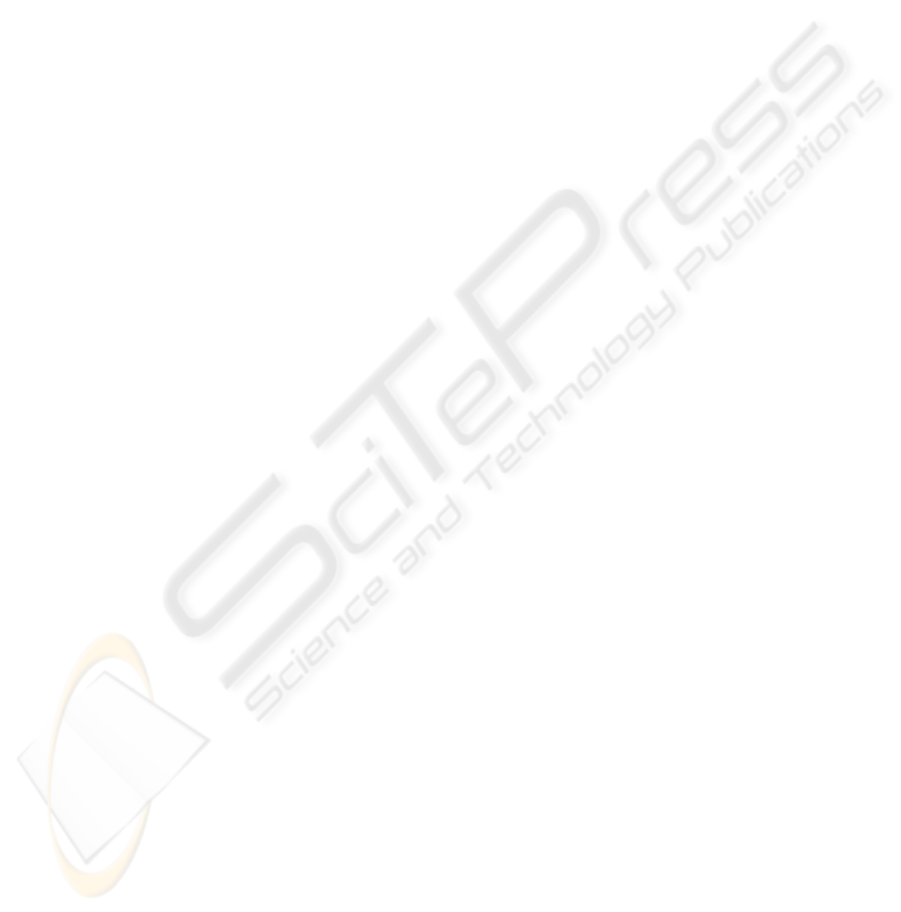
cleanse them and keep them firm, and then drains
away through the lymphatic system. Cancer cells
may drain into nearby lymph nodes, which are bean-
shaped structures that help fight against infection.
Lymph nodes are the filters along the lymphatic sys-
tem. Since the lymph nodes function to filter out
harmful cells, in particular cancer cells, this is a log-
ical place to look for cancer cells that have escaped
the original tumor and are seeking to metastasise to a
distal location. Lymph node dissection prevents can-
cer cells from further growth. The number of involved
lymph nodes strongly predicts the nature of the cancer
and the type of treatment needed to fight it. For these
reasons, it is considered by clinicians to be of great
importance to assess lymph nodes in the management
of cancer.
In the case of colorectal cancer, the lymph nodes
appear as dark small blobs on magnetic resonance
(MR) images and are distributed mostly in the
mesorectum, a fatty tissue that envelops the colorec-
tum. In practice, lymph nodes are characterised by
human vision using explicit properties, such as size
and shape of lymph nodes. We are not aware of any
method of image analysis approach of colorectal im-
ages that makes this subjective approach more scien-
tific.
In this paper we develop methods to aid precise
staging and estimation of the circumferential resec-
tion margin of colorectal cancers, namely detection
and characterisation of a lymph node. The contribu-
tion of the proposed methods can be divided into two
categories: modeling an intensity probability density
function (PDF) and segmentation of non-trivial MR
images.
Section 2 describes the methods we propose; char-
acterising a lymph node by building a PDF of inten-
sity of the mesorectal fascia. For this purpose, we
need to segment the mesorectal fascia on non-trivial
images as well as lymph node candidates. Our ex-
periments are presented in section 3, and section 4
concludes and discusses our work.
2 METHODS
Our methods include lymph node detection and char-
acterisation; the two unrelated categories are com-
bined here.
We attempt to detect and analyse lymph nodes
from 3D MR colorectal images, taken at our lo-
cal hospitals by GE 1.5T magnetic resonance imag-
ing scanners with parameters of TE = 120ms, TR =
6500ms. Each 3D dataset comprises a set of 23 to 30
slices each corresponding to a 3mm to 5mm slab of
tissue.
Lymph nodes generally appear as small dark
blobs, of a distinctively low intensity compared to
the surrounding fat which has high intensity. How-
ever, lymph nodes may be heterogeneous in intensity:
such heterogeneity is generally considered evidence
for a lymph node being affected by cancer. Further-
more, since lymph nodes are small and the sampling
in MRI is large compared to their size, the partial vol-
ume effect (PVE, in which multiple tissue structures
are present in a single voxel) has a substantial im-
pact on the intensities at locations that correspond to
lymph nodes. Also, the generally bright background
is punctuated with folds in the fat, which compli-
cate it appearance. The dark blobs corresponding to
lymph appear locally similar to blood vessels, espe-
cially when viewed in a single two dimensional slide
image. We can eliminate blood vessels on the basis of
their three dimensional shapes (extended over several
slices). The consecutive slices are registered and ex-
amined if they extend over several slices; if so, they
are more likely to be blood vessels. As a result, we
may estimate locations of some possible lymph node
candidates. For each candidate we test our methods
described in this section.
Current characterisations of lymph nodes stress ei-
ther their size and/or shape (Lee et al., 1984; Brown
et al., 2003; Bond, 2006). Such criteria are observa-
tions of explicit properties, as seen on images. We
aim to extract implicit properties of lymph nodes us-
ing image analysis techniques, and which can lead to
a higher precision of lymph node criteria.
Lymph nodes are distributed in a priori unpre-
dictable locations within the mesorectum. Because
they are small, subject to the PVE, and are in un-
predictable locations, we first determine information
about the surrounding mesorectum and use it to lo-
cate the lymph nodes. More precisely, we first model
the PDF of the mesorectum. However, to estimate the
mesorectum PDF we first need to isolate it from its
surrounding tissues.
The dark round object at the lower center in Figure
1 is the colorectum. The object of higher intensity that
envelops the colorectum is the mesorectum, bound by
the mesorectal fascia. The mesorectal fascia can be
recognised by human vision as a very thin low signal
intensity, however, because of the low signal to noise
and the poor sampling density, edge detectors tend to
produce disconnected edges.
To isolate the mesorectum, we need to delineate
the boundary of the mesorectal fascia and the bound-
ary of the colorectum. The difficulty of this segmenta-
tion task derives from the fact that there is, in several
places, no intensity or texture difference between the
VISAPP 2008 - International Conference on Computer Vision Theory and Applications
404