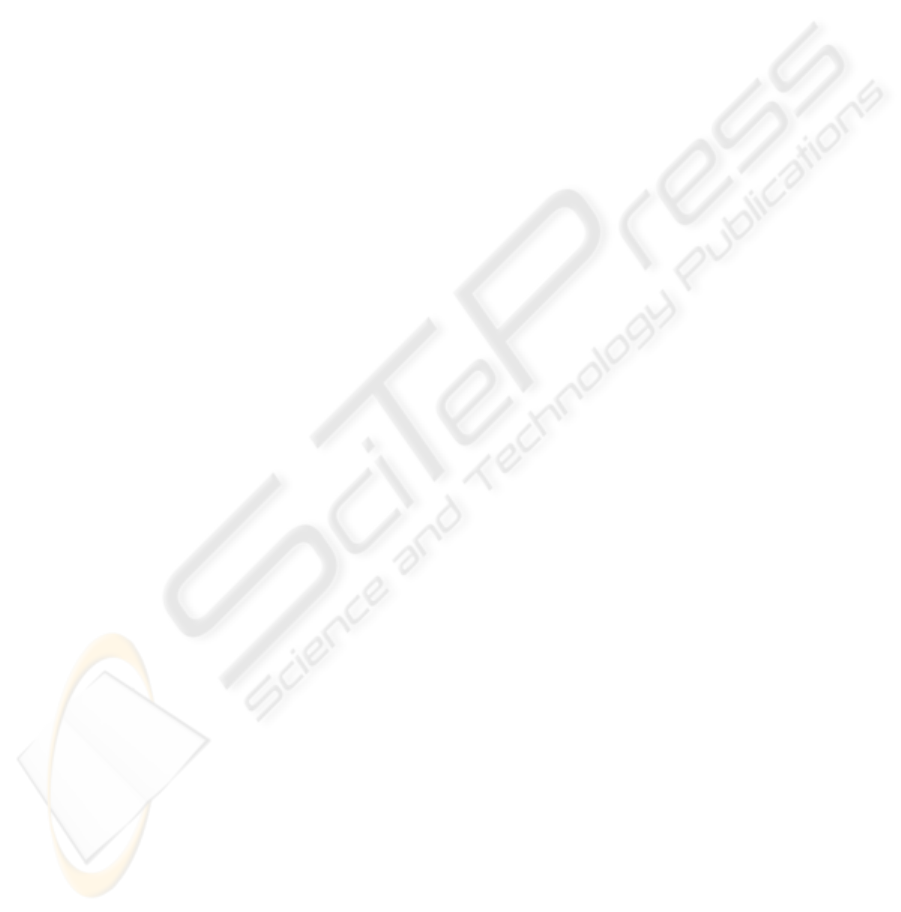
step by looking for the point after which the perfor-
mance of the system is not significantly improved by
increasing the value of σ. The associated value of σ
is then used in the test stage.
Tables 2 contains a summary of the results ob-
tained using the individual scoring function on the
evaluation and test sets when manually annotated eyes
position were used for the face geometric normalisa-
tion. The values in the table indicate the Total Error
rates in the Evaluation (TEE) and Test (TET) stages
respectively. For the sake of simplicity of compar-
ison, the evaluation and test results have been also
shown in figures 2(a) and (b) respectively.
These results clearly demonstrate that among the
adopted metric, individually,the GD metric is the out-
right winner. In a few cases, the NC results is com-
parable to the GD one. The performance of the other
metrics is much worse.
Plots (b) and (c) of the figure 2 demonstrate a sum-
mary of the results when the face registration step was
performed based on automatically localised eyes po-
sition. These results also confirm that the GD met-
ric is again the best scoring function for this applica-
tion. A comparison of the GD results and the results
of the similar experiments reported in (Sadeghi and
Kittler, 2006)using the basic form of the GD metric
shows that by optimising the GD metric as discussed,
the problem of sensitivity of this metric to the miss-
registration error is reduced so that the optimised GD
metric outperforms the NC function for both manu-
ally the automatically registered data.
In the next step, the problem of combining classi-
fiers derived from different similarity measures was
considered. We adopted the decision level fusion
strategy using the SVMs in order to combine the de-
signed classifiers. Table 3 contains the combined veri-
fication results using manually and automatically reg-
istered data. These results demonstrate that a better
performance is achieved using the combined method
especially on Mc and G protocols.
6 CONCLUSIONS
The problem of measuring similarity in LDA face
space and fusing the resulted classifiers were consid-
ered. It was shown that the optimised Gradient Direc-
tion metric outperforms the other metrics in different
conditions considering both manually and automati-
cally registered data. It was also demonstrated that the
performance of the verification system can be further
improved by fusing the adopted similarity measures
in the decision level using the SVM classifier.
ACKNOWLEDGEMENTS
The financial support from the Iran Telecommuni-
cation Research Centre is gratefully acknowledged.
A partial support from the Engineering and Physical
Sciences Research Council (EPSRC), Research Grant
GR/S46543 is also gratefully acknowledged.
REFERENCES
Bao, Q. and Guo, P. (2004). Comparative studies on simi-
larity measures for remote sensing image retrieval. In
IEEE International Conference on Systems, Man and
Cybernetics.
Belhumeur, P., Hespanha, J., and Kriegman, D. (1997).
Eigenfaces vs. Fisherfaces: Recognition using class
specific linear projection. IEEE Trans. on Pattern
Recognition and Machine Intelligence, 19(7):711–
720.
Hamouz, M., Kittler, J., Kamarainen, J.-K., Paalanen, P.,
Kalviainen, H., and Matas, J. (2005). Feature-based
affine-invariant localization of faces. IEEE Transac-
tions on Pattern Analysis and Machine Intelligence,
27(9):1490–1495.
Kittler, J., Li, Y. P., and Matas, J. (2000). On matching
scores for LDA-based face verification. In Mirmehdi,
M. and Thomas, B., editors, Proceedings of British
Machine Vision Conference 2000, pages 42–51.
Perlibakas, V. (2004). Distance measures for pca based face
recognition. Pattern Recognition letters, 25(6).
Sadeghi, M. and Kittler, J. (2004). Decision making in the
LDA space: Generalised gradient direction metric. In
the 6th International Conference on Automatic Face
and Gesture Recognition, pages 248–253, Seoul, Ko-
rea.
Sadeghi, M. and Kittler, J. (2006). Confidence based gat-
ing of multiple face authentication experts. In Joint
IAPR International Workshops on Syntactical and
Structural Pattern Recognition and Statistical Pattern
Recognition, S+SSPR 2006, pages 667–676, Hong
Kong, China.
Seredin, O., Kostin, A., Kittler, J., and Mottl, V. (2001).
Leave-one-out procedure for finding discriminant
function threshold in machine learning by support
vector method. In Proceedings of 10th conference
on Mathematical Methods of Pattern Recognition,
MMRO-10, pages 128–131.
Vapnik, V. (1995). The Nature of Statistical Learning The-
ory. Springer-Verlag, New York.
Yambor, W., Draper, B., and Beveridge, J. (2002). Analyz-
ing pca-based face recognition algorithm: Eigenvector
selection and distance measures. In Christensen, H.,
Phillips, J. (Eds.), Empirical Evaluation Methods in
Computer Vision. World Scientific Press, Singapore.
Zhang, D. and Lu, G. (2003). Evaluation on similarity mea-
surement for image retrieval. Neural Network and Sig-
nal Processing, 2.
SIMILARITY MEASURES FUSION USING SVM CLASSIFIER FOR FACE AUTHENTICATION
109