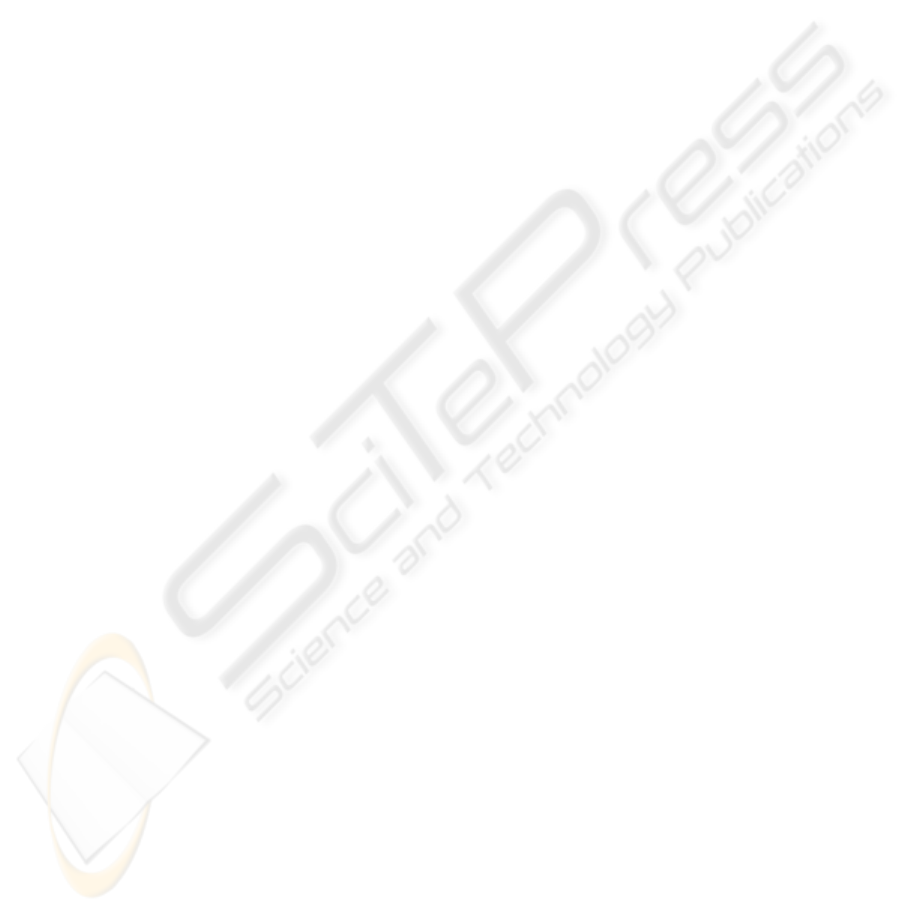
6 CONCLUSIONS
Although wastewater treatment plants are
implemented with properly functioning control
loops concerning the biological process, in practice,
this type of plant requires a major time investment
on the side of the operator, involving many manual
operations. These difficulties can be overcome by an
intelligent controller which incorporates the human
experience. The mined data, characterized as
multivariate and interrelated, constitutes a
combination of measurements of the process’s
variables and actions of the controller. The
consequences of the mining and knowledge
discovery procedure are used to adapt the soft
structure of the intelligent controller through a semi-
automatic scheme that provides deeper
understanding and better operation of the controlled
plant. The experimental results give us basic
directions to improve the operation of the control
system but it is obvious that a longer validation
period of data monitoring and processing is needed.
REFERENCES
Boverie, S, Demaya, B and Titli, A, 1991, Fuzzy logic
control compared with other automatic control
approaches, Proceedings of 30
th
CDC Conference,
Brighton.
Comas, J., Dzeroski, S., Gibert, K., Roda, I.R. and
Sanchez-Marre M., 2001. Knowledge discovery by
means of inductive methods in wastewater treatment
plant data”, AI Communications, Vol.14(1), pp.45-62.
Condras, P, Cook, J and Roehl E, 2002, “Estimation of
Tidal Marsh Loading Effects in a Complex Estuary”
American Water Resources Association Annual
Conference, New Orleans.
Condras, P and Roehl, E, 1999, Real-time control for
matching wastewater discharges to the assimilative
capacity of a complex tidally affected river basin
South Carolina Environmental Conference, Myrtle
Beach.
DeSilva, C W, 1995, Intelligent Control, Fuzzy Logic
Applications, CRC Press, Boca Raton.
Dixon, M., Gallop, J.R., Lambert, S.C., Lardon L., Steyer
P. and Healy J.V., 2004. Data mining to support
anaerobic WWT plant monitoring and control,
Proceedings of the IFAC Workshop on Modelling and
Control for Participatory Planning and Managing
Water Systems, Venice Italy,.
Dixon, M., Gallop, J.R., Lambert S.C. and Healy, J.V.,
2007. Experience with data mining for the anaerobic
wastewater treatment process, Environmental
Modelling & Software, Elsevier, , vl.22, pp.315-322.
Gibert, K., Sanchez-Marre, M. and Flores X., 2005.
Cluster discovery in environmental databases using
GESCONDA: The added value of comparisons, AI
Communications, Vol.18(4), pp.319-331.
Han, J and Kamber, M, 2001, Data Mining: Concepts and
Techniques, San Francisco, Morgan Kaufmann.
Harris, CJ, Moore, CG and Brown, M, 1993, Intelligent
control-aspects of fuzzy logic and neural nets, NJ,
World Scientific Publishers.
Huang, YC and Wang, XZ, 1999, Application of fuzzy
causal networks to wastewater treatment plants,
Chemical Engineering Science 54:2731-2738.
Jamshidi, M, Vadiee, N and Ross, TJ, 1993, Fuzzy Logic
and Control–Intelligentware and Hardware Applicati-
ons, NJ, Prentice Hall.
Katebi, R, Johnson, M and Wilkie, J, 2000, The future of
Advanced Control in Wastewater Treatment Plants,
Proceedings of the CIWEM Millennium Conference
Leeds UK.
King, R, 1992, Expert supervision and control of a large-
scale plant, Journal Intelligent and Robotic Systems,
2(3).
Lambert, S, 2004, (CCLRC-Data mining Resp.)
Telemonitoring and advanced tele-control of high
yield wastewater treatment plants, TELEMAC, R&D
project, No. IST-28156.
Mamdani, EH, 1974, An application of fuzzy algorithms
for the control of a dynamic plant, Proceedings IEE ,
121(12).
Manesis, S, Sapidis DJ and King RE, 1998, Intelligent
Control of Wastewater treatment Plants, Journal
Artificial Intelligence in Engineering (12):275-281.
Patyra, MJ and Mylnak, DM, 1996, Fuzzy Logic
Implementation and Application, NJ, J. Wiley-
Teubner.
Rodriguez-Roda, I, Sanchez-Marre, M, Comas, J, Baeza,
J, Colprim, J, Lafuente, J, Cortes, U and Poch M,
2002, A Hybrid Supervisory System to Support
WWTP Operation: Implementation and Validation,
Water Science and Technology Journal (45): 289-297.
Sanguesa, R, Cortés, U and Béjar, J, 1997, Causal
dependency discovery with posscause: an application
to wastewater treatment plants, Proceedings of the 1
st
International Conference on the practical application
of knowledge discovery and data mining, London.
Serra, P, Sànchez, M, Lafuente, J, Cortès, U and Poch, M,
1994, DEPUR: a knowledge-based tool for wastewater
treatment plants, Engineering Applications of
Artificial Intelligence, 7(1):23-30.
Vazirgiannis, M and Halkidi, M, 2000, Data Mining:
Concepts and Techniques, Athens University of
Economics and Business, TR HELDiNET 10.
Victor Ramos, J, Goncalves, C and Durado, A, 2004, On-
line Extraction of Fuzzy Rules in a Wastewater
Treatment Plant, Proceedings of the 1
st
International
Conference on Artificial Intelligence Applications and
Innovations, 1:87-102.
DATA MINING AND KNOWLEDGE DISCOVERY FOR MONITORING AND INTELLIGENT CONTROL OF A
WASTEWATER TREATMENT PLANT
93