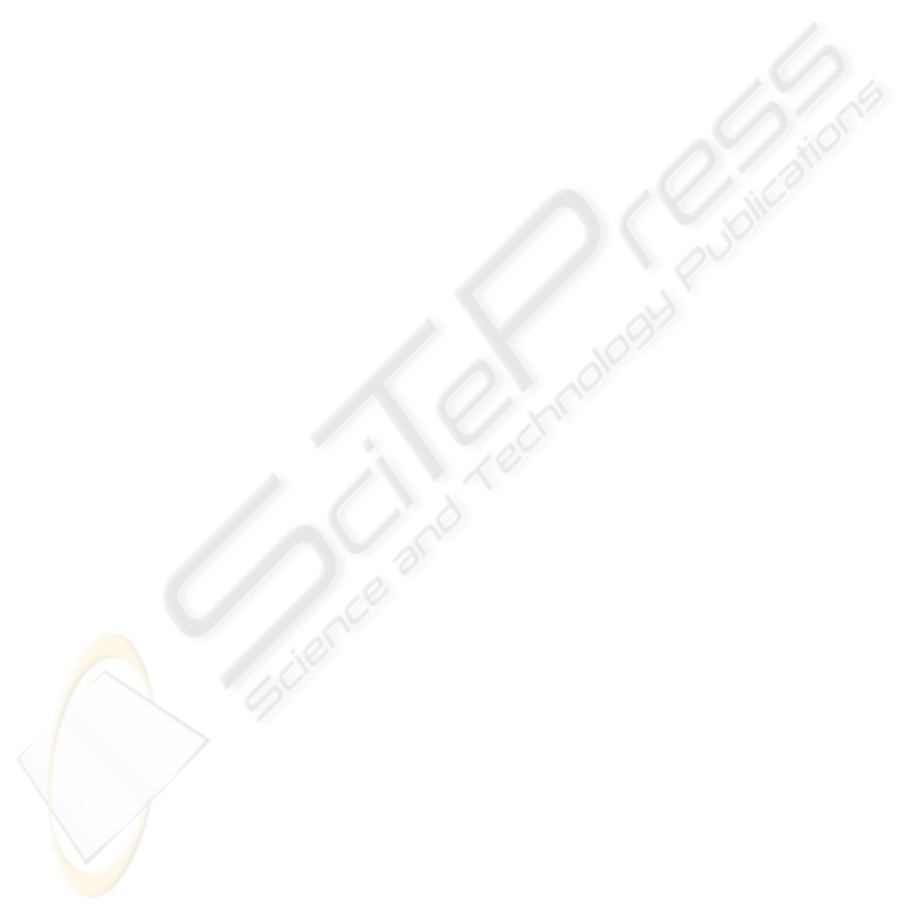
training data which is a general operation condition (requirement) for all artificial
learning based techniques.
The main future perspective of this work is related to T-DTS automatic optimization
of and to the extension of database decomposition methods.
References
1. Bouyoucef E., Chebira A., Rybnik M., Madani K.: Multiple Neural Network Model Gen-
erator with Complexity Estimation and Self-Organization Abilities: International Scientific
Journal of Computing (2005) Vol. 4. Issue 3. 20–29.
2. Rybnik M.: Contribution to the Modeling and the Exploitation of Hybrid Multiple Neural
Networks Systems: Application to Intelligent Processing of Information, PhD Thesis, Uni-
versity Paris XII, LISSI (2004)
3. Bouyoucef E.: Contribution à l’étude et la mise en œuvre d’indicateurs quantitatifs et
qualitatifs d’estimation de la complexité pour la régulation du processus d’auto
organisation d’une structure neuronale modulaire de traitement d’information, PhD Thesis
(in French), University Paris XII, LISSI (2006)
4. Budnyk I., Chebira A., Madani K.: Estimating Complexity of Classification Tasks Using
Neurocomputers Technology: International Scientific Journal of Computing (2007)
5. Madani M., Rybnik M., Chebira A.: Data Driven Multiple Neural Network Models Genera-
tor Based on a Tree-like Scheduler: Lecture Notes in Computer Science edited Mira. J.,
Prieto A.: Springer Verlag (2003). ISBN 3-540-40210-1, 382-389.
6. Mehmet I. S., Bingul Y., Okan K. E., Classification of Satellite Images by Using Self-
organizing Map and Linear Support Vector Machine Decision Tree, 2nd Annual Asian
Conference and Exhibition in the field of GIS, (2003).
7. Chi H., Ersoy O.K., Support Vector Machine Decision Trees with Rare Event Detection,
International Journal for Smart Engineering System Design (2002), Vol. 4, 225-242.
8. Fukunaga K.: Introduction to statistical pattern recognition, School of Electrical Engineer-
ing, Purdue University, Lafayette, Indiana, Academic Press, New York and London, (1972).
9. Edmonds B., What is Complexity? - The philosophy of complexity per se with application
to some examples in evolution: Heylighen F. & Aerts D. (Eds. 1999): The Evolution of
Complexity, Kluwer, Dordrecht.
10. Feldman D.P., Crutchfield J.P.: Measure of statistical complexity: Why? Phys. Lett.(1998)
A 238: 244-252.
11. Budnyk I., Chebira A., Madani K.: ZISC Neural Network Base Indicator for Classification
Complexity Estimation, ANNIIP (2008), 38-47.
12. Madani K., Chebira A.: Data Analysis Approach Based on a Neural Networks Data Sets
Decomposition and it’s Hardware Implementation, PKDD, Lyon, France (2000).
13. Dasarathy B.V. editor (1991) Nearest Neighbor (NN) Norms: NN Pattern Classification
Techniques. ISBN 0-8186-8930-7
14. Park. J., Sandberg J. W.: Universal Approximation Using Radial Basis Functions Network,
Neural Computation (1991) Volume 3, 246-257.
15. Aha. D. W., Incremental Constructive Induction: An Instance-Based Approach. In Proceed-
ings of the Eight International Workshop on Machine Learning. Morgan Kaufmann (1991),
117-121.
27