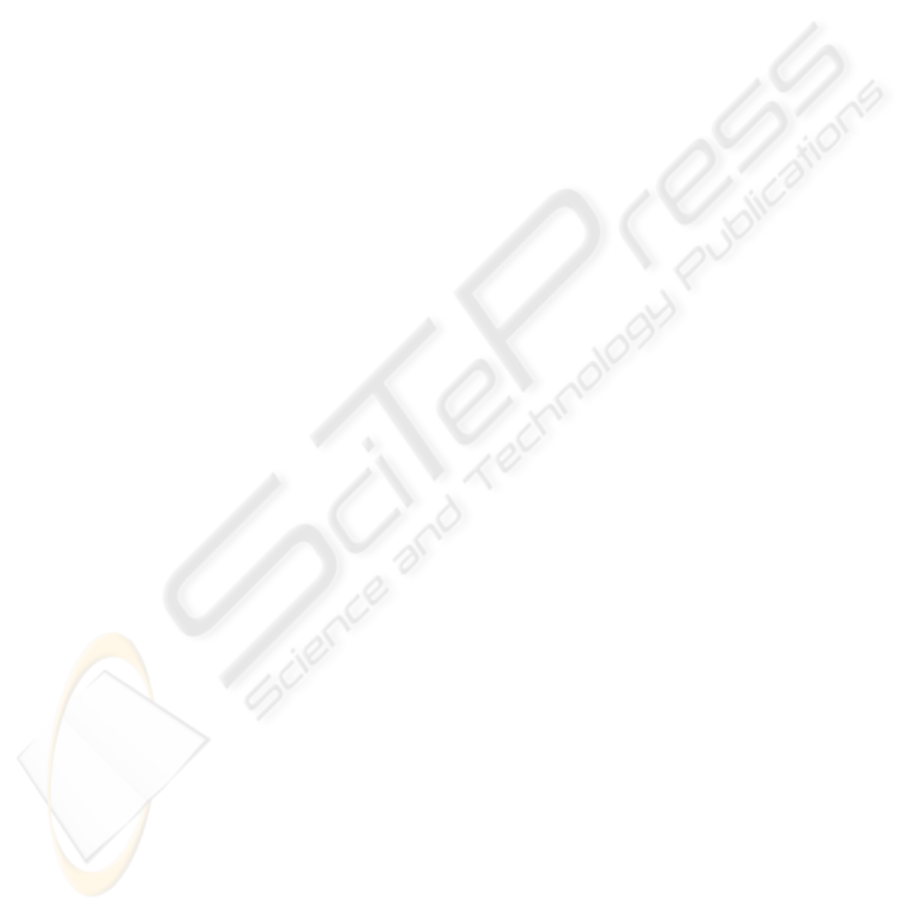
based fitting. These objective functions are learned
from annotated images. Generic and person-specific
objective functions are learned by training them with
all or only images with a specific person in them re-
spectively. In practice, it is infeasible to learn objec-
tive function for each person individually. We there-
fore extend the person-specific approach by first au-
tomatically partitioning the set of images into similar
partitions before learning, and then learning partition-
specific objective functions.
The main application of partition-specific objec-
tive functions, is tracking models through image se-
quences. Although the appearance of a face might
change during an image sequence due to lighting etc.,
the face itself does not. Therefore, once the partition
a face belongs to is established, a partition-specific
objective function can be used throughout the image
sequence.
The empirical evaluation first shows how person-
specific objective functions achieve a substantial
higher fitting accuracy for the person for which it was
trained. We then show the result of applying differ-
ent partition-specific objective functions on images in
and outside of the partition. As expected, partition-
specific objective function perform substantially bet-
ter than generic ones for persons from the partition for
which they were trained, but worse on persons not in
this partition. Higher accuracy comes at the cost of
lower generality. This trade-off is influenced by the
number of intended partitions G.
The off-line partitioning for learning partition-
specific objective functions is performed automati-
cally. We are currently investigating the use of an au-
tomatic classification to determine on-line, to which
partition a person belongs, and which objective func-
tion should be used.
ACKNOWLEDGEMENTS
This research is partially funded by a JSPS Post-
doctoral Fellowship for North American and Euro-
pean Researchers (FY2007) as well as by the German
Research Foundation (DFG) as part of the Transre-
gional Collaborative Research Center SFB/TR 8 Spa-
tial Cognition.
It has been jointly conducted by the Perceptual
Computing Lab of Prof. Tetsunori Kobayashi at
Waseda University, the Chair for Image Under-
standing at the Technische Universit
¨
at M
¨
unchen,
and the Group of Cognitive Neuroinformatics at the
University of Bremen.
REFERENCES
Chibelushi, C. C. and Bourel, F. (2003). Facial expression
recognition: A brief tutorial overview.
Cohen, I., Sebe, N., Chen, L., Garg, A., and Huang, T.
(2003). Facial expression recognition from video se-
quences: Temporal and static modeling. CVIU special
issue on face recognition, 91(1-2):160–187.
Cootes, T. F. and Taylor, C. J. (1992). Active shape models
– smart snakes. In BMVC, pp 266 – 275.
Cootes, T. F. and Taylor, C. J. (2004). Statistical models of
appearance for computer vision. Technical report, U
of Manchester, Imaging Science and Biomedical En-
gineering, Manchester M13 9PT, UK.
Cristinacce, D. and Cootes, T. F. (2006). Facial feature
detection and tracking with automatic template selec-
tion. In FGR, pp 429–434.
Ginneken, B., Frangi, A., Staal, J., Haar, B., and Viergever,
R. (2002). Active shape model segmentation with op-
timal features. IEEE Transactions on Medical Imag-
ing, 21(8):924–933.
Gross, R., Baker, S., Matthews, I., and Kanade, T. (2004).
Face recognition across pose and illumination. In
Li, S. Z. and Jain, A. K., editors, Handbook of Face
Recognition. Springer-Verlag.
Gross, R., Matthews, I., and Baker, S. (2005). Generic vs.
person specific active appearance models. Image and
Vision Computing, 23(11):1080–1093.
Hanek, R. (2004). Fitting Parametric Curve Models to Im-
ages Using Local Self-adapting Seperation Criteria.
PhD thesis, Dept of Informatics, TU M
¨
unchen.
Jones, M. J. and Viola, P. (2003). Fast multi-view face
detection. Technical Report TR2003-96, Mitsubishi
Electric Research Lab.
Nefian, A. and Hayes, M. (1999). Face recognition using an
embedded HMM. In Proc. of the IEEE Conference on
Audio and Video-based Biometric Person Authentica-
tion, pp 19–24.
Pantic, M. and Rothkrantz, L. J. M. (2000). Automatic anal-
ysis of facial expressions: The state of the art. IEEE
TPAMI, 22(12):1424–1445.
Quinlan, R. (1993). C4.5: Programs for Machine Learning.
Morgan Kaufmann, San Mateo, California.
Romdhani, S. (2005). Face Image Analysis using a Multi-
ple Feature Fitting Strategy. PhD thesis, U of Basel,
Computer Science Department, Basel, CH.
Tian, Y.-L., Kanade, T., and Cohn, J. F. (2001). Recogniz-
ing action units for facial expression analysis. IEEE
TPAMI, 23(2):97–115.
Viola, P. and Jones, M. (2001). Rapid object detection using
a boosted cascade of simple features. In CVPR, vol 1,
pp 511–518, Kauai, Hawaii.
Wimmer, M., Stulp, F., Pietzsch, S., and Radig, B. (2007).
Learning local objective functions for robust face
model fitting. In IEEE PAMI. to appear.
Witten, I. H. and Frank, E. (2005). Data Mining: Practi-
cal machine learning tools and techniques. Morgan
Kaufmann, San Francisco, 2
nd
edition.
VISAPP 2008 - International Conference on Computer Vision Theory and Applications
12