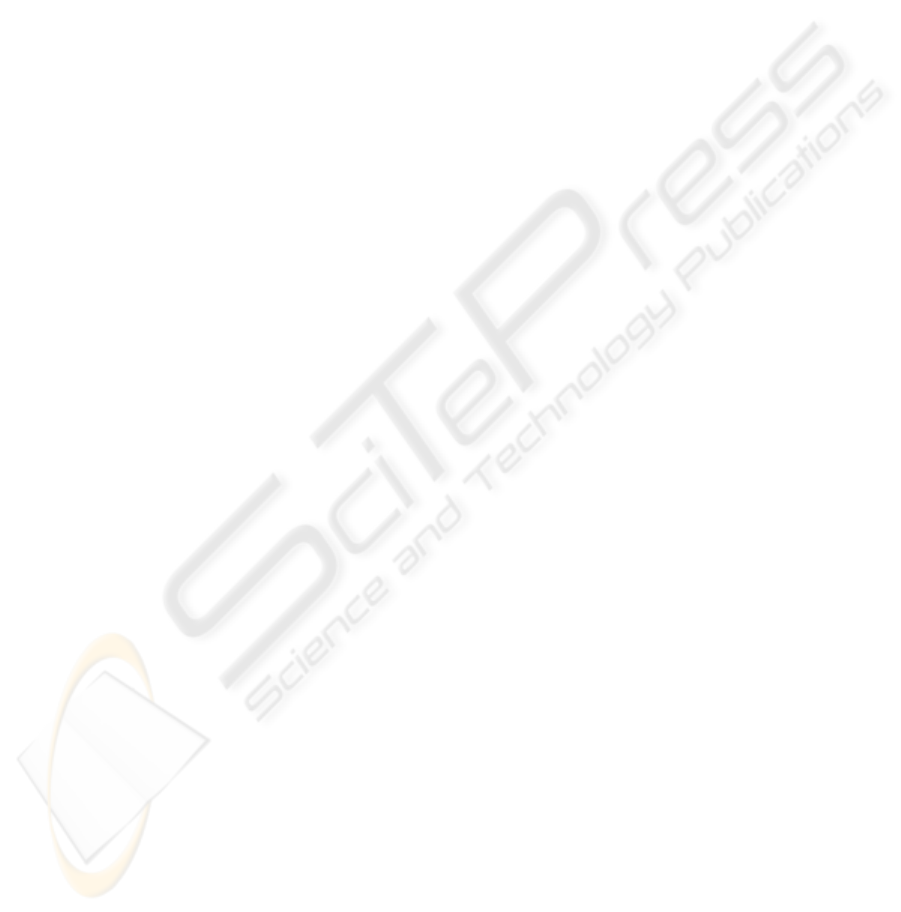
be quite different for the two subjects - 100 for sub-
ject 1 and 25 for subject 2. This indicates that while
different subjects might have a common understand-
ing of the task definition, they might have different
preferences when it comes to the relative weighting
of accuracy, energy and time. These varying inter-
nal preferences might explain the stylistic variations
observed among subjects performing the same task.
This matter requires further study. In our experiment
we use different ρ values for the different subjects
during mode estimation.
Since the data set is fairly small, we set all the
modes to be equally likely apriori. The average time
spent in any mode τ, as observed in the training data
set, was used to set the transition probabilities as H
ii
=
1−(1/τ), i = 1,. ..N and H
ij
= (1−H
ii
)/(N−1)∀i 6=
j. The value of τ was fixed at 20 (sampling instants)
for the results below, but the estimation performance
was found to be not very sensitive to the value of τ.
The average accuracy of the mode estimation was
86 percent. The errors are almost entirely confined
to the segmentation boundaries as can be seen in fig-
ures 5 and 6. At other times, the mode is usually
correctly estimated with a high degree of confidence.
Figures 7 and 8 compare the estimated joint angles
with the ground truth obtained from the tracking al-
gorithm (Lien et al., 2007).
5 CONCLUSIONS
In this paper, we have proposed a new approach to
the problem of representation and recognition of hu-
man motion. Our experimental results clearly indi-
cate the validity of our proposal. However, there are
several issues that need to be addressed to solve the
action recognition problem comprehensively, within
this framework. Our experiments indicate that while
different subjects might share a common goal for the
motion, they might tend to tradeoff the competing
concerns of accuracy, energy and time differently. We
are currently working on extending the estimation al-
gorithm to estimate the relative weights online, along
with the state and the mode.
REFERENCES
Blom, H. A. P. and Bar-Shalom, Y. (1988). The interact-
ing multiple model algorithm for systems with marko-
vian switching coefficients. Automatic Control, IEEE
Transactions on, 33(8):780–783.
Bregler, C. and Malik, J. (1997). Learning and recognizing
human dynamics in video sequences. In IEEE Con-
ference on Computer Vision and Pattern Recognition
(CVPR), pages pp 568–674.
de Freitas, N. (2002). Rao-blackwellised particle filtering
for fault diagnosis. Aerospace Conference Proceed-
ings, 2002. IEEE, 4.
Del Vecchio, D., Murray, R., and Perona, P. (2003). Decom-
position of human motion into dynamics based primi-
tives with ap- plication to drawing tasks. Automatica,
39(12):2085–2098.
Fod, A., Matari´c, M., and Jenkins, O. (2002). Automated
Derivation of Primitives for Movement Classification.
Autonomous Robots, 12(1):39–54.
Harris, C. and Wolpert, D. (1998). Signal-dependent noise
determines motor planning. Nature, 394(6695):780–
4.
Lewis, F. and Syrmos, V. (1995). Optimal Control. Wiley-
Interscience.
Li, W. and Todorov, E. (2004). Iterative linear-quadratic
regulator design for nonlinear biological movement
systems. First International Conference on Informat-
ics in Control, Automation and Robotics, 1:222–229.
Lien, J.-M., Kurillo, G., and Bajcsy, R. (2007). Skeleton-
based data compression for multi-camera tele-
immersion system. In Proceedings of the Interna-
tional Symposium on Visual Computing, Lake Tahoe,
Nevada/California,Nov 2007, to appear.
McGinnity, S. and Irwin, G. (2000). Multiple model
bootstrap filter for maneuvering target tracking.
Aerospace and Electronic Systems, IEEE Transac-
tions on, 36(3):1006–1012.
Murray, R., Sastry, S., and Li, Z. (1994). A Mathematical
Introduction to Robotic Manipulation. CRC Press.
Nori, F. and Frezza, R. (2005). Control of a manipula-
tor with a minimum number of motion primitives.
Proceedings of the 2005 IEEE International Confer-
ence on Robotics and Automation, 2005., pages 2344–
2349.
Oliver, N., Garg, A., and Horvitz, E. (2004). Layered rep-
resentations for learning and inferring office activity
from multiple sensory channels. Computer Vision and
Image Understanding, 96(2):163–180.
Park, S. and Aggarwal, J. (2004). A hierarchical Bayesian
network for event recognition of human actions and
interactions. Multimedia Systems, 10(2):164–179.
Pitt, M. K. and Shephard, N. (2001). Auxiliary variable
based particle filters. In book Sequential Monte Carlo
Methods in Practice, Arnaud Doucet - Nando de Fre-
itas - Neil Gordon (eds). Springer-Verlag, 2001.
Safonova, A., Hodgins, J., and Pollard, N. (2004). Syn-
thesizing physically realistic human motion in low-
dimensional, behavior-specific spaces. ACM Trans-
actions on Graphics (TOG), 23(3):514–521.
Scott, S. (2004). Optimal feedback control and the neu-
ral basis of volitional motor control. Nature Reviews
Neuroscience, 5(7):532–546.
Todorov, E. (2004). Optimality principles in sensorimotor
control. Nature Neuroscience, 2004:907–915.
REPRESENTATION AND RECOGNITION OF HUMAN ACTIONS - A New Approach based on an Optimal Control
Motor Model
103