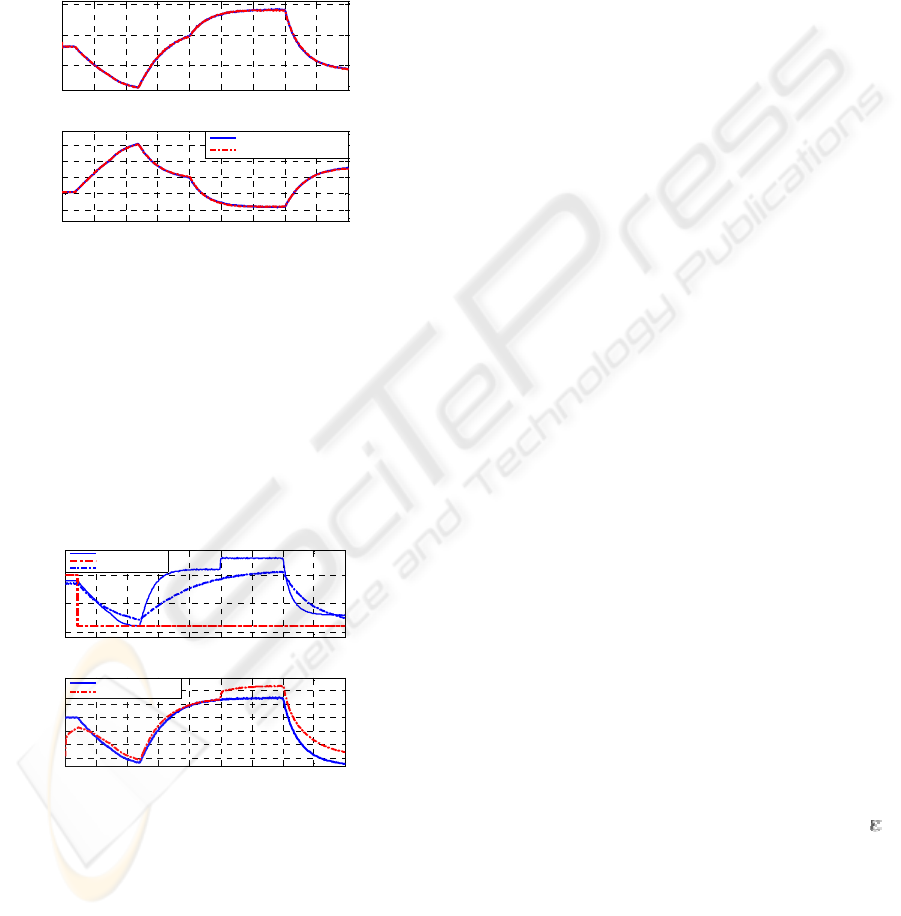
To achieve a desired speed and regard to the
nonlinear nature of the responses that we have still
in the SNs, we utilize a linear transfer function with
the order more than two. Whereas the higher order
models will cause some difficulties in the
application, we don’t use the order more than three.
Separate estimations using SISO models have less
accuracy than those using MISO models.
0 0.5 1 1.5 2 2.5 3 3.5 4 4.5
x 10
4
5
10
15
c. Multivariable High order model es timation
Temperature (C)
time (sec)
0 0.5 1 1.5 2 2.5 3 3.5 4 4.5
x 10
4
20
25
30
35
40
d. Multivariable High order model estimation
R. Humidity (%)
time (sec)
S1:Actual
S1:High order, Using K1,K2
Figure 8: T and H, actual, estimation using high order
multivariable model from K1, K2.
More important results are obtained when there
is a disturbance in vicinity of the SNs influences
some of the KSNs. fig. 9 shows the variation of T at
25000 seconds which affects both K1 and S1. Model
obtained from inlet-S1 can’t show this influence on
S1 because there are no influences on the inlet.
However, floating input method can estimate it
because at least one KSN senses it.
0 0.5 1 1.5 2 2. 5 3 3.5 4 4.5
x 10
4
0
5
10
a. Source and measurement results when there is a T disturbance on K1
Temperature (C)
time (sec)
K1: Measurement
T in inlet
K2: Measurement
0 0.5 1 1.5 2 2. 5 3 3.5 4 4.5
x 10
4
2
4
6
8
10
12
b. Disturbance effects with High order SISO model of K1-S1
Temperature (C)
time (sec)
S1:Estimated by inlet
S1:Estimated by K1
Figure 9: a. measured T in inlet, K1 and K2 and b.
estimation using inlet and K1with existing a disturbanc.
7 CONCLUDING REMARKS
This paper propuses a new hybrid model for
environmental conditions inside a container and
shows that it has much more accuracy for wide
range of parameter variations compared to other
conventional linear models between inlet and a
desired place. The new technique provides a
simplified multivariable model based on the
surrounding sensor nodes used for estimating the
ECs in the desired nodes. The simulation results and
mathematical proofs for different situations endorse
the capability of the proposed technique. At the end,
it should be noted that the comparison among
different multivariable estimation methods and their
implementations as well as finding the minimum
number and the best place of the KSNs are real
challenges main concerns on this issue.
REFERENCES
Ghiaus, C., Chicinas, A. and Inard, C., April 2007, ”Grey-
box identification of air-handling unit elements,
Control Engineering Practice”, Vol.15, Issue 4, pp.
421-433.
Shaikh, N. I and Prabhu, V., May 2007,”Mathematical
modeling and simulation of cryogenic tunnel
freezers”, Journal of Food Engineering, Vol. 80, Issue
2, pp 701-710.
Smale, N.J., Moureh, J. and Cortella, G., Sep. 2006, ”A
review of numerical models of airflow in refrigerated
food applications”, International Journal of
Refrigeration, Vol. 29, Issue 6, pp. 911-930.
Van Brecht, A., Quanten, S., Zerihundesta, T., Van
Buggenhout, S. and Berckmans, D., 20 Jan. 2005,
“Control of the 3-D spatio-temporal distribution of air
temperature”, International Journal of Control, 78:2,
pp. 88- 99.
Zerihun Desta, T., Van Brecht, A., Meyers, J., Baelmans,
M. and Berckmans, D., June 2004, ”Combining CFD
and data-based mechanistic (DBM) modeling
approaches, Energy and Buildings”, Vol. 36, Issue 6,
pp 535-542.
Frausto, H. U. and Jan G. Pieters, Jan.2004, ”Modeling
greenhouse temperature using system identification by
means of neural networks”, Neurocomputing, Vol. 56,
pp.423-428.
J. Moureh and Flick, D., Aug. 2004; ”Airflow pattern and
temperature distribution in a typical refrigerated truck
configuration loaded with pallets”, International
Journal of Refrigeration, V. 27, Issue 5, pp. 464-474.
Rouaud, O. and Havet, M., May 2002, ”Computation of
the airflow in a pilot scale clean room using K-
turbulence models”, International Journal of
Refrigeration, Vol. 25, Issue 3, pp. 351-361.
Sohlberg B., May 2002, Apr. 2003, ”Grey box modeling
for model predictive control of a heating process”,
Journal of Process Control, Vol. 13, Issue 3, pp. 225-
238.
J. Elson and D. Estrin. Sensor Networks: A Bridge to the
Physical World, chapter 1. Wireless Sensor Networks.
Kluwer Academic Publishers, 2004.
A NEW APPROACH FOR MODELING ENVIRONMENTAL CONDITIONS USING SENSOR NETWORKS
83