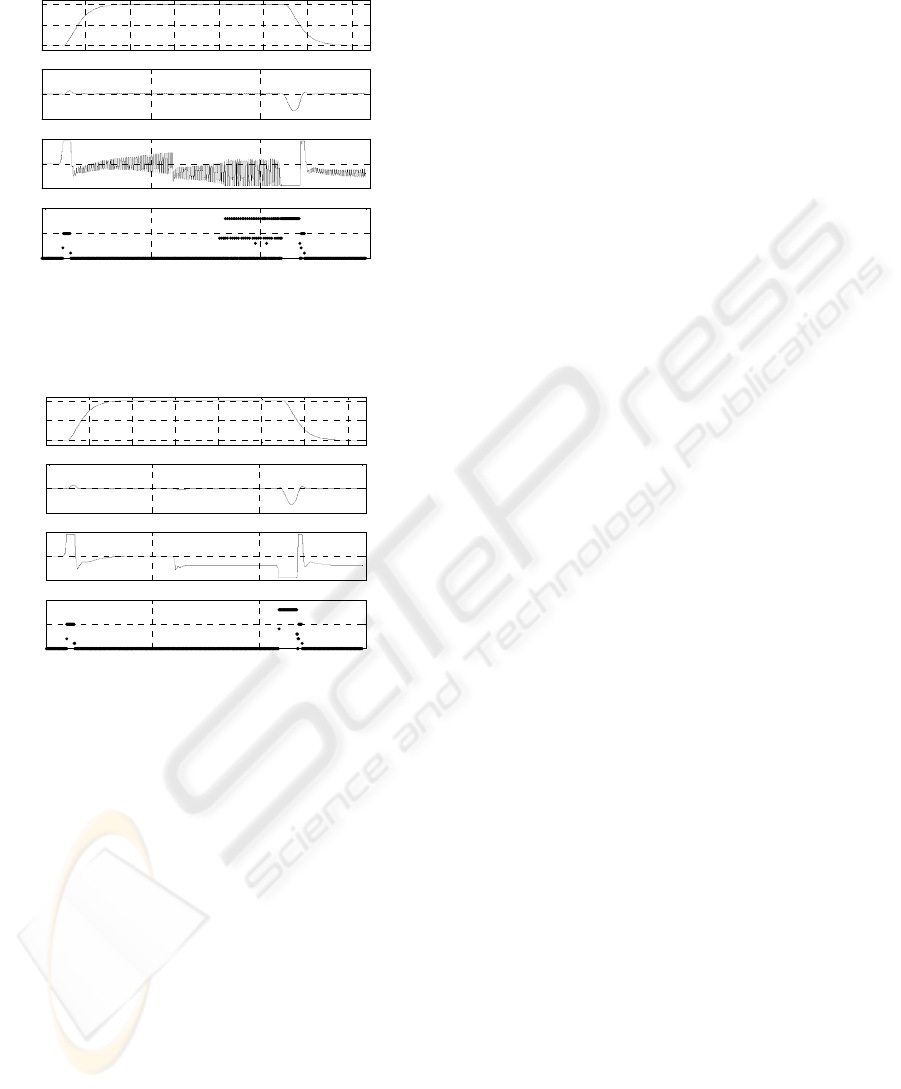
behaviour towards uncertainties in high frequency
and the continuity between regions is guaranteed.
0 0.2 0.4 0.6 0.8 1 1.2 1.4
0
0.5
1
Pos. Ref.
(rad)
0 0.5 1 1.5
-0.2
0
0.2
Pos. Error
(rad)
0 0.5 1 1.5
-2
0
2
Control
signal
0 0.5 1 1.5
0
5
10
Active
Region
time (s)
Figure 3: Position reference, position error, control signal
and active region for the initial controller and uncertain
model.
0 0.2 0.4 0.6 0.8 1 1.2 1.4
0
0.5
1
Pos. Ref.
(rad)
0 0.5 1 1.5
-0.2
0
0.2
Pos. Error
(rad)
0 0.5 1 1.5
-2
0
2
Control
signal
0 0.5 1 1.5
0
5
10
Active
Region
time (s)
Figure 4: Position reference, position error, control signal
and active region for the robustified controller and
uncertain model.
6 CONCLUSIONS
The paper investigated the robustification methods
for the control laws obtained in a constrained
predictive control framework. The idea is to design
in a first instance a piecewise polynomial controller
which satisfy the basic demands in terms of tracking
performances. In a second stage, the same predictive
control structure (prediction horizon, weightings,
etc.) is robustified using the model arguments
accounting for the noise influence. The idea is
similar to that of using a fixed observer, but
exploring all the class of stabilizing controllers of
the unconstrained system. This increases the number
of degrees of freedom.
The robustification of initial unconstrained
controller is made using the Youla-Kučera
parametrization, and then this robustification is
expanded to all the piecewise structure of the
controller. For this, the noise model corresponding
to the Youla-Kučera parameter is found, and use to
regenerate the robust piecewise controller by
preserving the same input/output behavior but being
more robust.
The limitations of the method are in the existence
of the corresponding noise model of the Youla-
Kučera parameter. This is transparent in the
resolution of a non linear equation system. The
robustification being done off-line, any infeasibility
can be handled by retuning the GPC parameters.
REFERENCES
Ansay P., M. Gevers and V. Wertz (1998). Enhancing the
robustness of GPC via a simple choice of the Youla
parameter. European Journal of Control, 4, 64-70.
Bemporad A., C. Filippi (2006). An Algorithm for
Approximate Multiparametric Convex Programming.
Computational Optimization and Applications, 35, 87-
108.
Bemporad A., F. Borelli and M. Morari (2002a). Model
predictive control based on linear programming: The
explicit solution. IEEE Transactions on Automatic
Control, 47, 1974–1985.
Bemporad A., M. Morari, V. Dua and E. Pistikopoulos
(2002b). The explicit linear quadratic regulator for
constrained systems. Automatica, 38, 3–20.
Bitmead R.R., M. Gervers and V. Wertz (1990).
Adaptative optimal control. The thinking Man’s GPC.
Prentice Hall. Englewood Cliffs, N.J.
Camacho E.F., C. Bordons, “Model predictive control”,
Springer-Verlag, London, 2nd ed., 2004.
Clarke D. W., C. Mohtadi and P.S. Tuffs (1987).
Generalized predictive control – Part I and II.
Automatica, 23(2), 137-160.
Clarke D.W. and R. Scattolini (1991). Constrained
receding-horizon predictive control. IEE Proceedings-
D, 138(4).
Ehrlinger A., P. Boucher and D. Dumur (1996). Unified
Approach of Equality and Inequality Constraints in
G.P.C. 5th IEEE Conference on Control Applications.
Goodwin, G.C., M.M. Seron, J.A. De Dona (2004).
Constrained Control and Estimation, Springer-Verlag,
London.
Kerrigan E. and J.M. Maciejowski (2004). Feedback min-
max model predictive control using a single linear
program: robust stability and the explicit solution.
International Journal of Robust and Nonlinear
Control, 14, 395-413.
Kouvaritakis B, J.A. Rossiter and A.O.T. Chang (1992).
Stable generalized predictive control: an algorithm
with guaranteed stability. IEE Proceedings-D, 139(4),
349-362.
ICINCO 2008 - International Conference on Informatics in Control, Automation and Robotics
100