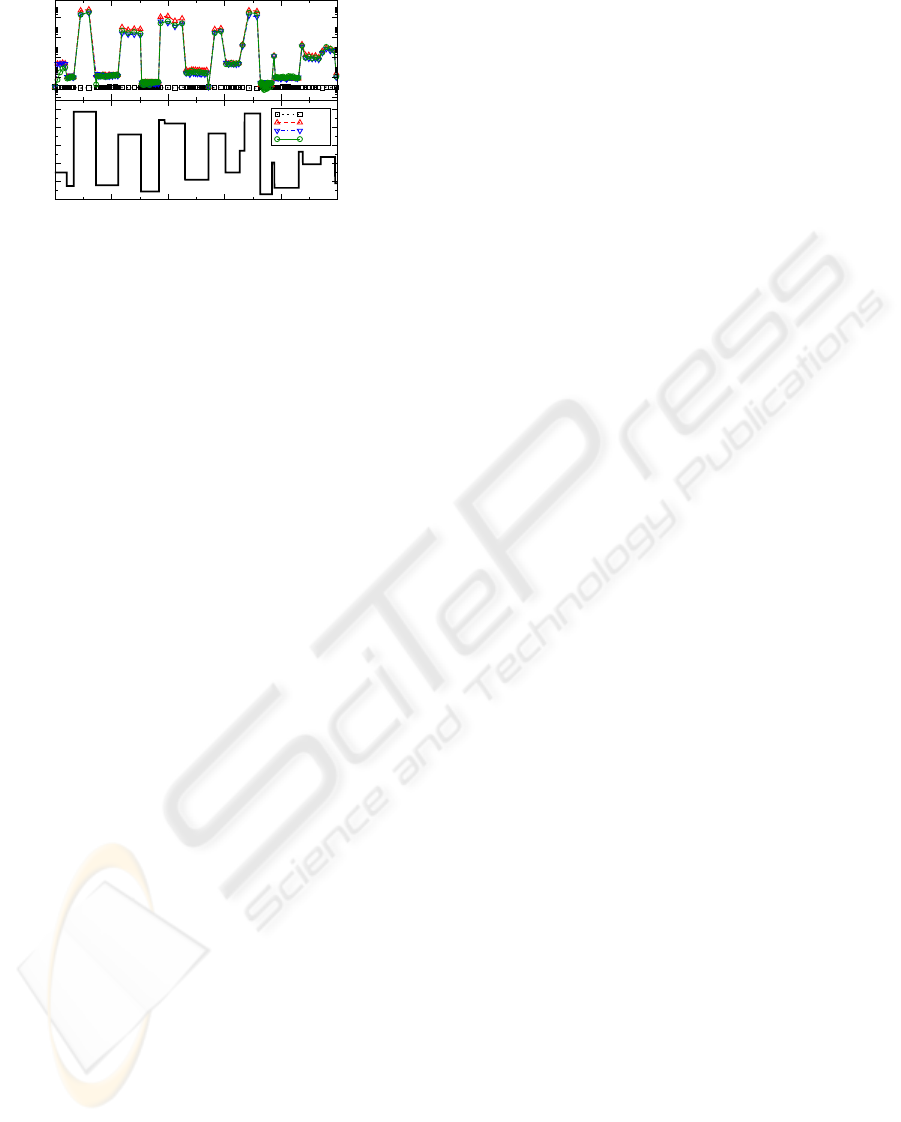
10
0
10
2
10
4
10
6
10
8
x
CP
SW
MLS
GACE
0 20000 40000 60000 80000 100000
t
0
20
40
60
80
100
T
Figure 4: (Top) Budget for different strategies and (bottom)
Periodicity of the returns, both in the course of time for RoI
with parameters T
max
= 100, t
max
= 10
4
and σ = 0.1.
investment proportionsto patterns that may be present
in the returns. We analyzed the performance of GACE
for different scenarios, and compared its performance
in the course of time against other strategies used here
as a reference. We showed that even though the strat-
egy GACE has no knowledge of the dynamics of the
returns, after a given number of time steps it may
lead to large gains, performing as well as other strate-
gies with some knowledge. This particularly is shown
for long-lasting periodicities, where an ever increas-
ing growth of budget was observed. Further work
includes the analysis of the performance of the strat-
egy GACE for real returns, and to compare the perfor-
mance of GACE against other approaches from Ma-
chine Learning.
ACKNOWLEDGEMENTS
We thank to Dr. Dagmar Monett for providing us the
program +CARPS. We also thank Frank Schweitzer
and H.-D. Burkhard for helpful advice.
REFERENCES
Dawid, H. (1999). Adaptive learning by genetic algorithms:
Analytical results and applications to economic mod-
els. Springer, Berlin.
Geibel, P. and Wysotzki, F. (2005). Risk-sensitive reinforce-
ment learning applied to control under constraints. J.
of Artif. Intell. Res., 24:81–108.
Grefenstette, J. J. (1992). Genetic algorithms for changing
environments. In Manner, R. and Manderick, B., ed-
itors, Parallel Problem Solving from Nature 2., pages
137–144. Elsevier, Amsterdam.
Harvey, I. (1992). The sage cross: The mechanics of recom-
bination for species with variable-length genotypes.
In Parallel Problem Solving from Nature, volume 2,
pages 269–278. Elsevier, Amsterdam.
Holland, J. H. (1975). Adaptation in Natural and Artificial
Systems. The University of Michigan Press.
Kahneman, D. and Tversky, A. (1979). Prospect theory of
decisions under risk. Econometrica, 47:263–291.
Kelly, J. L. (1956). A new interpretation of information rate.
AT&T Tech. J., 35:917–926.
LeBaron, B. (2001). Empirical regularities from interact-
ing long and short horizon investors in an agent-based
stock market. IEEE Transactions on Evolutionary
Computation, 5:442–455.
Magdon-Ismail, M., Nicholson, A., and Abu-Mostafa, Y.
(2001). Learning in the presence of noise. In Haykin,
S. and Kosko, B., editors, Intelligent Signal Process-
ing, pages 120–127. IEEE Press.
Michalewic¸z, Z. (1999). Genetic Algorithms + Data Struc-
tures = Evolution Programs. Springer, Berlin.
Monett, D. (2004). +CARPS: Configuration of Metaheuris-
tics Based on Cooperative Agents. In Blum, C., Roli,
A., and Sampels, M., editors, Proc. of the 1
st
Int.
Workshop on Hybrid Metaheuristics, at the 16
th
Eu-
ropean Conference on Artificial Intelligence, (ECAI
’04), pages 115–125, Valencia, Spain. IOS Press.
Navarro, J. E. and Schweitzer, F. (2003). The investors
game: A model for coalition formation. In Czaja, L.,
editor, Proc. of the Workshop on Concurrency, Speci-
fication & Programming, CS&P ’03, volume 2, pages
369–381, Czarna, Poland. Warsaw University Press.
Navarro-Barrientos, J. E., Cantero-Alvarez, R., Rodrigues,
J. F. M., and Schweitzer, F. (2008). Investments in ran-
dom environments. Physica A, 387(8-9):2035–2046.
Richmond, P. (2001). Power law distributions and dynamic
behaviour of stock markets. The European Physical
Journal B, 20(4):523–526.
Schulenburg, S. and Ross, P. (2001). Strength and money:
An LCS approach to increasing returns. In Lecture
Notes in Computer Science, volume 1996, pages 114–
137. Springer.
Turing, G. (1960). An introduction to matched filters. IEEE
T. Inform. Theory., 6(3):311–329.
Yang, S. (2005). Experimental study on population-based
incremental learning algorithms for dynamics opti-
mization problems. Soft Computing, 9(11):815–834.
CONTROLLING INVESTMENT PROPORTION IN CYCLIC CHANGING ENVIRONMENTS
213