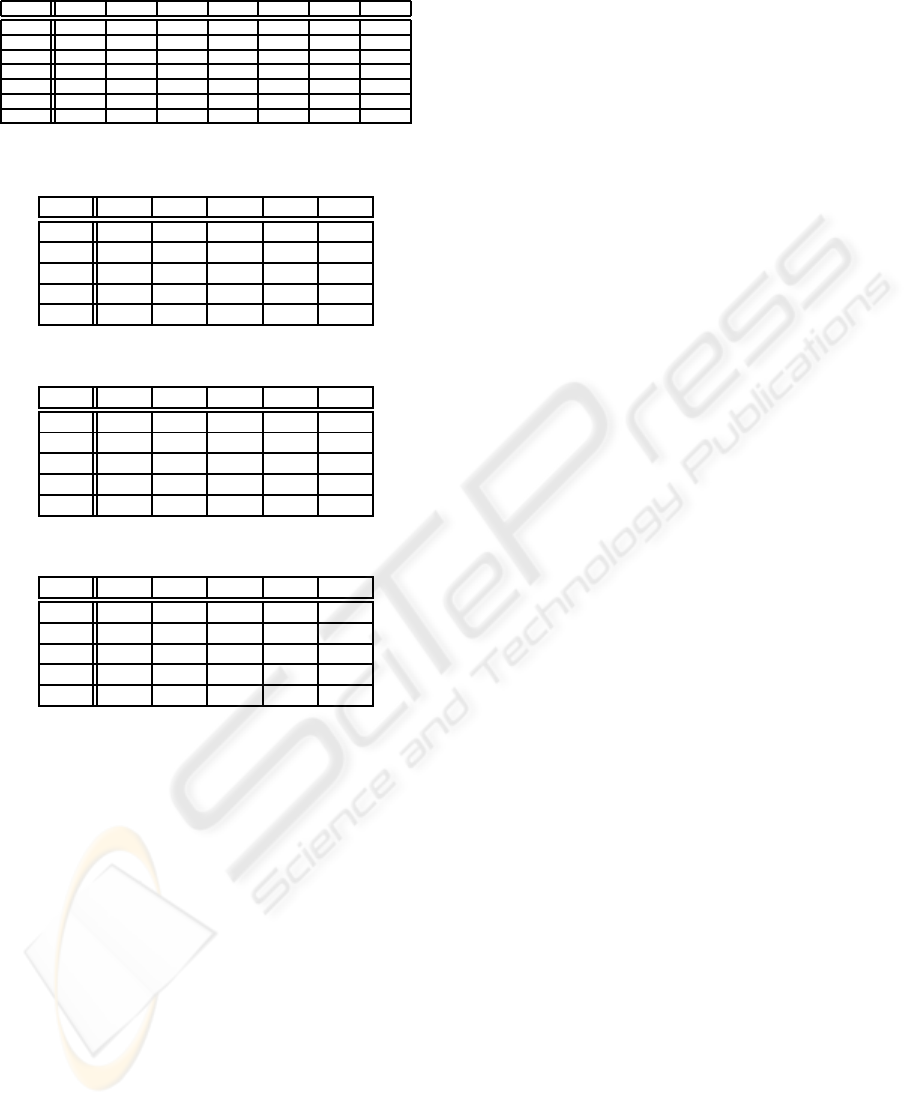
Table 6: Confusion Matrix 99.0% Overal recognition rate = 61.2%.
Neut Happ Sad Surp Ang Fear Disg
Neut 52.38 0 42.86 0 4.76 0 0
Happ 0 90.48 4.76 0 0 4.76 0
Sad 4.76 4.76 76.19 0 4.76 4.76 4.76
Surp 0 0 0 76.19 0 23.81 0
Ang 4.76 0 9.52 0 33.33 23.81 28.57
Fear 0 9.52 4.76 14.29 4.76 66.67 0
Disg 0 23.81 4.76 0 33.33 4.76 33.33
Table 7: Confusion Matrix 97.0% Overal recognition rate = 74.3%.
Neut Happ Surp Fear Disg
Neut 57.14 0 0 42.86 0
Happ 0 90.48 0 9.52 0
Surp 0 0 76.19 23.81 0
Fear 9.52 4.76 23.81 61.90 0
Disg 0 9.52 0 4.76 85.71
Table 8: Confusion Matrix 98.0% Overal recognition rate = 76.2%.
Neut Happ Surp Fear Disg
Neut 90.48 0 0 9.52 0
Happ 0 85.71 0 4.76 9.52
Surp 0 0 71.43 28.57 0
Fear 4.76 4.76 19.05 66.67 4.76
Disg 0 19.05 0 14.29 66.67
Table 9: Confusion Matrix 99.0% Overal recognition rate = 63.8%.
Neut Happ Surp Fear Disg
Neut 80.95 9.52 4.76 4.76 0
Happ 9.52 66.67 0 14.29 9.52
Surp 4.76 0 66.67 28.57 0
Fear 4.76 9.52 28.57 52.38 4.76
Disg 9.52 23.81 4.76 9.52 52.38
5 CONCLUSIONS
We used standart AAM model to discribe face entities
in a compact way. With LDA we are able to separate
the several emotion expression classes and perform
classification using mahalanobis distance.
On the AAM model building process, holding
more information on the appearance vectors not al-
ways results on a better discrimination result. In our
experiments, this value rounds 99.0% of variance re-
tained.
The number of LDA eigenvectors used is another
parameter of great importance. K-means is used in
order to give us a good estimation for this value.
As expected, the larger the number of expressions
used, the worse is the overal successful classification
rate. The reason for this is that there are correlations
between the two pairs of expressions neutral, sad and
anger, disgust, comproved by psico-physics studies.
We achieved, with all the seven expressions, an
overal successful recognition rate of 61.1%. Remov-
ing correlated expressions, this recognition rate in-
creases to a maximum of 76.2%.
REFERENCES
Batista, J. P. (2007). Locating facial features using an an-
thropometric face model for determining the gaze of.
faces in image sequences. ICIAR2007 - Image Analy-
sis and Recognition.
Bouchra Abboud, Frank Davoine, M. D. (2004). Facial
expression recognition and synthesis based on an ap-
pearance model. Signal Processing Image Communi-
cation.
G. Finlayson, S. Hordley, G. S. and Tian, G. Y. (2005). Il-
luminant and device invariant color using histogram
equalisation. Pattern Recognition.
Killgore, W. D. and Yurgelun-Todd, D. A. (2003). Acti-
vation of the amygdala and anterior cingulate during
nonconscious processing of sad versus happy faces.
NeuraImage.
Krzanowski, W. J. (1988). Principles of multivariate analy-
sis. Oxford University Press.
Peter N. Belhumeur, J. P. H. and Kriegman, D. J. (1997).
Eigenfaces vs. fisherfaces: Recognition using class
specific linear projection. IEEE Transactions on Pat-
tern Analysis and Machine Intelligence.
P.Viola and Jones, M. (2004). Rapid object detection using
a boosted cascate of simple features. Proceeding of
the IEEE Conference on Computer Vision and Pattern
Recognition.
Stegmann, M. B. (2000). Active appearance models theory,
extensions & cases. Master’s thesis, IMM Technical
Univesity of Denmark.
Stegmann, M. B. and Gomez, D. D. (2002). A brief intro-
duction to statistical shape analysis. Technical report,
Informatics and Mathematical Modelling, Technical
Univesity of Denmark.
T. Dalgleish, M. P. (1999). Handbook of cognition and emo-
tion. John Wiley & Sons Ltd.
T.F.Cootes and C.J.Taylor (2004). Statistical models of ap-
pearance for computer vision. Technical report, Imag-
ing Science and Biomedical Engineering - University
of Manchester.
T.F.Cootes, G. E. and C.J.Taylor (2001). Active appearance
models. IEEE Transactions on Pattern Analysis and
Machine Intelligence.
FACIAL EXPRESSION RECOGNITION USING ACTIVE APPEARANCE MODELS
129