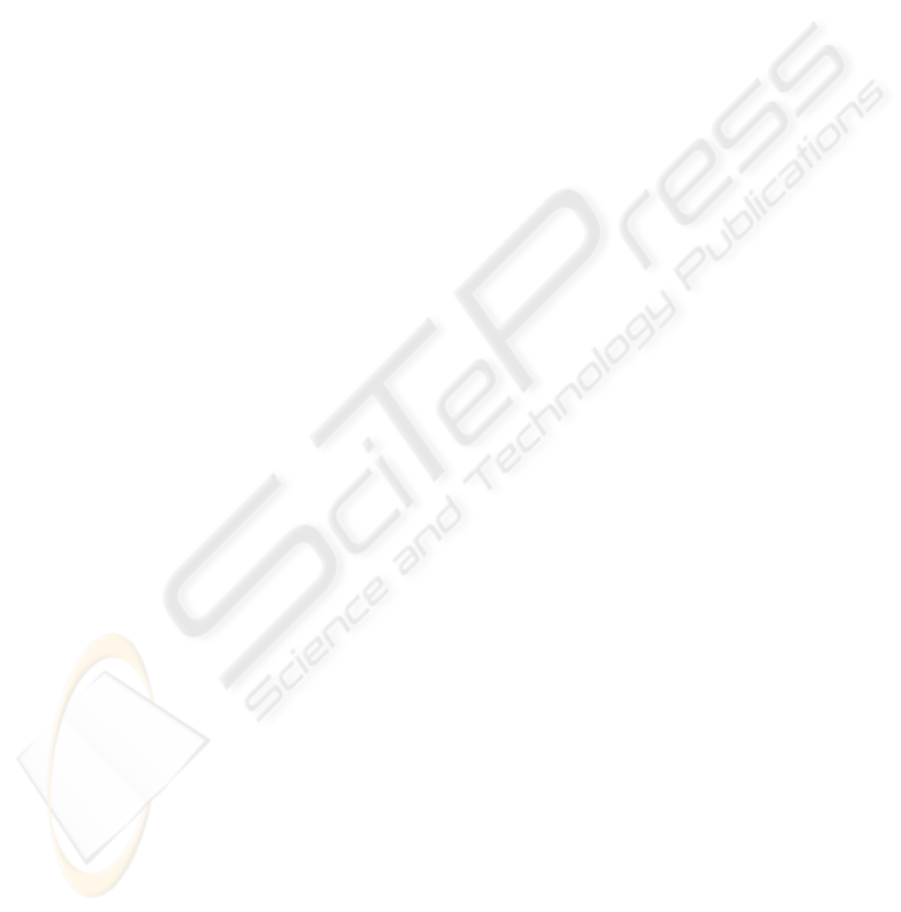
6 CONCLUSIONS
This paper proposes a new identification criterion for
multi-dimensional system disturbed by color noise,
and further develops a recursive algorithm, MSDCN,
based on it. Weighting both on the prediction er-
rors and the difference of each prediction error, the
identification criterion makes the weighting matrix
definite in calculation. Based on this performance
criterion, MSDCN is developed using a two-step
method to estimate both the system parameters and
the noise part. The MSDCN algorithm has high anti-
disturbance performance in the prediction of multi-
dimensional systems disturbed by color noise. It both
decreased the estimation errors and got smooth pre-
diction curves. The performance of the MSDCN al-
gorithm was demonstrated by simulations.
ACKNOWLEDGEMENTS
This work is supported by the Funds NSFC60672110,
NSFC60474026,and the JSPS Foundation.
REFERENCES
Andersson, A. and Broman, H. (1998), A second-order re-
cursive algorithm with applications to adaptive filter-
ing and subspace tracking. IEEE Trans. Signal Pro-
cessing, vol. 46, pp. 1720C1725, June.
Griffith Jr, D. W. and Arce, G. R. (1999), A partially decou-
pled RLS algorithm for volterra filters. IEEE Trans.
Signal Processing, vol. 47, pp. 579C582, Feb.
Kuo, C. J. , Jr, R. D. , Lin, C. Y. and Tsai, Y. C. (2000), Set-
theoretic estimation based on a priori knowledge of
the noise distribution. IEEE Trans. Signal Processing,
vol. 48, pp. 2150C2156, July.
Lo, K. M. , et al. (2006), Empirical frequency-domain
optimal parameter estimate for Black-box processes.
IEEE Transactions on Circuits and Systems-I: Regu-
lar Papers, vol.53, No.2, 419-430.
Lo, K. M. , Kwon, W. H. (2003), New identification ap-
proaches for disturbed models. Automatica.vol.39,
No.9, 1627-1634.
Lo, K. M. , Kimura, H. (2003), Recursive Estimation Meth-
ods for Discrete Systems. IEEE Trans. On Circruits
and Systems.vol.49, NO.6, No.6, 439-446.
Ljung, L. (1985), On the estimation of transfer function.
Automaticavol. 21, 677-696.
Ljung, L. (1999), System Identification: Theory for the
User. Upper Saddle River,NJ: Prentice-Hall, 1999.
Mershed, R. and Sayed, A. J. (2000), Order-recursive RLS
Laguerre adaptive filtering. IEEE Trans. Signal Pro-
cessing, vol. 48, pp. 3000C3010, July.
Stoica, P., Friedlander, B.and Soderstrom, T.(1987) Op-
timal Intrumental Variable Multistep Algorithms for
Estimation of the AR Parameters of an ARMA Pro-
cess, Int. J. Control 45(1987), 2083-2107.
Stoica, P. and Jansson, M.(2001) Estimating Optimal
Weights for Instrumental Variable Methods. Digital
Signal Processing - A Review Journal vol. 11, no. 3,
pp. 252-268, Jul. 2001.
Trump, T. (2001), Maximum likelihood trend estimation
in exponential noise. IEEE Trans. Signal Processing,
vol. 49, pp. 2087C2095, Sept.
ICINCO 2008 - International Conference on Informatics in Control, Automation and Robotics
148