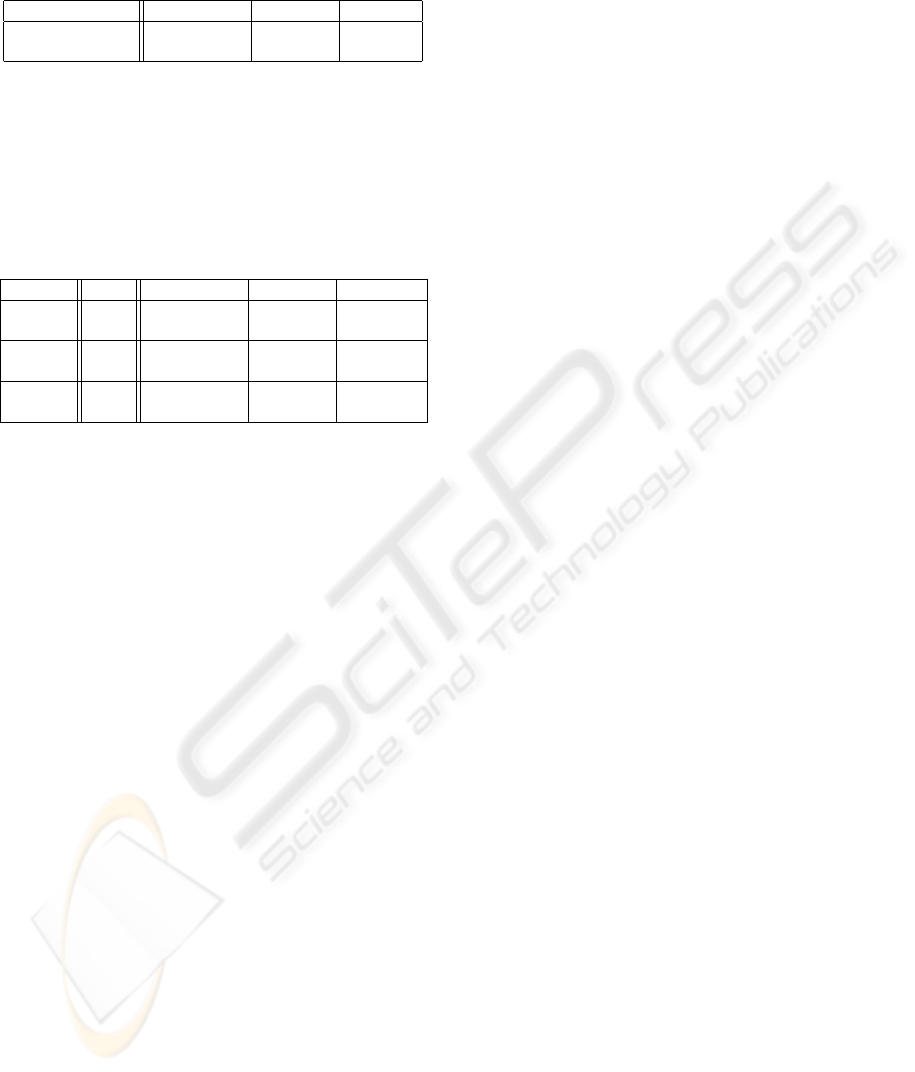
Table 1: Simulation Measure Process and Sensor Simula-
tion Process.
processing type Time Images/s Pixels/s
colour simulation 0.010311149 96.9824 547 ∗ 10
6
sensor simulation 0.00648231 154.266 870 ∗ 10
6
per-pixel process which requires the execution of a
pipeline of several complex mathematical and vector
calculations (FM04). As the GPU is more suited to
these kinds of operations, the results obtained are an
improvement in comparison to using only the CPU.
Table 2: Segmentation process.
colours ΔE Time Images/s Pixels/s
1 1976 0.000652175 1533.33 8650 ∗ 10
6
1 2000 0.003529765 283.305 1598 ∗ 10
6
5 1976 0.005996138 166.774 940 ∗ 10
6
5 2000 0.043635729 22.917 129 ∗ 10
6
10 1976 0.01042655 95.909 541 ∗ 10
6
10 2000 0.086909666 11.5062 65 ∗ 10
6
Table 2 indicates that the GPU performs very well
in this particular case study; especially if we only seg-
ment one colour. Nevertheless, it looks like this algo-
rithm grows linearly with the number of colour refer-
ences used.
5 CONCLUSIONS
The main aim of this work has been to investigate and
develop a new tool to help in the study and the anal-
ysis of multispectral images of paintings. The work
was focused on developing something innovative for
the Cultural Heritage domain using new technologies
such as GPU-based computation systems. The poten-
tial offered by colour spectrum decomposition tech-
niques opens up new ways to study works of art.
The Mona Lisa not only gave inspiration to
Leonardo, but also to our group to develop an applica-
tion capable of discovering details otherwise invisible
to the naked eye. This approach based on the fusion
between art, science and computer vision represents
a valuable aid in the field of art conservation, restora-
tion and art history and will require more in-depth in-
vestigation and a more multi-disciplinary community.
REFERENCES
Berns R. S.: The science of digitizing paintings for colour
accurate image archives: a review. In Journal of Imag-
ing Science and Technology 45 (2001), pp. 305–325.
Colantoni P., Boukala N., Rugna J. D.: Fast and accu-
rate colour image processing using 3d graphics cards.
In Vision Modeling anv Visualization, VMV (2003),
pp. 383–390.
CIE, Commission Internationale de l’Eclairage: Interna-
tional Congress on Illumination. Proceedings, In-
ternational Congress on Illumination (1931), Cam-
bridge.
CIE, Commission Internationale de l’Eclairage: Industrial
colour-difference evaluation. Proceedings, Interna-
tional Congress on Illumination (1995), Tech. Rep.
Colantoni P., Pillay R.,Lahanier C. and Pitzalis D.: Analy-
sis of multispectral images of paintings. In EUSIPCO
(2006), Florence, Italy.
Fung J., Mann S.: Computer Vision Signal Processing
on Graphics Processing Units. In Proceedings of the
IEEE International Conference on Acoustics, Speech,
and Signal Processing (2004), Montreal, Quebec,
Canada, May 17–21, 2004.
Luo M., Cui G., Rigg B.: The development of the CIE 2000
colour-difference formula: CIEDE2000. In Ph.D.
Thesis, University of Derby (1998), Derby.
Rib
´
es A., Schmitt F., Pillay R., Lahanier C.: Calibration
and spectral reconstruction for crisatel: an art painting
multispectral acquisition system. In Journal of Imag-
ing Science and Technology 49 (2005), 563–573.
Staniforth S.: Retouching and colour marching: the restorer
and metamerism. In Journal of Studies in Conserva-
tion 30 (1985), 101–111.
Vrhel M. J., Trussell H. J.: colour device calibration: a
mathematical formulation. In IEEE Transactions on
Image Processing 8, 12 (1999), 1796–1806.
GRAPP 2008 - International Conference on Computer Graphics Theory and Applications
496