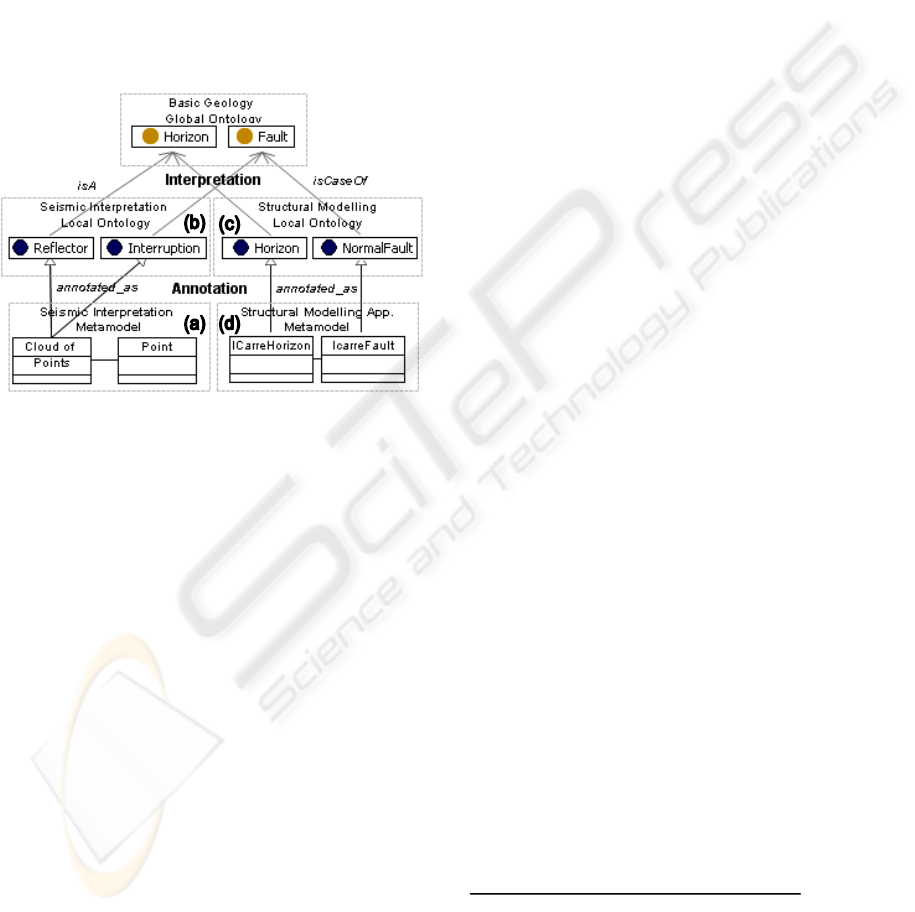
to be parts of one same structural horizon. And
he/she can specify the structural category of the fault
(normal, reverse), by observing the way it affects the
structure. This illustrates the subsumption link
between the concepts of the GO and the concepts of
Structural Geology LO: NormalFault is_a Fault
(
Figure 6 (c)).
Finally, when the user stores the structural
model, the objects are saved in binary files that
represent a specific structural interpretation
metamodel (such as ICarre metamodel
3
), whose
entities are annotated with the concepts of the
Structural modelling LO. (Figure 6 (d).
Figure 6: Links between GO, LO and Metamodels.
Setting up this approach, we are able to answer
to queries that refer to different domains. Such a
query would be, for example, by which seismic
reflectors is formed the structural horizon H1? An
structural horizon is represented as an
ICarreHorizon (d), which is annotated as
StructuralHorizon (c), which is subsumed by the
concept Horizon in the GO. All those instances have
the same ID. It is then easy to retrieve the instances
of Reflector in the Seismic LO that are subsumed by
the GO Horizon and that are used to annotate the
looked-for “cloud of points” files.
5 CONCLUSIONS
We have presented here an approach based on
ontologies to annotate specific domain conceptual
models. The application domain is the workflow of
oil reservoir modelling, which is a multi-
representation multi-interpretation domain. In this
process, an interpretation can be considered as
putting in correspondence concepts belonging to
different specialized domains. So, our architecture
proposes to create semantic annotations from the
specific metamodels to the local ontologies. Then,
the local ontologies concepts are subsumed by the
global ontology concepts, which is the pivot of the
modelling process.
Creating correspondences between the models is
likely to enable us to answer queries that cannot be
addressed at present, because we cannot recover the
relation between objects identified in different
phases of the process.
The next steps in this work will be to automate
most complex mapping rules, which will represent
inferences that can be made within specific domains.
Moreover, we expect to scale up the proposed
approach to deal with large file size interpretations.
We plan to use persistent models with ontology
based databases.
REFERENCES
Lin, Y., Strasunskas, D., Hakkarainen, S., Krogstie, J., &
Solvberg, A. (2006). Semantic Annotation Framework
to Manage Semantic Heterogeneity of Process
Models. In S. B. Heidelberg (Ed.), Advanced
Information Systems Engineering (Vol. 4001/2006,
pp. 433-446): Springer.
Mastella, L., Perrin, M., Abel, M., Rainaud, J.-F., &
Touari, W. (2007). Knowledge Management for
Shared Earth Modelling. Paper presented at the EAGE
Conference & Exhibition, London.
Noy, N. F. (2004, December 2004). Semantic Integration:
A Survey Of Ontology-Based Approaches. SIGMOD
Record, Special Issue on Semantic Integration, 33.
Perrin, M., Zhu, B., Rainaud, J.-F., & Schneider, S.
(2005). Knowledge-driven applications for geological
modeling. Journal of Petroleum Science and
Engineering, 47(1-2), 89-104.
Uschold, M., & Gruninger, M. (2005). Architectures for
Semantic Integration. Paper presented at the Dagstuhl
Seminar Proceedings: Semantic Interoperability and
Integration, Dagstuhl, Germany.
1
. UML-like class diagram from TopBraid Composer tool
(http://topbraidcomposer.com).
2
. UML class diagram.
3
. OpenFlow ICarre proprietary modelling application of French
Institute of Petroleum (http://www.ifp.fr).
ONTOLOGY-BASED MODEL ANNOTATION OF HETEROGENEOUS GEOLOGICAL REPRESENTATIONS
293