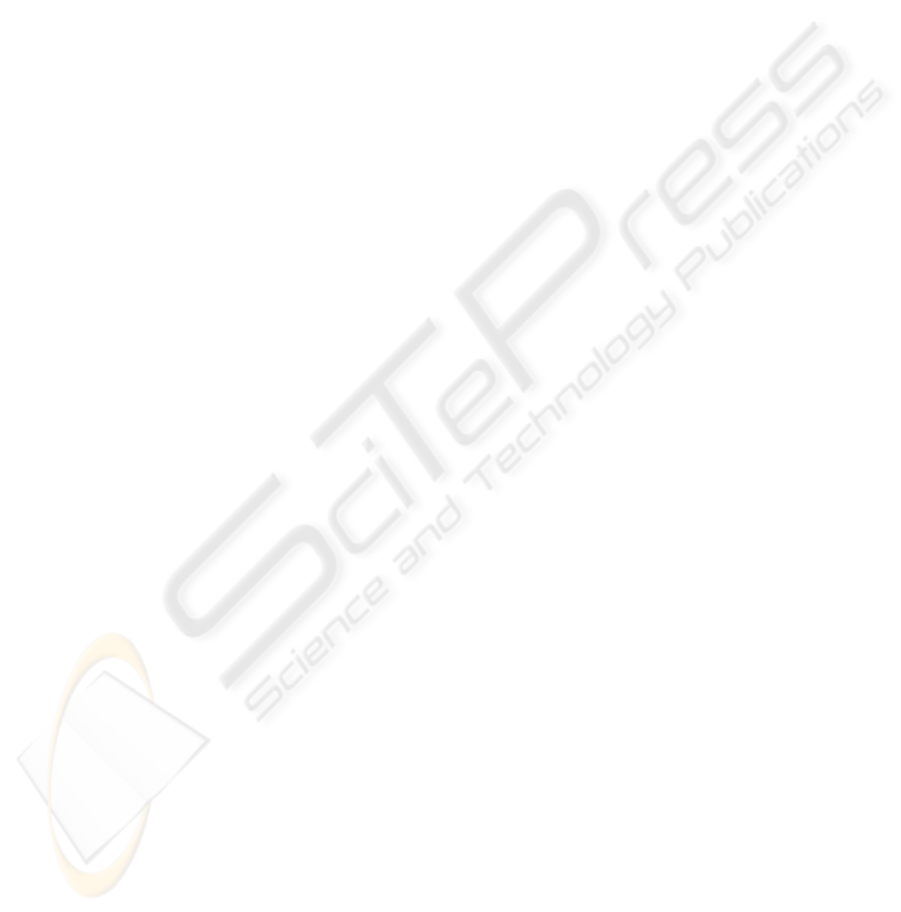
capability on the same level as legitimate
organizations. As discussed in the introduction, only
data from a single target in the financial services
area was used to develop the investigation
methodology. However, anecdotal evidence suggests
that most banks and financial institutions are
experiencing qualitatively similar attacks. Our first
task in generalizing our findings will be to replicate
the results across data sets from other institutions. Of
course, practical difficulties exist in obtaining this
data from organizations that keep their operational
security issues secret.
A second major challenge is to validate the
findings across further time periods, and get a sense
of the variation in both group composition and
features used. One can anticipate a high-level of
turnover in the features used, however, if they are
not revealed in the public arena and/or incorporated
into anti-spam signature databases, then our
experience is that the values are not altered.
We are also investigating methods that enable
automated profiling of phishing attacks by groups in
real time and be built in to commercial tools for law
enforcement based on classification techniques from
natural language processing (Watters,2002). We
intend to extend the approach by utilizing
hierarchical clustering to identify more complex
patterns of heredity among the different techniques
being used by each group.
ACKNOWLEDGEMENTS
This work was funded by a major Australian
financial institution that wishes to remain
anonymous for operational security reasons.
REFERENCES
Alleged Phishing and Organized Crime Group Arrests.
Technology News Daily 2006.
Card fraud losses continue to fall 14 March 2007 (on-line)
http://www.apacs.org.uk/media_centre/press/07_14_0
3fraud.html
Abad, C., The Economy of Phishing: A Survey of the
Operations of the Phishing Market, 2005.
Chandrasekaran, M., Narayanan, K., and Upadhyaya, S.
Phishing E-mail Detection Based on Structural
Properties. In Proceedings of the NYS Cyber Security
Conference. 2006
[de-Vel, O. Mining E-mail Authorship In Proceedings of
the Workshop on Text Mining, ACM International
Conference on Knowledge Discovery and Data
Mining (KDD'2000). 2000
de-Vel, O., Anderson, A., Corney, M., et al., Mining E-
mail Content for Author Identification Forensics.
SIGMOD: Special Section on Data Mining for
Intrusion Dection and Threat ANalysis, 2001
Dhamija, R., Tygar, J.D., and Hearst, M. Why Phishing
Works. In Proceedings of the CHI 2006. Montréal,
Québec, Canada, 2006
Fette, I., Sadeh, N., and Tomasic, A. Learning to Detect
Phishing E-mails. In Proceedings of the 16th
international conference on World Wide Web (WWW
2007).p.649 - 656:ACM Press, 2007
Jagatic, T., Johnson, N., Jakobsson, M., et al., Social
Phishing, School of Informatics Indiana University, 12
December, 2005
Jakobsson, M., Modeling and Preventing Phishing
Attacks, School of Informatics Indiana University at
Bloomington, 27 October, 2005
James, L., Phishing Exposed. Rockland MA: Syngress
Publishing, 2005
McMillan, R. 'Rock Phish' blamed for surge in phishing,
(on-line) http://www.infoworld.com
/article/06/12/12/HNrockphish_1.html
Naraine, R. Return of the Web Mob, April 10, 2006 (on-
line)
http://www.eweek.com/article2/0,1895,1947561,00.as
p
Ramzan, Z. and W¨uest, C. Phishing Attacks: Analyzing
Trends in 2006. In Proceedings of the Fourth
Conference on E-mail and Anti-Spam (CEAS 2007).
2007
Stamp, P., Penn, J., Adrian, M., et al., Increasing
Organized Crime Involvement Means More Targeted
Attacks, Forrester Research, October 12, 2005
Watters, P.A., Discriminating English word senses using
cluster analysis. Journal of Quantitative Linguistics.
9(1): 77-86,2002
FORENSIC CHARACTERISTICS OF PHISHING - Petty Theft or Organized Crime?
157