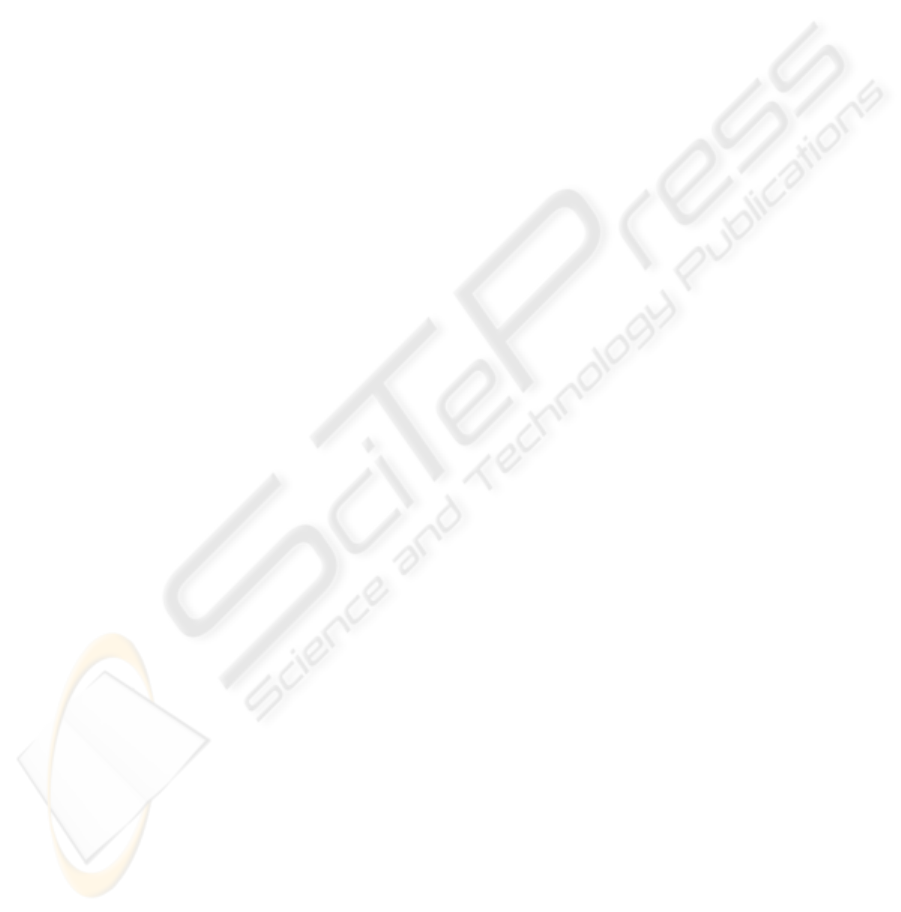
Considering the reduced order system, the Riccati
equation becomes:
F
T
P
a
+ P
F
− P
a
GR
−1
G
T
P
a
+ Q
a
= 0, (20)
so that the following control action is obtained:
u
a
(t) = −R
−1
G
T
P
a
ζ(t) = −R
−1
G
T
P
a
Cx(t)
= −K
a
x(t)
(21)
By pre- and post- multiplying eq. (20) by C
T
and C,
respectively, and considering the aggregation condi-
tions, it is straightforward to obtain:
A
T
(C
T
P
a
C) + (C
T
P
a
C)A+
− (C
T
P
a
C)BR
−1
B
T
(C
T
P
a
C) + C
T
Q
a
C = 0.
(22)
Equations (15) and (22) coincide provided that the
following positions hold:
P = C
T
P
a
C,
Q = C
T
Q
a
C.
(23)
Hence, the Q
a
matrix can be obtained as:
Q
a
= (CC
T
)
−1
CQC
T
(CC
T
)
−1
. (24)
Finally, K
a
matrix is obtained from F, G, Q
a
and R
matrices.
In this paper, a 6
th
order aggregated model is de-
rived from the full order suspension system and used
to derive the control law.
5 SIMULATION RESULTS
To evaluate the controller performances, a set of tests
is performed on the virtual prototype by applying
different road profiles. In particular, the following
benchmarks used in industrial practice are considered
(Canale et al., 2006):
•
Sine wave hole
profile: a sine profile hole with
0.03 m of amplitude and 6 m of width; for the
sake of brevity, only 30 and 90 Km/h car speeds
are considered in this paper;
•
Short back
profile: a positive step of road profile
with 0.02 m of amplitude and 0.5 m of width, with
a travelling speed of 30 Km/h;
•
Drain well
profile: a negative step variation of
road profile with 0.05 m of amplitude and 0.6 m
of width, with a traveling speed of 30 km/h.
Moreover, the system response in case of entry in
a curve or braking is analysed. The proposed con-
troller performances have been compared with those
obtained by using an active decoupled controller (Ike-
naga et al., 2000) and an LQR controller based on the
full order model. In particular, the control scheme
proposed in (Ikenaga et al., 2000) includes a ride con-
trol loop, for road disturbances rejection, and an atti-
tude control loop, for roll, pitch and vertical dynam-
ics regulation. To former includes an active filtering
feedback, the latter is based on a sky-hook control
strategy. A decoupling is performed to deal with the
under-actuation problem.
Figure 4 shows pitch and vertical displacement
dynamics when applying the sine wave hole road pro-
file. Since the road disturbance is symmetrically ap-
plied to left and right wheels, the roll dynamics is neg-
ligible and not displayed.
It is evident that the LQR controller guarantees
better performances than the near-optimum and the
decoupled controllers, in terms of overshoot and set-
tling time. Nevertheless, the near optimum controller
allows acceptable pitch angle and vertical displace-
ment dynamics, improving the results obtained with
the decoupled controller.
In Figure 5, the drain well and the short back
disturbances are only applied to left wheels, so that
the roll angle dynamics is excited. Simulation results
show that the LQR controller still guarantees a better
system behaviour for all conditions thanks to a prompt
control action, while the near-optimum controller im-
proves results obtained with the decoupled controller.
Finally, Figure 6 displays the effect of sudden
longitudinal and lateral accelerations determined by
braking (Fig. 6(a)), and entry into a curve (Fig. 6(b)),
which independently affect the pitch dynamics and
the roll dynamics, respectively.
In the former case, the near-optimum and the LQR
controllers show similar performances; in the latter
case, the near-optimum controller cannot reduce sig-
nificantly the roll angle overshoot. In general, the
decoupled controller cannot guarantee fast transients
due to actuator saturation; the near optimum and
LQR controller can suitably restrain the control ac-
tion thanks to a suitable choice of the performance in-
dex weights. To sum up, the near optimum controller
represents a compromise in terms of system perfor-
mances and complexity, while the LQR controller al-
ways shows the best performances.
6 CONCLUSIONS
In this paper, a near-optimum control strategy applied
to a full-car active suspension system has been pro-
posed. The design process relies on the use of a high
order analytical model, from which an aggregated low
order model is derived, and of a virtual prototype de-
veloped by using the AMESim simulation package.
ICINCO 2008 - International Conference on Informatics in Control, Automation and Robotics
50