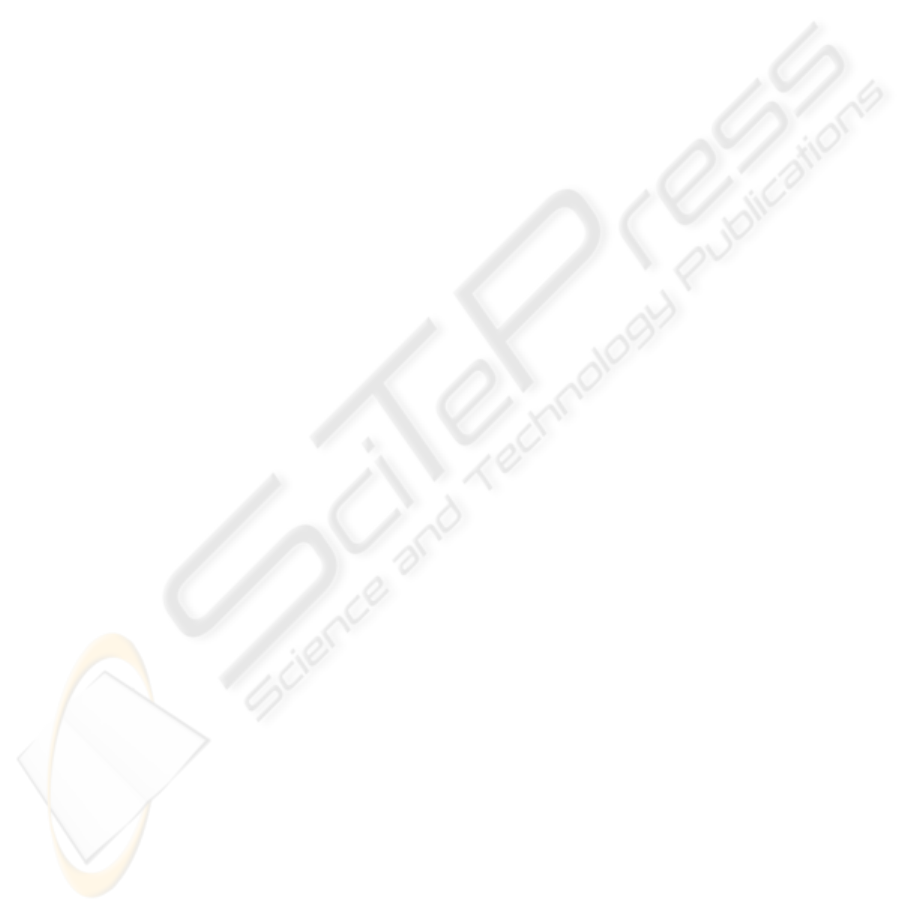
functions. This proposed method always guarantees
the positivity of the covariance of the errors and al-
lows for considering multiplicative noise on both the
state and control of the system.
The proposed probabilistic DHP critic method is
suitable for deterministic and stochastic control prob-
lems characterized by functional uncertainty. Unlike
current established control methods, it takes uncer-
tainty of the forward model and inverse controller into
consideration when deriving the optimal control law.
Theoretical development in this paper is demon-
strated through linear quadratic control problem.
There, the correct value of the cost function which
satisfies the Bellman equation is evaluated and shown
to be equal to its corresponding value produced by the
proposed probabilistic critic network.
REFERENCES
Botto, M. A., Wams, B., van den Boom, and da Costa,
J. M. G. S. (2000). Robust stability of feedback lin-
earised systems modelled with neural networks: Deal-
ing with uncertainty. Engineering Applications of Ar-
tificial Intelligence, 13(6):659–670.
Fabri, S. and Kadirkamanathan, V. (1998). Dual adaptive
control of nonlinear stochastic systems using neural
networks. Automatica, 34(2):245–253.
Ge, S. S., Hang, C. C., Lee, T. H., and Zhang, T. (2001). Sta-
ble Adaptive Neural Network Control. Kluwer, Nor-
well, MA.
Ge, S. S. and Wang, C. (2004). Adaptive neural control
of uncertain mimo nonlinear systems. IEEE Transac-
tions on Neural Networks, 15(3):674–692.
Herzallah, R. (2007). Adaptive critic methods for stochas-
tic systems with input-dependent noise. Automatica.
Accepted to appear.
Herzallah, R. and Lowe, D. A Bayesian perspective on sto-
chastic neuro control. IEEE Transactions on Neural
Networks. re-submited 2006.
Herzallah, R. and Lowe, D. (2007). Distribution model-
ing of nonlinear inverse controllers under a Bayesian
framework. IEEE Transactions on Neural Networks,
18:107–114.
Hovakimyan, N., Nardi, F., and Calise, A. J. (2001). A
novel observer based adaptive output feedback ap-
proach for control of uncertain systems. In Proceed-
ings of the American Control Conference, volume 3,
pages 2444–2449, Arlington, VA, USA.
Howard, R. A. (1960). Dynamic Programming and Markov
Processes. The Massachusetts Institute of Technology
and John Wiley and Sons, Inc., New York. London.
Karny, M. (1996). Towards fully probabilistic control de-
sign. Automatica, 32(12):1719–1722.
Lewis, F. L., Yesildirek, A., and Liu, K. (2000). Robust
backstepping control of induction motors using neural
netwoks. IEEE Transactions on Neural Networks,
11:1178–1187.
Mine, H. and Osaki, S., editors (1970). Markovian Decision
Processes. Elsevier, New York, N.Y.
Murray-Smith, R. and Sbarbaro, D. (2002). Nonlinear adap-
tive control using non-parametric gaussian process
prior models. In 15th IFAC Triennial World Congress,
Barcelona.
Sanner, R. M. and Slotine, J. J. E. (1992). Gaussian net-
works for direct adaptive control. IEEE Transactions
on Neural Networks, 3(6).
Sastry, S. S. and Isidori, A. (1989). Adaptive control of
linearizable systems. IEEE Transactions on Automatic
Control, 34(11):1123–1131.
Wang, D. and Huang, J. (2005). Neural network-based
adaptive dynamic surface control for a class of uncer-
tain nonlinear systems in strict-feedback form. IEEE
Transactions on Neural Networks, 16(1):195–202.
Wang, H. (2002). Minimum entropy control of non-
gaussian dynamic stochastic systems. IEEE Transac-
tions on Automatic Control, 47(2):398–403.
Wang, H. and Zhang, J. (2001). Bounded stochastic dis-
tribution control for pseudo armax stochastic systems.
IEEE Transactions on Automatic Control, 46(3):486–
490.
Werbos, P. J. (1992). Approximate dynamic programming
for real-time control and neural modeling. In White,
D. A. and Sofge, D. A., editors, Handbook of In-
tillegent Control, chapter 13, pages 493–526. Multi-
science Press, Inc, New York, N.Y.
Zhang, Y., Peng, P. Y., and Jiang, Z. P. (2000). Stable neural
controller design for unknown nonlinear systems us-
ing backstepping. IEEE Transactions on Neural Net-
works, 11:1347–1359.
ICINCO 2008 - International Conference on Informatics in Control, Automation and Robotics
288