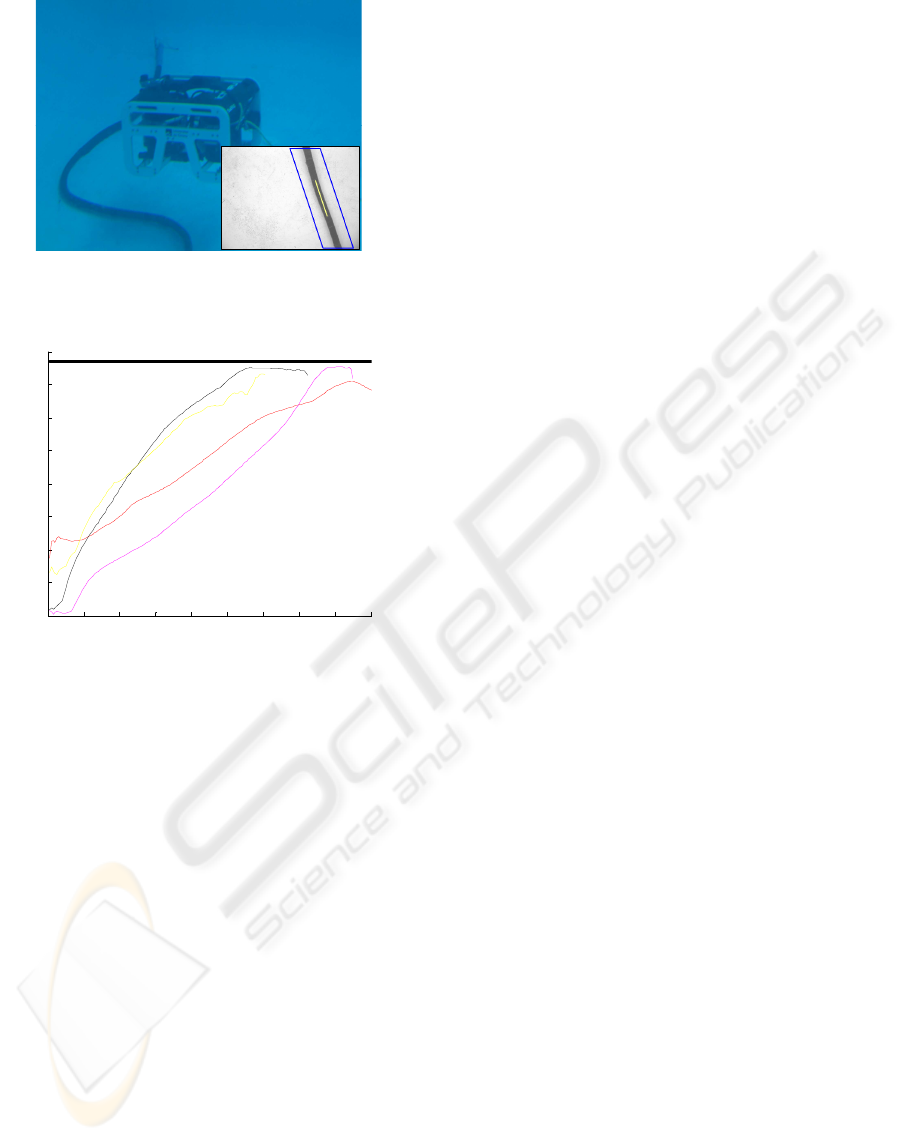
Figure 10: ICTINEU
AUV
in the test pool. Small bottom-
right image: Detected cable.
0 20 40 60 80 100 120 140 160 18
0.8
0.9
1
1.1
1.2
1.3
1.4
1.5
1.6
Number of Iterations
Theta angle (Radiants)
Real Variation of the Theta angle while attempting to center the cable
Figure 11: Real measured trajectories of the θ angle of the
image plane while attempting to center the cable.
ACKNOWLEDGEMENTS
This work has been financed by the Spanish Govern-
ment Comission MCYT, project number DPI2005-
09001-C03-01, also partially funded by the MO-
MARNET EU project MRTN-CT-2004-505026 and
the European Research Training Network on Key
Technologies for Intervention AutonomousUnderwa-
ter Vehicles FREESUBNET, contract number MRTN-
CT-2006-036186.
REFERENCES
Aberdeen, D. A. (2003). Policy-Gradient Algorithms
for Partially Observable Markov Decision Processes.
PhD thesis, Australian National University.
Anderson, C. (2000). Approximating a policy can be easier
than approximating a value function. Computer sci-
ence technical report, University of Colorado State.
Antich, J. and Ortiz, A. (2003). Underwater cable track-
ing by visual feedback. In First Iberian Conference
on Pattern recognition and Image Analysis (IbPRIA,
LNCS 2652), Port d’Andratx, Spain.
Atkenson, C., Moore, A., and Schaal, S. (1997). Lo-
cally weighted learning. Artificial Intelligence Re-
view, 11:11–73.
Bagnell, J. and Schneider, J. (2001). Autonomous he-
licopter control using reinforcement learning policy
search methods. In Proceedings of the IEEE Interna-
tional Conference on Robotics and Automation, Ko-
rea.
Baxter, J. and Bartlett, P. (1999). Direct gradient-based rein-
forcement learning: I. gradient estimation algorithms.
Technical report, Australian National University.
El-Fakdi, A., Carreras, M., and Ridao, P. (2006). Towards
direct policy search reinforcement learning for robot
control. In IEEE/RSJ International Conference on In-
telligent Robots and Systems.
Hammer, B., Singh, S., and Scherer, S. (2006). Learning
obstacle avoidance parameters from operator behav-
ior. Journal of Field Robotics, Special Issue on Ma-
chine Learning Based Robotics in Unstructured Envi-
ronments, 23 (11/12).
Haykin, S. (1999). Neural Networks, a comprehensive foun-
dation. Prentice Hall, 2nd ed. edition.
Kohl, N. and Stone, P. (2004). Policy gradient reinforce-
ment learning for fast quadrupedal locomotion. In
IEEE International Conference on Robotics and Au-
tomation (ICRA).
Konda, V. and Tsitsiklis, J. (2003). On actor-critic algo-
rithms. SIAM Journal on Control and Optimization,
42, number 4:1143–1166.
Lin, L. (1992). Self-improving reactive agents based on re-
inforcement learning, planning and teaching. Machine
Learning, 8(3/4):293–321.
Marbach, P. and Tsitsiklis, J. N. (2000). Gradient-based op-
timization of Markov reward processes: Practical vari-
ants. Technical report, Center for Communications
Systems Research, University of Cambridge.
Matsubara, T., Morimoto, J., Nakanishi, J., Sato, M., and
Doya, K. (2005). Learning sensory feedback to CPG
with policy gradient for biped locomotion. In Pro-
ceedings of the International Conference on Robotics
and Automation ICRA, Barcelona, Spain.
Meuleau, N., Peshkin, L., and Kim, K. (2001). Explo-
ration in gradient based reinforcement learning. Tech-
nical report, Massachusetts Institute of Technology,
AI Memo 2001-003.
Ortiz, A., Simo, M., and Oliver, G. (2002). A vision system
for an underwater cable tracker. International Journal
of Machine Vision and Applications, 13 (3):129–140.
Ribas, D., Palomeras, N., Ridao, P., Carreras, M., and Her-
nandez, E. (2007). Ictineu auv wins the first sauc-
e competition. In IEEE International Conference on
Robotics and Automation.
Ridao, P., Tiano, A., El-Fakdi, A., Carreras, M., and Zirilli,
A. (2004). On the identification of non-linear models
of unmanned underwater vehicles. Control Engineer-
ing Practice, 12:1483–1499.
LEARNING BY EXAMPLE - Reinforcement Learning Techniques for Real Autonomous Underwater Cable Tracking
67