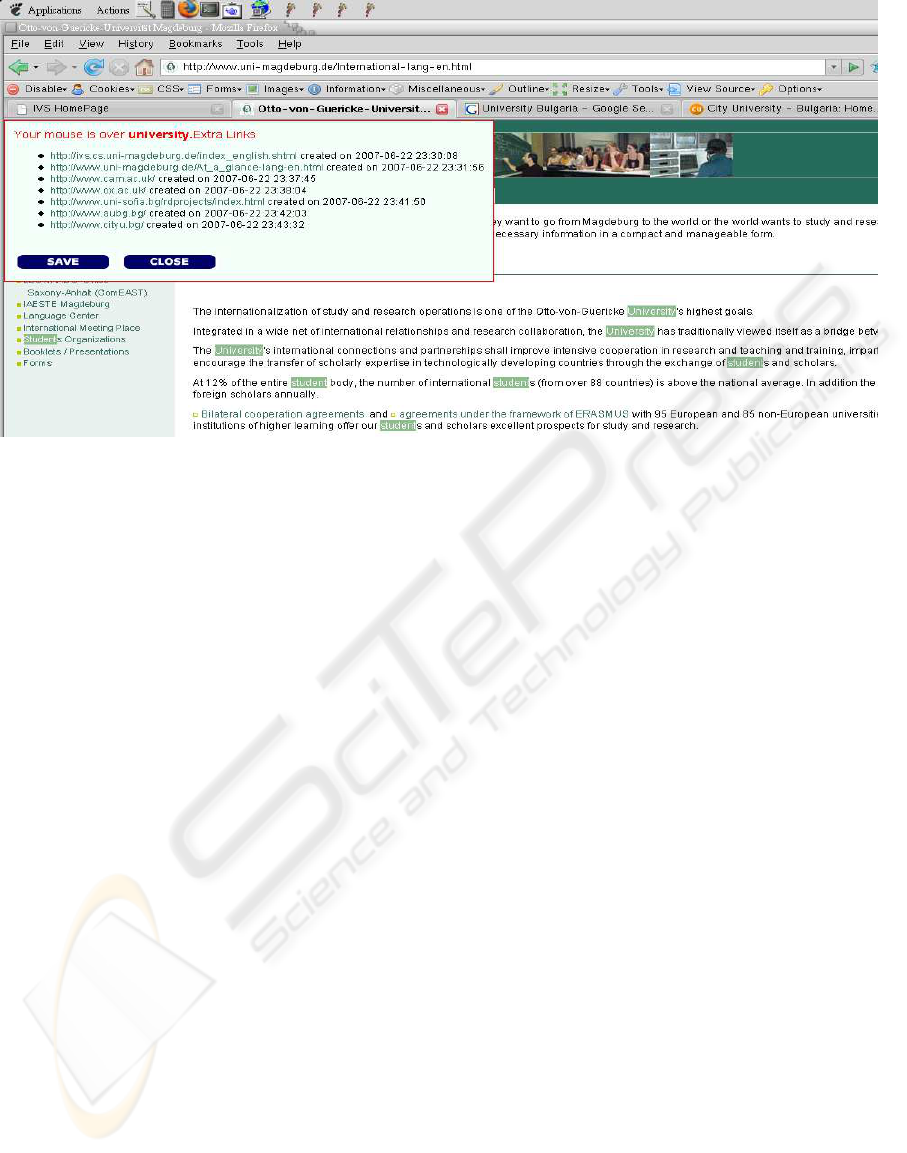
Figure 2: Screenshot of an enriched Web page.
which the Semantic window is created. For the sake
of simplicity datatype properties are not taken into
consideration. The cost function f
cost
is given in ta-
ble 1 and the maximum cost is A = 3. Filled circles
represent concepts, filled squares represent instances
and filled diamonds on arrows represent object prop-
erties, all being located within the range of the Se-
mantic Window around C
6
.
Based on the developed architecture, a prototype
was implemented. To proof the applicability of the
proposed approach a web-based example was chosen
for the enrichment of web pages using semantic infor-
mation from an ontology (cp. figure 2).
3 CONCLUSIONS AND FURTHER
WORK
In this paper the authors presented an algorithm for
the ontology-based content enrichment for the do-
main of e-learning. Other areas of application are the
enrichment of courses, assessments, interaction tools
as well as tools for the creation and management of
content and more complex learning units.
Another key aspect of this paper is the presenta-
tion of the Semantic Window idea. It support the se-
lection of semantically-related enrichment resources.
Based on a given cost function and a maximum cost,
the size of the Semantic Window can be determined.
The integration of ontology adaptation mecha-
nisms as well as a central ontology repository for
a community-based usage are possible future exten-
sions. Another focus will be the refinement and im-
provement of the enrichment algorithm.
REFERENCES
Adomavicius, G. and Tuzhilin, A. (2005). Toward the Next
Generation of Recommender Systems: A Survey of
the State-of-the-Art and Possible Extensions. IEEE
Transactions on Knowledge and Data Engineering,
17(6):734–749.
Alani, H. and Brewster, C. (2005). Ontology Ranking Based
on the Analysis of Concept Structures. In K-CAP
’05: Proceedings of the 3rd International Conference
on Knowledge Capture, pages 51–58, New York, NY,
USA. ACM Press.
Drachsler, H., Hummel, H., van den Berg, B., Eshuis,
J., Berlanga, A., Nadolski, R., Waterink, W., Boers,
N., and Koper, R. (2007). Recommendation Strate-
gies for e-Learning: Preliminary Effects of a Personal
Recommender System for Lifelong Learners. URL:
http://hdl.handle.net/1820/1010.
Juneidi, S. J. and Vouros, G. A. (2005). Engineering an E-
learning Application using the ARL Theory for Agent
Oriented Software Engineering. In 2005 AAAI Fall
Symposium, Arlington, Virginia, USA. MIT press.
Princeton University (2006). WORDNET - A Lexi-
cal Database for the English Language. URL:
http://wordnet.princeton.edu/.
Tartir, S., Arpinar, I. B., Moore, M., Sheth, A. P., and
Aleman-Meza, B. (2005). OntoQA: Metric-Based
Ontology Quality Analysis. In Proceedings of IEEE
ICDM 2005 Workshop on Knowledge Acquisition
from Distributed, Autonomous, Semantically Hetero-
geneous Data and Knowledge Sources.
PROACTIVE AUTONOMOUS RESOURCE ENRICHMENT FOR E-LEARNING
467