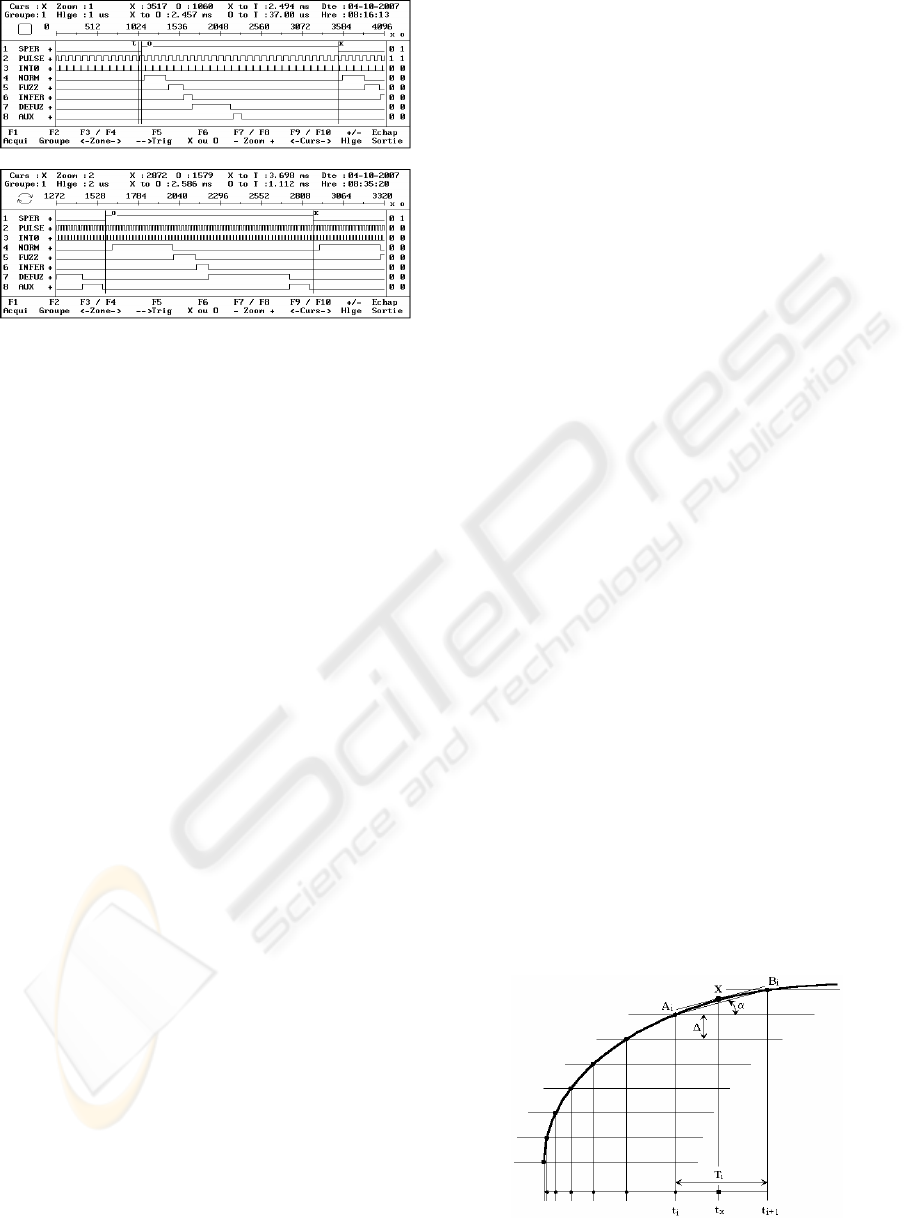
a.
b.
Figure 4: An on-line T in fuzzy control.
3 A FUZZY CONTROLLER
WITH ADAPTIVE T VALUES
The idea is to relate T with the variation rate of the
main variable of the system. During the intervals
with small variations (or in steady state regimes), T
is greater and during the high rate dynamic regimes,
T decreases. The variation for the (generic) variable
v from the step t induces an adequate adaptation of T
at t+δt. The figure 5 gives an image of the principle
and helps for obtaining some relations. A first
possibility is to evaluate the amplitude variation for
the main variable during a constant time interval
(easily in real-time). Another idea is to use an
amplitude quantization of the v variable using a
constant step Δ and to evaluate then the time
intervals associated with this variation. They can be
directly assimilated with the adapted T. Next
relations make connects the derivative value and Δ.
v = f(t); Δ = f(t
i+1
) - f(t
i
) ; tg α
i
= Δ / T
i
(5)
T
i
= Δ / tg α
i
≅ Δ / ( ⏐|df / dt|
ti
⏐+ ε) (6)
Δ could be chosen by practical considerations. If
f is known, (5) is useful for evaluate T. If not, t
i
result by detecting the amplitude thresholds and by
that the next T value is available. ε is for a
limitation of max.T. A limitation is also necessary
for minT, (systemic, on-line processing constraints):
T
min
≤ Τ ≤ Δ / ε (7)
For a servodrive where the main variable is the
position, let be the speed ω the variable v (the
variation of the position). It is more suitable to use a
T adaptation in accordance with the variation rate
not after the amplitude of the v variable. Indeed, for
that last case, even in a steady-state regime, the
sampling rate is high and for a low speed during a
strong dynamic regime the sampling has a slow
rate. If the main characteristic variable of the
system is the speed, v could be the acceleration.
The next idea is to adapt also the step value Δ
upon another characteristic variable of the
controlled system. The results for two Δ (constant)
values are depicted by figure 6, with the distribution
of the sampling moment. Position 1 is the sampled
position with an adaptive period. “State space FLC
path” is the trajectory of the system. The global
behaviour is good for both variants, the final
position error being null and the system response in
speed and position being a smooth one. Figures 7a
and 7b give the variations of T for Δ=2.456 and
Δ=20, during the whole regime. The max/min rate
values are almost 100/Δ=10 and 35/Δ=50. Figures
7c, 7d make visible the large variation range of the
max/min T along 3 magnitude ranges (logarithmic
scale).
The next idea is to use a variable quantization step
for adapting T as a double adaptive sampling
strategy. Another adaptation parameter is involved.
The fig. 8 gives the elements for that, considering
the speed as an additional modulator (by its change
rate) for the adaptive sampler of the position. In this
way, it is no more necessary to make different
experiments in order to adopt the best step value
Δ (Q). The distribution of the sampling moments is
different (fig. 8a) and the step value is variable (fig.
8b). The image of the new sampled position is given
by fig. 8c. The results from 8d concern another
values range for the modulator of the adaptive
sampler (a larger one – see Q_ad). It is depicted
also the quantified speed – Speed 1, as the source
of the modulator for the quantization step necessary
for the sampler with double adaptive T. The
overshoot for the position is related with its
quantized final values.
Figure 5: For adaptation of T values.
ON THE SAMPLING PERIOD IN FUZZY CONTROL ALGORITHMS FOR SERVODRIVES - A Strategy for Variable
Sampling
223