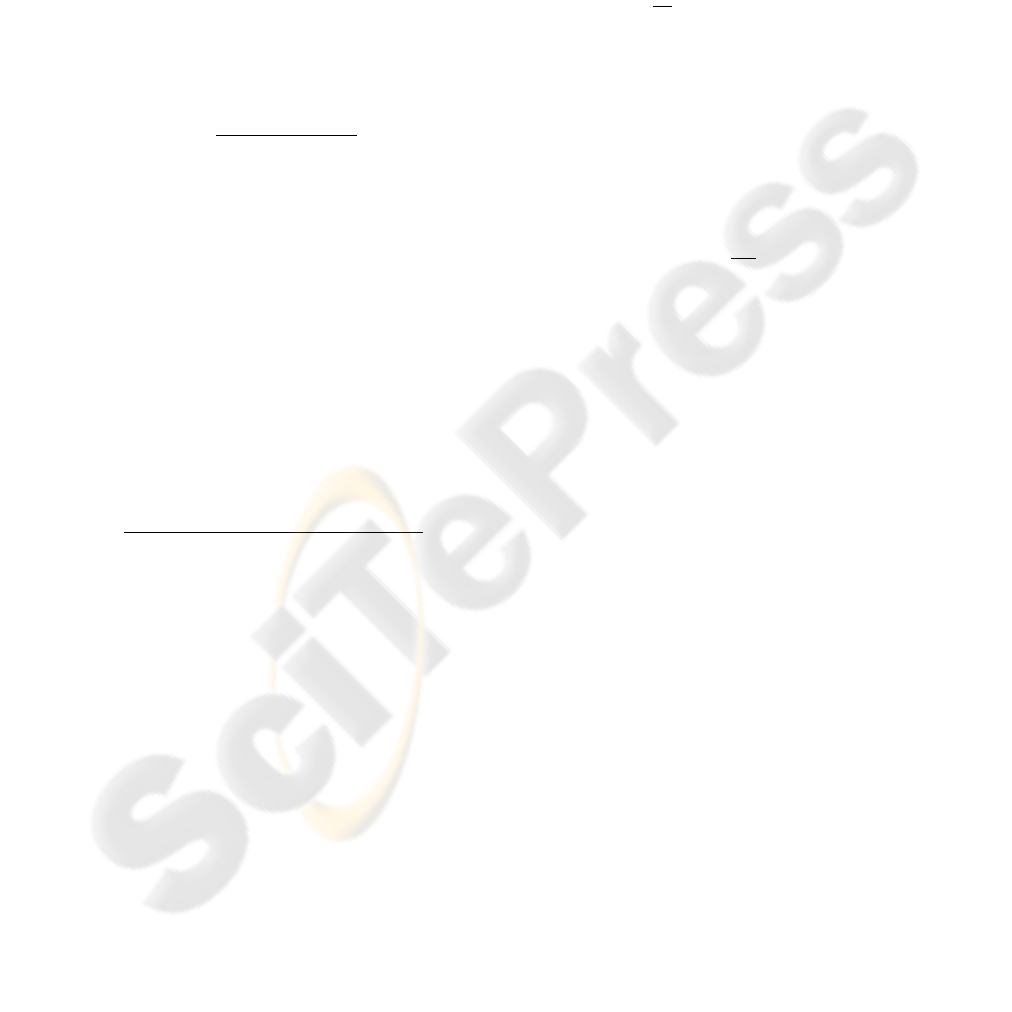
dressed through a split and merge criterion. However,
these methods are either too slow for online learning
(Hall and Hicks, 2005), assume that data arrives in
chunks (Song and Wang, 2005) or does not guarantee
the fidelity of the resulting model (Arandjelovic and
Cipolla, 2005). Here we propose a new efficient on-
line method that explicitly guarantees the accuracy of
the model through a fidelity criterion.
2.1 Update of the Gaussian Mixture
Model
Suppose we have already learned a precise GMM
from the observations up to time t:
p
t
(x) =
∑
N
i=1
π
t
i
g(x;µ
t
i
,C
t
i
)
∑
N
i=1
π
t
i
(1)
where each Gaussian is represented by its weight
π
t
i
, its mean µ
t
i
and its covariance C
t
i
. We then re-
ceive a new data point represented by its distribution
g
t
(x;µ
t
,C
t
) and its weight π
t
. C
t
here represents the
observation noise. As suggested by Hall and Hicks
(Hall and Hicks, 2005), the new resulting GMM is
computed in two steps:
1. Concatenate – produce a model with N + 1 com-
ponents by trivially combining the GMM and the
new data into a single model.
2. Simplify – if possible, merge some of the Gaus-
sians to reduce the complexity of the GMM.
The GMM resulting from the first step is simply
p
t
(x) =
∑
N
i=1
π
t−1
i
g(x;µ
t−1
i
,C
t−1
i
) + π
t
g
t
(x;µ
t
,C
t
)
∑
N
i=1
π
t−1
i
+ π
t
(2)
The goal of the second step is to reduce the complex-
ity of the model while still giving a precise descrip-
tion of the observations. Hall and Hicks (Hall and
Hicks, 2005) propose to group the Gaussians using
the Chernoff bound to detect overlapping Gaussians.
Different thresholds on this bound are then tested and
the most likely result is kept as the simplified GMM.
Since this method is too slow for an on-line process,
we use a different criterion proposed by Declercq and
Piater (Declercq and Piater, 2007) for their uncertain
Gaussian model. This model provides a quantitative
estimate λ of its ability to describe the associated data
that takes on a value close to 1 if the data distribu-
tion is Gaussian and near zero if it is not. This value,
called the fidelity in the sequel, is useful to decide if
we can merge two given Gaussians without drifting
from the real data distribution.
2.2 Estimating the Fidelity of a
Gaussian Model
To estimate the fidelity λ of a Gaussian model, we first
need to compute the distance between this model and
its corresponding data set. This is done with a method
inspired from the Kolmogorov-Smirnoff test,
D =
1
|
I
|
Z
I
ˆ
F(x) − F
n
(x)
dx, (3)
where F
n
(x) is the empirical cumulative distribution
function of the n observations,
ˆ
F(x) is the correspond-
ing cumulative Gaussian distribution, and I is the in-
terval within which the two functions are compared.
To simplify matters, the distance D is assumed to have
a Gaussian distribution, which leads to the pseudo-
probabilistic weighting function
λ = e
−D
2
T
2
D
, (4)
where T
D
is a user-settable parameter that represents
the allowed deviation of observed data from Gaus-
sianity. Whereas the sensitivity of the Kolmogorov-
Smirnov test grows without bounds with n, λ provides
a bounded quantification of the correspondence be-
tween the model and the data. Therefore, this crite-
rion is more appropriate for our case since we need
to estimate the correspondence of the data with the
model and not their possible convergence to a Gaus-
sian distribution.
Thus, the original data are not required anymore
if we keep in memory an approximation of their cu-
mulative distribution within a given interval. Since
the number of dimensions of the data space can be
large, we compute the distance D for each dimension
separately to keep the computational cost linear in the
number of dimensions. The total distance is then sim-
ply the sum of these individual distances.
2.3 Simplification of the Gaussian
Mixture Model
To decide whether two Gaussians G
i
and G
j
can
be simplified into one, we merge them together and
check if the resulting Gaussian has a fidelity λ close
to one, say, exceeding a given threshold λ
+
min
= 0.95.
The resulting Gaussian is computed using the usual
equations supplemented by the combination of the cu-
VISAPP 2008 - International Conference on Computer Vision Theory and Applications
606