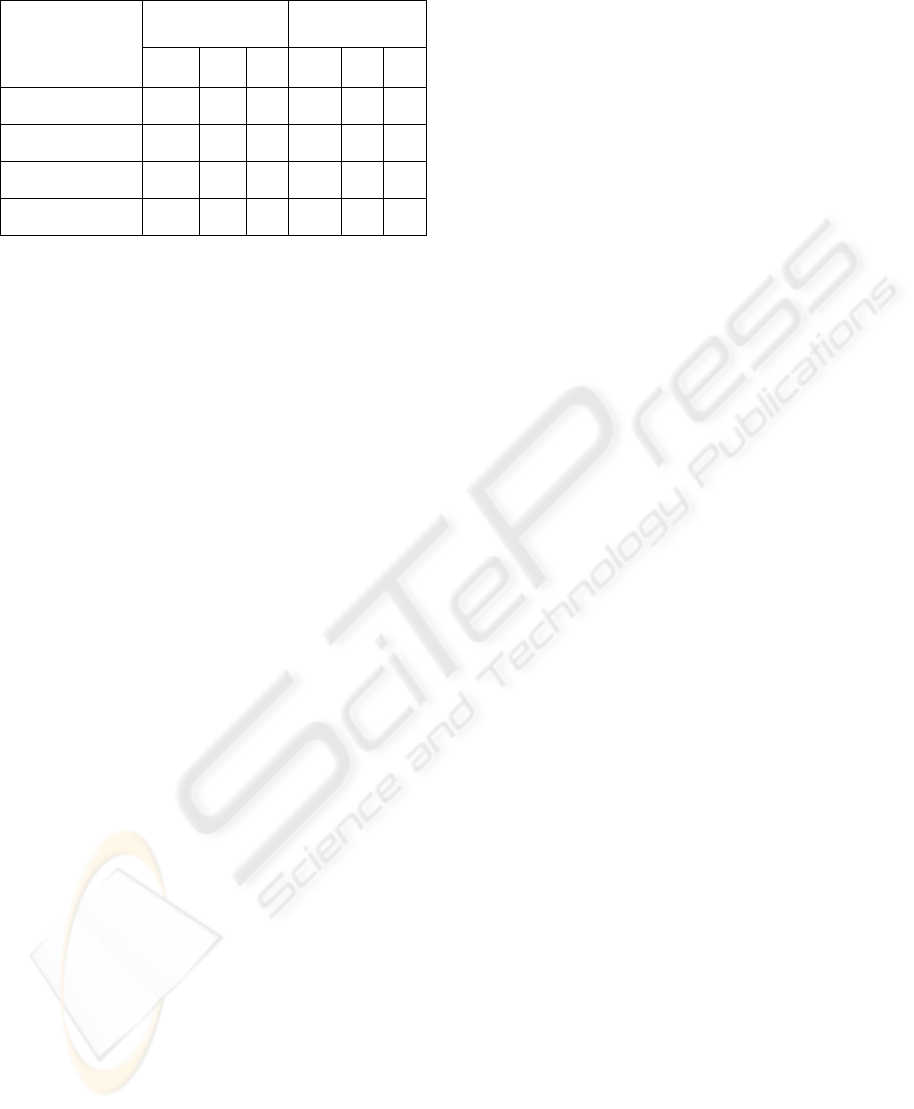
Table 2: Performance results for hard cut (H), dissolve (D)
and fades (F) detection.
The results with the proposed method are presented
in Table 2. From Tables 1 and 2 it can be observed
that hard cut detection ratio is 99.67% while dissolve
detection ratio is 79.3%. Only two fade-outs are
present in the documentary sequence and the two are
detected correctly. Miss detection ratio for hard cut
is 3.32% and for dissolve it is 20.22%. False
detection ratio for hard cut and dissolve are 21% and
9.2% respectively. Results indicate that the
performance of hard cut detection is very high and in
most cases, dissolve detection is also correct. Our
observation is that false detections occur in closeup
shots. Specifically, these can be observed very
prominently in the basketball sequence where
players are showed closely while moving fast.
For the qualitative evaluation of the proposed
method, we refer the results presented with various
algorithms in (Lienhart, 1999). In (Lienhart, 1999),
Lienhart evaluated the best known algorithms and
presented improvements to them. With these
improvements, Lienhart achieved correct and false
detection ratios for hard cut as 95% and 5% and for
dissolve as 80% and 20% respectively. Even though,
out test data is not as big as that Lienhart used, the
results do bring out the merits of our method. As we
have selected by carefully considering the various
types of camera actions and events, the results can
be considered as consistent over a large data set as
well.
4 CONCLUSIONS
This paper presents a novel algorithm for shot
boundary detection using Gaussian mixture models.
Performance of the algorithm is verified by testing
with different types of test sequences. Results
indicate that proposed method can handle zooming,
lighting change, and fast moving scenes effectively.
However, the performance degrades with closeup
shots with fast moving camera action or activity.
These are due to the delay in updating the GMMs.
Handling of these types of problems for reducing the
false detection is considered in part of our on going
work.
REFERENCES
Lienhart, R., 1999. Comparison of automatic shot
boundary detection algorithms. In proc. IS &T/SPIE
Storage and Retrieval for Image and Video Databases
VII. vol. 3656, pp 290-321.
Zhang, H., Kankanhalli, A., Smoliar, S.W., 1995.
Automatic partitioning of full-motion video.
Multimedia Systems. vol. 1, pp. 533-544.
Huang, X., Wei, G., Petrushin, V.A., 2003. Shot boundary
detection and high-level feature extraction for the
TREC video evaluation 2003. NIST TRECVID 2003.
Boussaid, L., Mtibaa, A., Abid, M., Paindavoine, M.,
2007. A real time shot cut detector: Hardware
implementation. Computer Standards & Interfaces.
Vol. 29, Issue 3, Pages 335-342.
Tardini, G., Grana, C., Marchi, R., Cucchiara, R., 2005.
Shot detection and motion analysis for automatic
MPEG-7 annotation for sports video. In Int. Conf. on
Image Analysis and Processing, pp. 653-660.
Zabih, R., Miller, J., Mai, K., 1995. Feature-based
algorithms for detecting and classifying scene breaks.
In Proc. ACM on Multimedia. pp. 189–200,
Bruyne, S.D., Wolf, K.D., Neve, W.D., Verhoeve, P.,
Walle, R.V.D., 2006. Shot boundary detection using
macroblock prediction type information. In Workshop
on Image Analysis for Multimedia Interactive
Services. pp. 205-208.
Fang, H., Jiang, J., Feng, Y., 2006. A fuzzy logic approach
for detection of video shot boundaries. Pattern
Recognition. Vol. 39, , Pages 2092-2100.
Stauffer, C., Grimson, W.E.L, 1999. Adaptive background
mixture models for real-time tracking. In Proc. of Int.
Conf. on Computer Vision and Pattern Recognition.
Vol. 2, pp.246-252.
Mo,X.,Wilson,R.,2004. Video modeling and segmentation
using Gaussian mixture models. In Proc. of Int. Conf.
on Pattern Recognition, Vol. 3, pp 854-857.
Test sequence
Correct
Detection
False Detection
H D F H D F
News 44 0 0 0 0 0
Documentary 87 8 2 10 2 2
Soccer 41 24 0 8 2 0
Basket-ball 90 37 0 39 4 7
VISAPP 2008 - International Conference on Computer Vision Theory and Applications
550