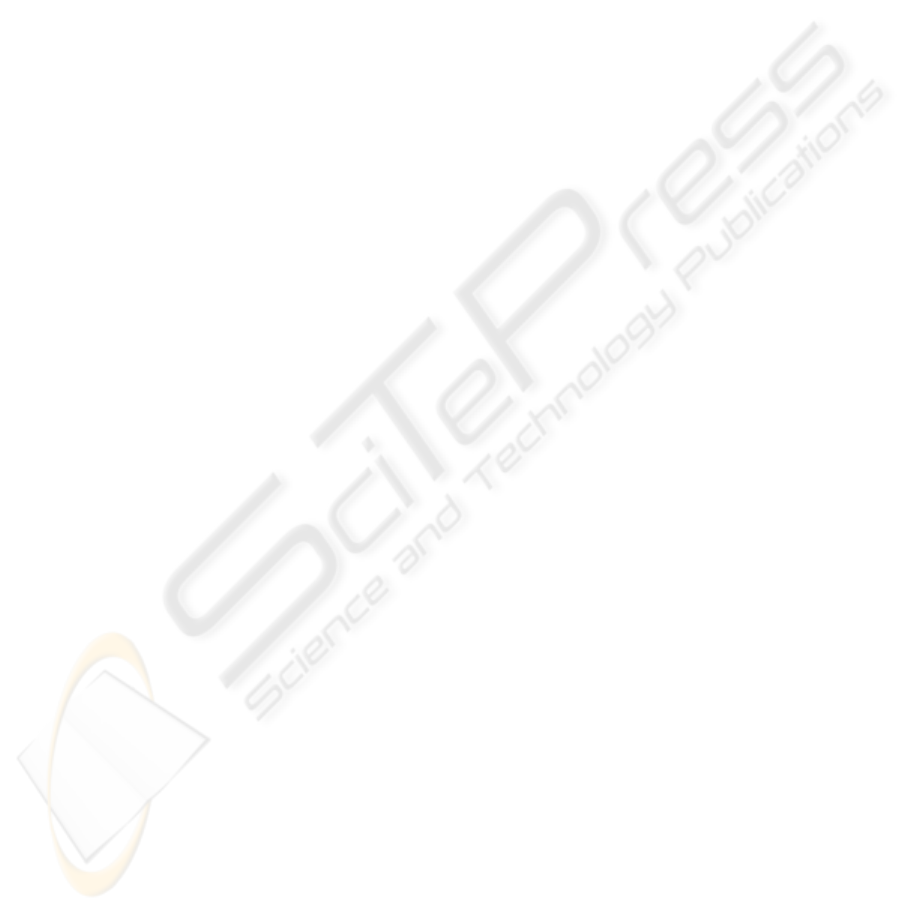
duced, among them the average page view duration
and the number of news categories visited by the par-
ticipants. For each attribute at least two pairs of user
tasks showed a significant difference. Using these at-
tributes, it is possible to differentiate between all three
tasks.
The next step will be to examine further behav-
ioral aspects, e.g. the scrolling. A question of par-
ticular importance will be whether the attributes nec-
essary to recognize a user task are general or can be
applied to only one kind of Web site. In the worst
case this would mean that a task recognition strategy
has to be developed for each type of Web site sepa-
rately with specialized attributes added to the general
attributes.
The findings of this study will be used for the
formulation of hypotheses describing the relationship
between user task and behavior. A further study in a
more natural surrounding with more participants and
thus more data will be conducted to prove these hy-
potheses. On the basis of the outcomes of this next
study, methods of automatic user task recognition can
be developed which will lead to a new quality of
user support. Knowing the user’s task means that the
user’s current needs are revealed. According to the
task, different services could be offered. Fact Find-
ers would certainly welcome a search functionality,
whereas forums concerning the topic which the users
are interested in can be offered when performing In-
formation Gathering. In the case of Just Browsing,
entertainment and distraction play an important role,
thus, these users might be interested in pictures and
videos. Moreover, the method with which link recom-
mendations are found could be altered; in the case of
Fact Finding, text mining is applicable whereas meth-
ods like the association rule mining can be used for
the other two tasks. However, Information Gathering
requires that the recommendations refer to the topic
which is currently of interest. These are only a few
of the manifold applications of user task recognition
which makes clear how important further investiga-
tions on this topic are.
ACKNOWLEDGEMENTS
We would like to thank Friedemann W. Nerdinger and
Stefan Melchior from the chair of Economic Psychol-
ogy at the University of Rostock for their support in
the study design. We also want to thank the peo-
ple who took part in the experiment. This work was
funded by the DFG (Graduate School 466).
REFERENCES
Broder, A. (2002). A taxonomy of web search. SIGIR Fo-
rum, 36(2):3–10.
Byrne, M. D., John, B. E., Wehrle, N. S., and Crow, D. C.
(1999). The tangled web we wove: a taskonomy of
www use. In CHI ’99: Proceedings of the SIGCHI
conference on Human factors in computing systems,
pages 544–551, New York, NY, USA. ACM Press.
Chen, M. C., Anderson, J. R., and Sohn, M. H. (2001).
What can a mouse cursor tell us more?: correlation
of eye/mouse movements on web browsing. In CHI
’01: CHI ’01 extended abstracts on Human factors in
computing systems, pages 281–282, New York, NY,
USA. ACM Press.
Choo, C. W., Detlor, B., and Turnbull, D. (2000). Infor-
mation seeking on the web: An integrated model of
browsing and searching. First Monday, 5(2).
Das, A., Datar, M., and Garg, A. (2007). Google news per-
sonalization: Scalable online collaborative filtering.
In Proceedings of the 16th international conference
on World Wide Web, pages 271–280. ACM Press.
G
´
ery, M. and Haddad, H. (2003). Evaluation of web usage
mining approaches for user’s next request prediction.
In WIDM ’03: Proceedings of the 5th ACM interna-
tional workshop on Web information and data man-
agement, pages 74–81, New York, NY, USA. ACM
Press.
Kellar, M. and Watters, C. (2006). Using web browser inter-
actions to predict task. In WWW ’06: Proceedings of
the 15th international conference on World Wide Web,
pages 843–844, New York, NY, USA. ACM Press.
Kellar, M., Watters, C., and Shepherd (2006). The impact
of task on the usage of web browser navigation mech-
anisms. In Proceedings of Graphics Interface (GI
2006), pages 235–242, Quebec City, Canada. Cana-
dian Information Processing Society.
Lee, U., Liu, Z., and Cho, J. (2005). Automatic identifi-
cation of user goals in web search. In WWW ’05:
Proceedings of the 14th international conference on
World Wide Web, pages 391–400, New York, NY,
USA. ACM Press.
Linden, G., Smith, B., and York, J. (2003). Amazon.com
recommendations - item-to-item collaborative filter-
ing. Internet Computing, IEEE, 7(1):76–80.
Morrison, J. B., Pirolli, P., and Card, S. K. (2001). A taxo-
nomic analysis of what world wide web activities sig-
nificantly impact people’s decisions and actions. In
CHI ’01: CHI ’01 extended abstracts on Human fac-
tors in computing systems, pages 163–164, New York,
NY, USA. ACM Press.
Rose, D. E. and Levinson, D. (2004). Understanding user
goals in web search. In WWW ’04: Proceedings of
the 13th international conference on World Wide Web,
pages 13–19, New York, NY, USA. ACM Press.
Sellen, A. J., Murphy, R., and Shaw, K. L. (2002). How
knowledge workers use the web. In CHI ’02: Pro-
ceedings of the SIGCHI conference on Human factors
in computing systems, pages 227–234, New York, NY,
USA. ACM Press.
WEBIST 2008 - International Conference on Web Information Systems and Technologies
222