA and LPCC. In ICTAI’05,
Proceedings of the 17
th
IEEE International
Conference on Tools with Artificial Intelligence, Hong
Kong.
Cui, J., Huang, J., Tan, T., Sun, Z., 2004. An iris image
synthesis method based on PCA and super-resolution.
In ICPR’04, 17th International Conference on Pattern
Recognition, Cambridge, UK.
Daugman, J., 2006. Probing The Uniqueness and
Randomness of IrisCodes: Results from 200 Billion
Iris Pair Comparisons. Proceedings of The IEEE,
Vol.94, No.11, pp.1927-1935.
Ho, T., Hull, J., Srihari, S., 1994. Decision combination in
multiple classifier systems, IEEE Transactions on
Pattern Analysis and Machine Intelligence, Vol.16,
pp. 66-75.
Kirby, M. and Sirovich, L., 1990. Application of the
Karhunen-Loeve Procedure for the Characterization of
Human Faces. In IEEE Trans. Pattern Analysis and
Machine Intelligence. Vol.12, pp. 103-107.
Kittler, J., Hatef, M., Duin, R.P.W., Matas, J., 1998. On
Combining Classifiers, IEEE Trans. Pattern Analysis
and Machine Intelligence, Vol.20, No.3, pp.226-239.
Ma, L., Tan T., Wang Y., Zhang D., 2004. Efficient iris
recognition by characterizing key local variations. In
IEEE Transactions on Image Processing. Vol.13,
no.6, pp.739-750.
Turk, M. and Pentland, A., 1991. Eigenfaces for
Recognition. In Journal of Cognitive Neuroscience.
Vol.3, pp.71-86.
Toygar, Ö. and Acan, A., 2004. Multiple Classifier
Implementation of a Divide-and-Conquer Approach
Using Appearance-Based Statistical Methods for Face
Recognition. In Pattern Recognition Letters, Vol.25,
pp. 1421-1430.
VISAPP 2008 - International Conference on Computer Vision Theory and Applications
546