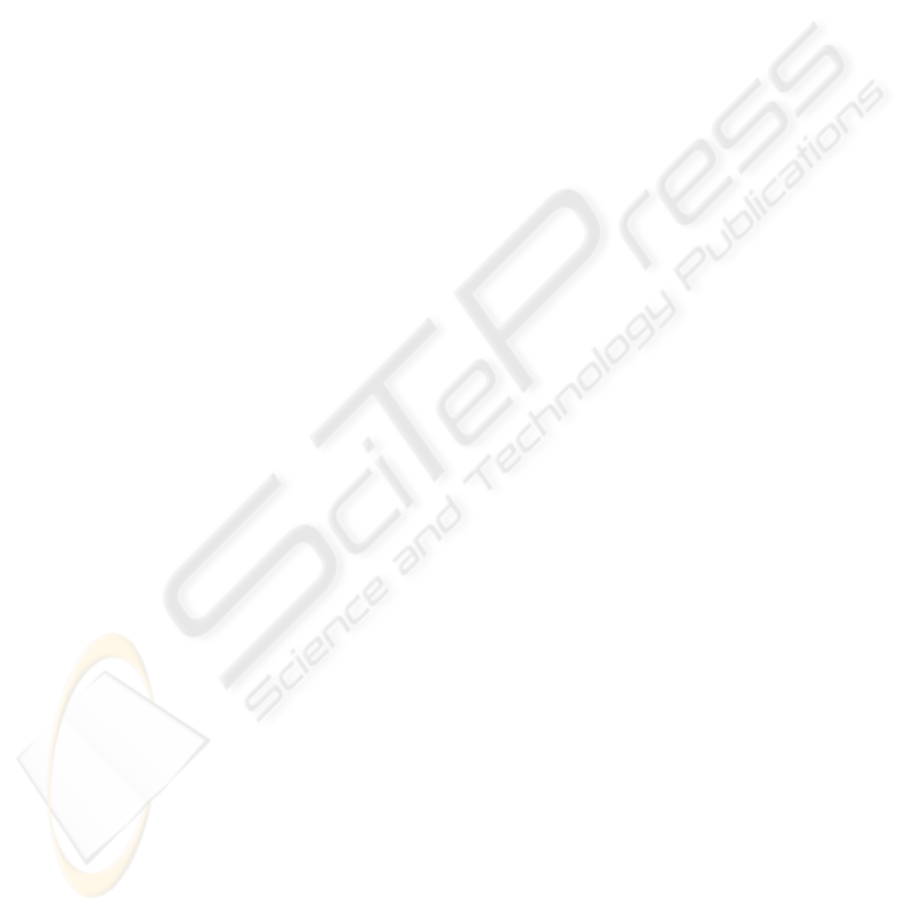
EEG values are directly correlated to valence, inde-
pendently of their initial standard level. The data pro-
vided suggests that beta and gamma waves strongly
seam to vary directly with valence, enabling, indi-
rectly and with conjugation with other inputs, emo-
tional state detection.
Despite the described positive outcome, there
were identified some features that, although did not
match the initial assumptions, have already been sub-
ject of turnaround strategy definition. The first one
is related to the inexistence of generic significative
changes in heart rate values along experimental ses-
sions and the second resides in the fact that the ex-
pected GSR readings curve – high conductivity with
high arousal situations – was not recorded as often
as predicted. The authors believe that the existence
of these issues is grounded on the fact that the de-
signed emotionally-evocative sessions based only on
pictures do not trigger such strong emotions capable
of significantly influence biosignals such as heart rate.
It is believed that it would be necessary much strong
multimedia content provided in a more immersive en-
vironment so that subjects could be more deeply in-
volved.
4.1 Future Work
The main future work topics are not only related to
this particular study, once it is a spin off/module of a
major one, but also with the main global project. With
this in mind, there were identified the following areas:
• More Sophisticated Equipment Reinforcement: It
is intended to acquire more sophisticated equip-
ment, specially and specifically in what concerns
to a multi-channel EEG and a more sensible and
reliable GSR;
• Equipment Diversity: It would be useful to inte-
grate in the developed framework new equipments
capable of reading and extract more biosignals,
namely pupil dilatation, voice analysis and facial
expression recognition;
• More Detailed Emotion Classification: using the
depicted key factors with conjugation with others
provided by the study continuation and data vol-
ume and diversity enhancement brought, by new
equipment acquisition, it would be plausible to
perform automatic subject emotion classification
with deeper detail levels.
• Software Control: The accomplishment of the
previous items would enable both conscious and
subconscious control of several tools and/or mul-
timedia contents;
Considering the studied problem as a whole, specif-
ically the emotion classification topic, several practi-
cal domain applications are not only feasible but also
attractive. Most of the immediate technology adapta-
tions shall reside in the entertainment industry, both in
audiovisual and videogame branches through multi-
media content adaptability to user’s emotional states.
Other possible application areas are user interface en-
hancement, direct advertising and medical applica-
tions, namely in phobia treatments and psychological
evaluations.
REFERENCES
A. Mehrabian, J. R. (1974). An approach to environmental
psychology. In The MIT Press.
Aftanas, L. (1997). Nonlinear forecasting measurements
of the human eeg during evoked emotions. In Brain
Topography, volume 10, pages 155–162.
Ebersole, J. (2002). Current Practice of Clinical Electroen-
cephalography. Lippincott Williams & Wilkins.
G. Chanel, J. Kronegg, D. G. (2005). Emotion assessment:
Arousal evalutation using eeg’s and peripheral physi-
ological signals. In Technical Report.
J. Allen, J. K. (2006). Frontal eeg asymmetry, emotion, and
phychopathology: the first, and the next 25 years. In
Biological Psychology, volume 67, pages 1–5.
K. Ishino, M. H. (2003). A feeling estimation system us-
ing a simple electroencephalograph. In Proceedings
of 2003 IEEE Int. Conference on Systems, Man, and
Cybernetics, pages 4204–4209.
L. Aftanas, A. A. (2001). Time-dependent cortical asym-
metries induced by emotional arousal: Eeg analysis of
event-related synchronization and desynchronization
in individually defined frequency bands. In Int. Jour-
nal of Psychophysiology, volume 44, pages 67–82.
Leuthardt, E. (2004). A braincomputer interface using elec-
trocorticographic signals in humans. In Journal of
Neural Engineering, pages 63–71.
Logothetis, N. (1957). The measurement of meaning. In
University of Illinois Press.
M. Muller, A. K. (1999). Processing of affective pictures
modulates right-hemispheric gamma band eeg activ-
ity. In Clinical Neurophysiology, volume 110, pages
1913–1920.
Pascalis, V. D. (1998). Eeg activity and heart rate during
recall of emotional events in hypnosis: relationships
with hypnotizability and suggestibility. In Int. Journal
of Psychophysiology, volume 29, pages 255–275.
P.J. Lang, M. Bradley, B. C. (2005). International affective
picture system (iaps): Affective ratings of pictures and
instruction manual. In Technical Report.
Takahashi, K. (2004). Remarks on emotion recognition
from bio-potencial signals. In The second Int. Con-
ference on Autonomous Robots and Agents.
ICINCO 2008 - International Conference on Informatics in Control, Automation and Robotics
252