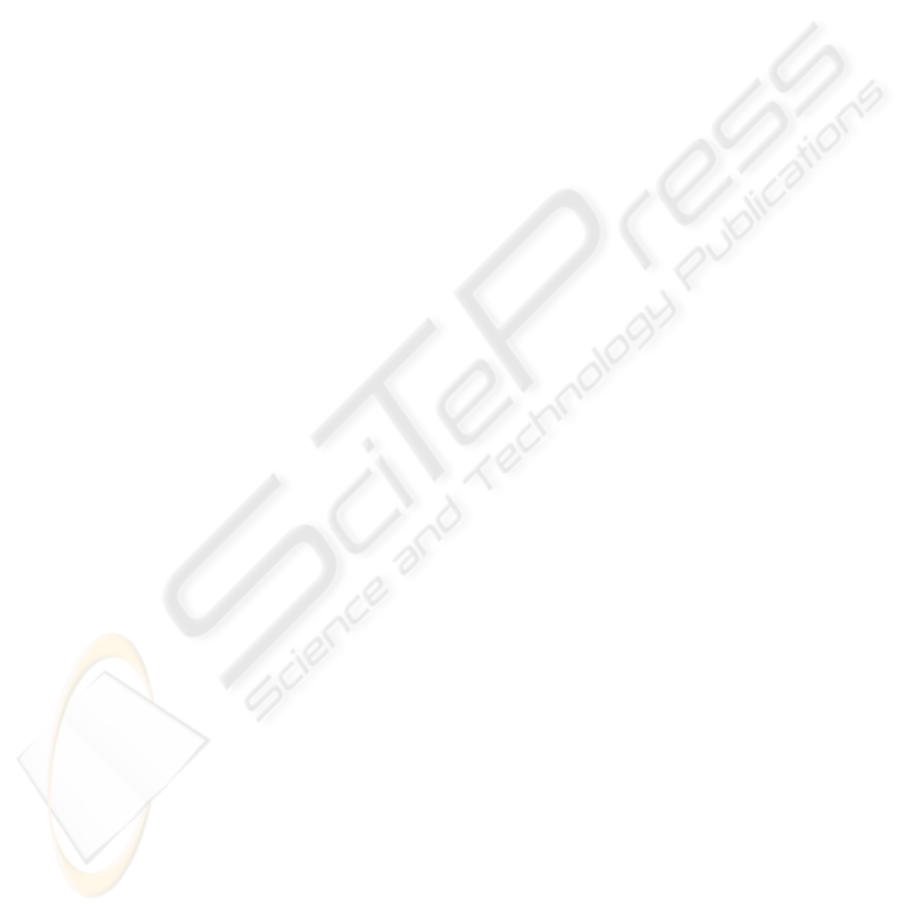
the last decade the mathematical complexity and computational intensity of physical
model-based OBD has stimulated research on alternative fault detection and diagnosis
approaches suitable for automotive engines (Nyberg, 1999; Grimaldi et al., 2001;
Crossman et al., 2003; Kimmich et al., 2005.)
Some work has also been done on statistical methods, including principal
component analysis (PCA) where a reduced set of statistically independent score
variables are generated for process monitoring. Unfortunately, while PCA in its
original form is only applicable to linear data, a nonlinear extension in the form of an
auto-associative neural network (AANN) (Kramer, 1992) is now available, where the
scores are produced in the network bottleneck layer. Because of its conceptual
simplicity and close relation to linear PCA, our previous work on diesel engines used
an AANN for monitoring during steady-state operation (Antory et al., 2005),
including increased sensitivity in detecting minor faults (Wang et al., 2008a) by
incorporating additional analysis in the form of the statistical Local Approach.
Nonlinear PCA (NLPCA) has also proved to be effective when applied to
experimental data recorded from the air intake system of a gasoline engine during
transient operation (Wang et al., 2008b). Here the AANN was trained on a modified
identification (MI) cycle, specially designed to ensure that the engine speed and
throttle position covered the complete operating map at rates similar to those
experienced during normal operation. Importantly, the resulting model proved
suitable for more general use with the New European Drive Cycle (NEDC), a
standardised test for all new model types representing a mixture of urban and
motorway driving. In this work also, rather than using the same operational cycle for
AANN training and testing, two completely different engine drive cycles will be
employed, as detailed later.
The major limitation in all the previous work is the absence of any consideration of
the effects of atmospheric changes on the ability of the model-based fault detection to
generalise in terms of avoiding false alarms while correctly identifying fault
conditions. Thus, the experimental data used for validating the AANN model and the
faulty data sets were all recorded under similar atmospheric temperature and pressure
conditions to those for the training data. Moreover, none of the measured engine
variables previously included in the monitoring model was in fact significantly
affected by such atmospheric changes. Any AANN model derived for IC engine
monitoring under laboratory conditions, should also be capable of being generalised
to a wider range of driving conditions. The aim of the present paper is to address this
important deficiency. The practical significance of the results reported later is that the
experimental data sets available for neural modelling not only covered two different
operational cycles, as required for dynamic monitoring, but were also recorded under
different temperature conditions.
Some work on the effect of changes in atmospheric pressure and temperature on
the performance of an engine has been reported in the literature, but from the
perspective of engine design (Sher, 1984). Here computer code was developed to
simulate the engine cycle for the purpose of evaluating an optimal engine design
giving the best performance at high altitude conditions. The focus of this paper is data
modelling of a modern automotive petrol engine, based on which its fault monitoring
under different driving conditions can be achieved. This further significantly extends
29