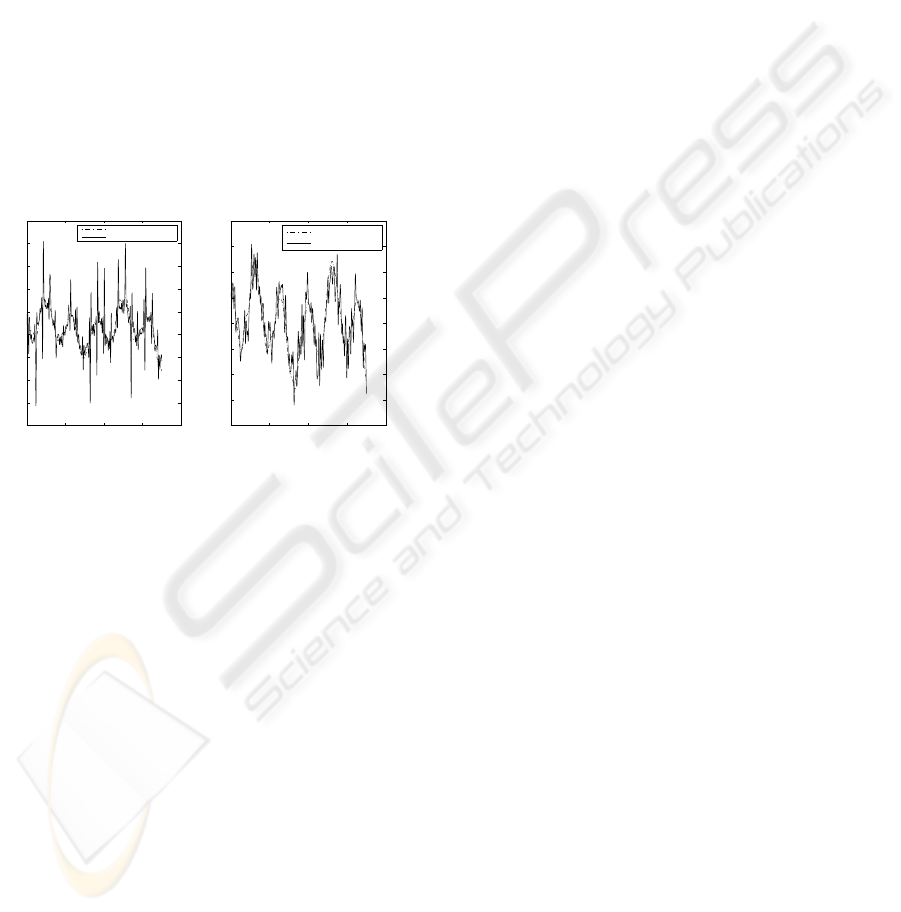
Note that the dynamic parameters present in most
cases a relative standard deviation lower than 10%,
which represents a good estimation. However the rel-
ative standard deviation of the parameters MZ, and
F
S5
is higher than 10%. This deviation is due to me-
chanical constraints, consequently, we conclude that
the obtained results are encouraging and we can state
that these identification results are globally accept-
able.
In order to validate the estimated dynamic param-
eters, we proceed to a cross validation which con-
sists in comparing the measured torques with those
obtained by the inverse dynamic model with the iden-
tified parameters. The trajectory which is usedfor this
validation has not been used previously for the iden-
tification. Figure 4 show the results of this validation.
For the others axis, we have also obtained the same
ting than figure 4
0 1000 2000 3000 4000
−8
−6
−4
−2
0
2
4
6
8
10
Time [ms]
Torque 1 [N.m]
0 1000 2000 3000 4000
−4
−3
−2
−1
0
1
2
3
4
Time [ms]
Torque 2 [N.m]
Estimated torque 2
Measured torque 2
Estimated torque 1
Measured torque 1
Figure 4: Estimated and measured torque for the joints 1
and 2.
Note that the calculated torques using the inverse
dynamic model with the estimated parameters are
close to those measured on the robot. Consequently,
one can conclude that the estimation of the dynamic
parameters, using the least squares method is valid.
7 CONCLUSIONS
In this paper, we identified the physical parameters
of the C5 parallel robot. The identification is based
on the least squares method. The application of this
identification method uses an exciting trajectory cal-
culated from a heuristic approach. To validate the
identified parameters, we considered another trajec-
tory different from that used in identification. The
cross validation enables us to conclude with the ef-
fectiveness of the considered identification. In short
term of our project, we propose to include the joint
elasticity, which is the major source of flexibility in
many practical applications.
REFERENCES
Swevers J., Ganseman C., Bilgin D., De Schutter J., Van
Brussel H., 1997. Optimal robot excitation and identi-
fication. IEEE Transactions on Robotics and Automa-
tion, 13(5):730–740.
Renaud, P., Vivas, A., Andreff, A., Poignet, P., 2006. Mar-
tinet, P., Pierrot, F., Company, O., Kinematic and dy-
namic identification of parallel mechanisms, In Con-
rol Engineering Practice 14, pp1099 - 1109.
Poignet, P., Ramdani, N., Vivas, A., 2003. Robust estima-
tion of parallel robot dynamic parameters with inter-
val analysis, Proceedings of the 42nd IEEE Confer-
ence on Decision and Control, pp. 6503-6508, Maui,
Hawaii, USA.
Khalil, W., Ibrahim, O., 2004. General Solution for the Dy-
namic Modeling of Parallel Robots, lnternational Con-
ference on Robotics & Automation, New Orleans, LA.
Gautier, M., Poignet, P., 2002. Identification en boucle
ferm´ee par mod`ele inverse des param`etres physiques
de syst`emes m´ecatroniques, Journal Eurepeen des
Syst`emes Automatis´es,36:465-480.
Gautier M., 1991. Numerical calculation of the base inertial
parameters, Journal of Robotics Systems, Vol. 8, No.
4, pp. 485-506.
An, C. H., Atkenson, C. G., Hollerbach, J. H., 1985. Es-
timation of inertial parameters of rigid body links of
manipulators, Proceedings of the 24th Conference on
Decision and control, pp. 990-995.
Janot, A., Bidard, C., Gosselin, F., Gautier, M., Keller, D.,
Perrot, Y., 2007. Modeling and Identification of a 3
DOF Haptic Interface,IEEE International Conference
on Robotics and Automation Roma, Italy, pp 4949-
4955
ICINCO 2008 - International Conference on Informatics in Control, Automation and Robotics
70