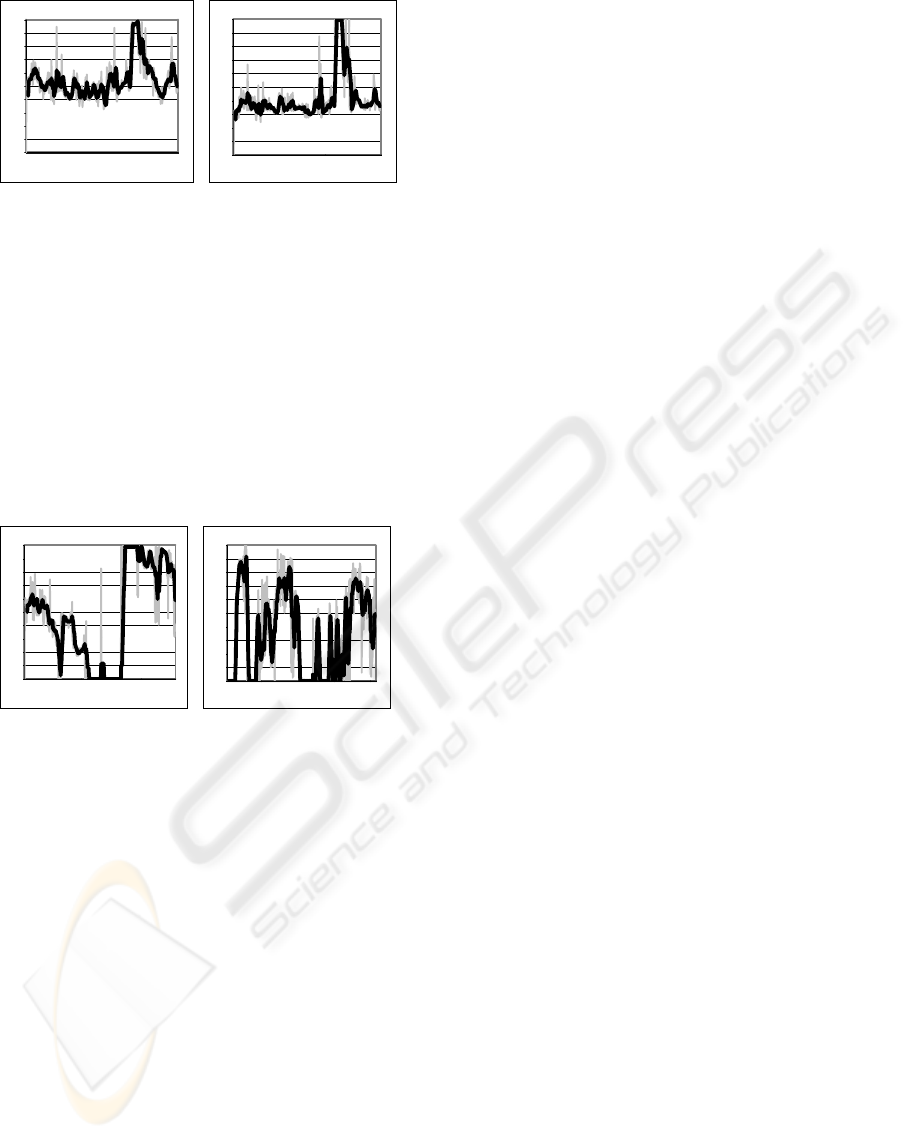
0
0,1
0,2
0,3
0,4
0,5
0,6
0,7
0,8
0,9
1
2201
2220
2239
2258
2277
2296
2315
2334
2353
2372
2391
2410
2429
2448
2467
2486
0
0,1
0,2
0,3
0,4
0,5
0,6
0,7
0,8
0,9
1
2201
2219
2237
2255
2273
2291
2309
2327
2345
2363
2381
2399
2417
2435
2453
2471
2489
Figure 3: Left:
),( MU
of the most suspicious blob based
on the GMM estimation for the video “Syn”. The
difference between usual and unusual events decreased
compared to the previous method. Right: Detection by
segmenting the probability field.
the result of the video where the bicyclist is detected
(“Crossing” sequence) while the right graph shows
the most suspicious blob’s probability in the
“Lanes” video. It is obvious where the bicycle
appears in the last third of the graph while in the
other example the first peak belongs to the people
crossing the street while other smaller peaks belong
to cars touching the centre lines.
0
0,1
0,2
0,3
0,4
0,5
0,6
0,7
0,8
0,9
1
27504
27591
27678
27765
27852
27939
28026
28113
28200
28287
28374
28461
28548
28635
28722
28809
28896
28983
0
0,1
0,2
0,3
0,4
0,5
0,6
0,7
0,8
0,9
1
6501
6607
6716
6821
6932
7044
7155
7267
7381
7493
7605
7711
7822
7930
8039
8151
8267
8377
Figure 4: Left:
),( MU
of the most suspicious blob
obtained by segmenting the probability field of the video
“Crossing”. Right: the same for the video “Lanes”.
6 CONCLUSIONS
We considered three pixel-based approaches for the
local representation of motion directions. The
Markovian hypothesis proved to be very useful
giving more discriminating power between unusual
and usual events. The method of Estimated
empirical probability requires the quantization of
motion directions which can reduce the sensitivity in
case of very complex motion fields and makes the
method less sensible for little deviations. Mixture of
Gaussians can reduce the memory requirements and
can maintain arbitrary directions. The traditional
update of model parameters (
Stauffer, 1999) can not
follow the changes in traffic; instead an Expectation
Maximization algorithm should be tested in future.
The Mean-shift segmented probability field
introduces spatial support with some improvements.
All methods run in real-time (@3-15Hz) on a 3GHz
PC considering a 320x240 colour image with
varying frame rate
ACKNOWLEDGEMENTS
The authors would like to thank the help of Attila
Licsár and the support of the GVOP-3.1.1.-2004-05-
0388/3.0 national project.
REFERENCES
E. L. Andrade, S. J. Blunsden, and R. B. Fisher.
Characterisation of optical flow anomalies in
pedestrian traffic. The IEE International Symposium
on Imaging for Crime Prevention and Detection, pp.
73-78, 2005.
J.R. Bergen & R. Hingorani. Hierarchical Motion-Based
Frame Rate Conversion. Technical report, David
Sarnoff Research Center Princeton NJ 08540, 1990.
O. Boiman, M. Irani, Detecting Irregularities in Images
and in Video. International Conference on Computer
Vision (ICCV), Bejing, pp. 462-469, 2005.
M. Brand and V. Kettnaker. Discovery and segmentation
of activities in video. IEEE Trans. Pattern Analysis
and Machine Intelligence, 22(8), pp. 844–851, August
2000.
Anthony R. Dick and Michael J. Brooks. Issues in
Automated Visual Surveillance, International
Conference on Digital Image Computing: Techniques
and Applications (DICTA 2003), Sydney, pp.195-204.
2003.
Bogdan Georgescu, Ilan Shimshoni, and Petert Meer.
Mean shift based clustering in high dimensions: A
texture classification example, 9th International
Conference on Computer Vision, Nice, pp. 456-463,
2003.
Weiming Hu, Tieniu Tan, Liang Wang, and Steve
Maybank. A Survey on Visual Surveillance of Object
Motion and Behaviours, IEEE Transactions on
Systems, Man and Cybernetics, Part C: Applications
and Reviews, Vol 34, Issue 3, pp. 334-352, 2004.
V. Nair and J.J. Clark. Automated visual surveillance
using hidden Markov models. In VI02, pp 88, 2002.
I. Pavlidis, V. Morellas, P. Tsiamyrtzia, and S. Harp.
Urban surveillance systems: from the laboratory to the
commercial world. Proceedings of the IEEE, 89(10),
pp. 1478–1497, 2001.
C. Stauffer and W.E.L. Grimson. Adaptive Background
Mixture Models for Real-time Tracking. CVPR, pp.
246-252, 1999.
ANOMALY DETECTION WITH LOW-LEVEL PROCESSES IN VIDEOS
681