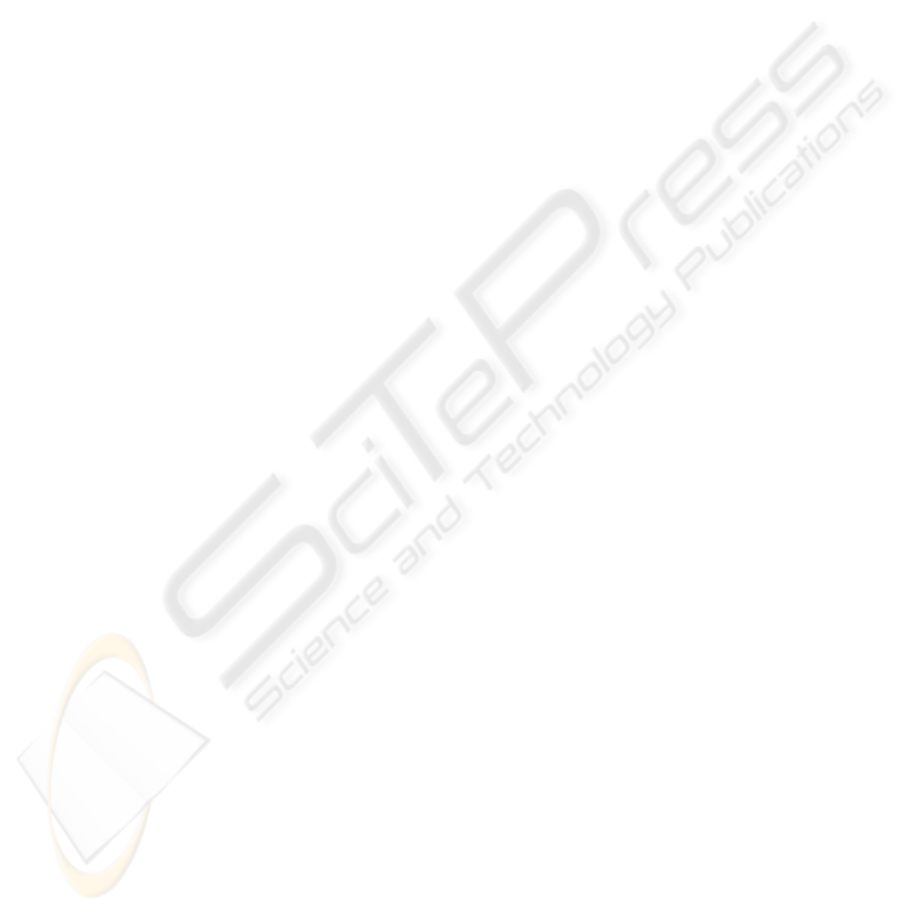
less, in operations research it was quantified with var-
ious solution concepts (Denda et al., 2000). The eq-
uitable optimization with the preference structure that
complies with both the efficiency (Pareto-optimality)
and with the Pigou-Dalton principle of transfers may
be used to formalize the fair solution concepts. Mul-
tiple criteria models equivalent to equitable optimiza-
tion allows to generate a variety of fair and efficient
resource allocation patterns (Kostreva et al., 2004;
Ogryczak et al., 2008).
In this paper we haveanalyzed how scalar inequal-
ity measures can be used to guarantee the fair consis-
tency. It turns out that several inequality measures can
be combined with the mean itself into the optimiza-
tion criteria generalizing the concept of the worst out-
come and generating fairly consistent underachieve-
ment measures. We have shown that properties of
convexity and positive homogeneity together with be-
ing bounded by the maximum downside semidevia-
tion are sufficient for a typical inequality measure to
guarantee the corresponding fair consistency. It al-
lows us to identify various inequality measures which
can be effectively used to incorporate fairness fac-
tors into various resource allocation problems while
preserving the consistency with outcomes maximiza-
tion. Among others, the standard semideviation and
the mean semideviation turn out to be such a consis-
tent inequality measure while the mean absolute dif-
ference is strongly consistent.
Our analysis is related to the properties of solu-
tions to resource allocation models. It has been shown
how inequality measures can be included into the
models avoiding contradiction to the maximization of
outcomes. We do not analyze algorithmic issues for
the specific resource allocation problems. Generally,
the requirement of convexity necessary for the consis-
tency, guarantees that the corresponding optimization
criteria belong to the class of convex optimization, not
complicating the original resource allocation model
with any additional discrete structure. Many of the in-
equality measures, we analyzed, can be implemented
with auxiliary linear programming constraints. Nev-
ertheless, further research on efficient computational
algorithms for solving the specific models is neces-
sary.
REFERENCES
Atkinson, A. (1970). On the measurement of inequality. J.
Economic Theory, 2:244–263.
Bonald, T. and Massoulie, L. (2001). Impact of fairness on
internet performance. In Proceedings of ACM Sigmet-
rics, pages 82–91.
Denda, R., Banchs, A., and Effelsberg, W. (2000). The
fairness challenge in computer networks. Lect. Notes
Comp. Sci., 1922:208–220.
Ibaraki, T. and Katoh, N. (1988). Resource Allocation
Problems, Algorithmic Approaches. MIT Press, Cam-
bridge.
Kelly, F., Mauloo, A., and Tan, D. (1997). Rate con-
trol for communication networks: shadow prices, pro-
portional fairness and stability. J. Opnl. Res. Soc.,
49:206–217.
Kleinberg, J., Rabani, Y., and Tardos, E. (2001). Fairness
in routing and load balancing. J. Comput. Syst. Sci.,
63:2–21.
Kostreva, M. M. and Ogryczak, W. (1999). Linear opti-
mization with multiple equitable criteria. RAIRO Op-
erations Research, 33:275–297.
Kostreva, M. M., Ogryczak, W., and Wierzbicki, A. (2004).
Equitable aggregations and multiple criteria analysis.
Eur. J. Opnl. Res., 158:362–377.
Luss, H. (1999). On equitable resource allocation problems:
A lexicographic minimax approach. Operations Re-
search, 47:361–378.
Marchi, E. and Oviedo, J. A. (1992). Lexicographic op-
timality in the multiple objective linear programming:
the nucleolar solution. Eur. J. Opnl. Res., 57:355–359.
Ogryczak, W. (2000). Inequality measures and equitable
approaches to location problems. Eur. J. Opnl. Res.,
122:374–391.
Ogryczak, W., Pi´oro, M., and Tomaszewski, A. (2005).
Telecommunications network design and max-min
optimization problem. J. Telecom. Info. Tech., 3:1–14.
Ogryczak, W. and
´
Sliwi´nski, T. (2006). On direct methods
for lexicographic min-max optimization. Lect. Notes
Comp. Sci., 3982:774–783.
Ogryczak, W., Wierzbicki, A., and Milewski, M. (2008). A
multi-criteria approach to fair and efficient bandwidth
allocation. OMEGA, 36:451–463.
Pi´oro, M. and Medhi, D. (2004). Routing, Flow and Ca-
pacity Design in Communication and Computer Net-
works. Morgan Kaufmann, San Francisco.
Rawls, J. (1971). The Theory of Justice. Harvard Univ.
Press, Cambridge.
Rawls, J. and Kelly, E. (2001). Justice as Fairness: A Re-
statement. Harvard Univ. Press, Cambridge.
Rothschild, M. and Stiglitz, J. E. (1973). Some further re-
sults in the measurement of inequality. J. Econ. The-
ory, 6:188–204.
Young, H. P. (1994). Equity in Theory and Practice. Prince-
ton Univ. Press, Princeton.
ICINCO 2008 - International Conference on Informatics in Control, Automation and Robotics
156