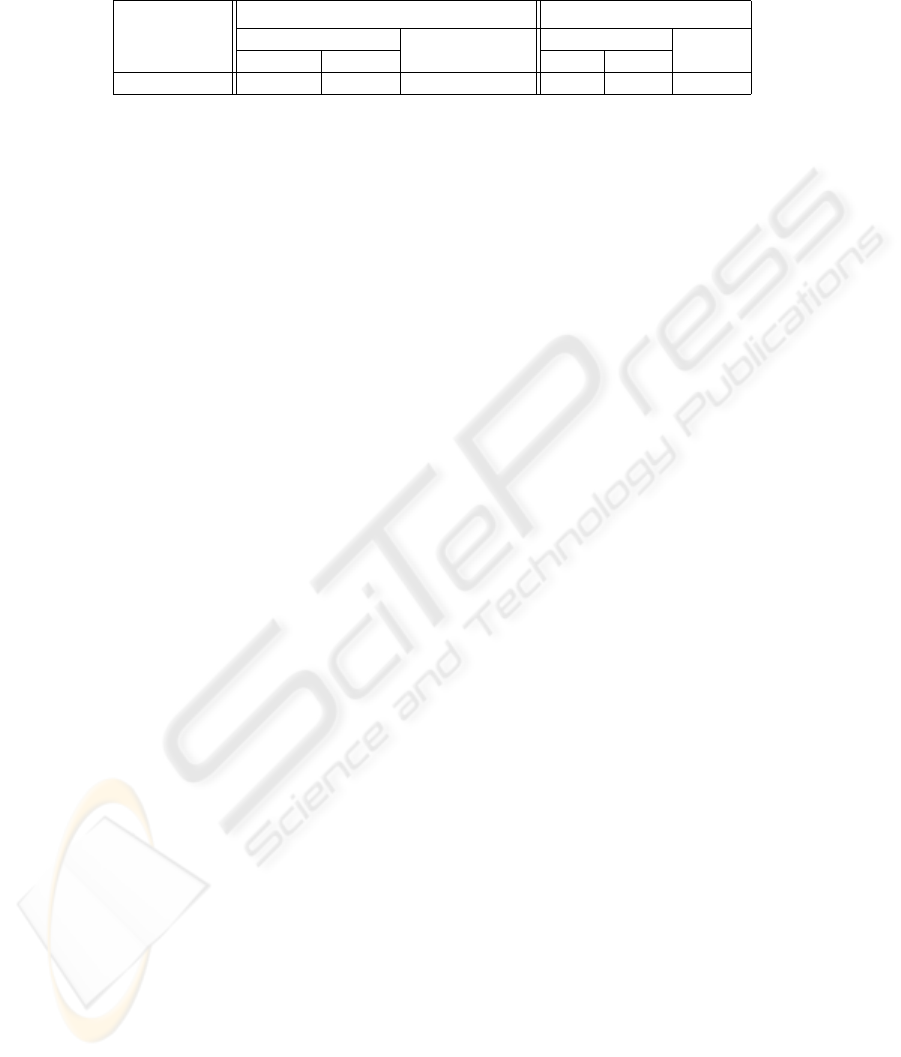
Table 5. The results of classifying the different validation and test sets. We also give the standard
deviation for validation sets.
Model
Validation Sets The independent test set
mean errors errors
easy set hard set
mean accuracy
easy set hard set
accuracy
2 gmms ‘diag’ 21 ± 4 48 ± 5 98.675 ± 0.126 49 119 98.717
6 Discussion
The fast decoding of a stream of data represented as pulses of light is a commercially
important and challenging problem. Computationally the challenge is in the speed of the
classifier and the need for simple processing. We have therefore restricted our classifier
to be, for the most part, an SLN and the data is either a sampled version of the light
waveform or just the energy of the pulse. Experiment 1 showed that an SLN trained
with the 96-ary representation of the waveform gave the best performance, reducing the
bit error rate from 2.8% to 1.23%. This figure is still quite high and we hypothesised
that the explanation was the fact that despite the data set being very large, 65532 items,
the number of difficult examples (those misclassified by the threshold method) was
very small and dominated by the number of straightforward examples. To see if we
could correctly identify a significant number of these infrequent but difficult examples
we undertook experiments 2 and 3 .
Although there was a small improvement obtained by using energy threshold band,
further work is needed to determine if there is an optimal band size. Similar results were
obtained by experiment 3.
This is early work and much of interest is still to be investigated, such as repre-
sentational issues of the waveform, threshold band sizes, and other methods to identify
difficult cases.
References
1. Bulow, H. (2002) Electronic equalization of transmission impairments, in. OFC, Anaheim,
CA, Paper TuE4.
2. Haunstein, H.F. & Urbansky, R. (2004) Application of Electronic Equalization and Error
Correction in Lightwave Systems. In Proceedings of the 30th European Conference on Op-
tical Communications (ECOC), Stockholm, Sweden.
3. Rosenkranz, W. & Xia, C. (2007) Electrical equalization for advanced optical communica-
tion systems. AEU - International Journal of Electronics and Communications 61(3):153-
157.
4. Watts, P.M., Mikhailov, V., Savory, S. Bayvel, P., Glick, M., Lobel, M., Christensen, B.,
Kirkpatrick, P., Shang, S., & Killey, R.I. (2005) Performance of single-mode fiber links using
electronic feed-forward and decision feedback equalizers. IEEE Photon. Technol. Lett 17
(10):2206 - 2208.
5. Bishop, C.M. (1995) Neural Networks for Pattern Recognition. New York: Oxford University
Press.
6. Dempster, A.P., Laird, N.M, & Rubin, D.B. (1977) Maximum likelihood from incomplete
data via the EM algorithm. J. Roy. Stat. Soc. B, 39 :1–38.
79