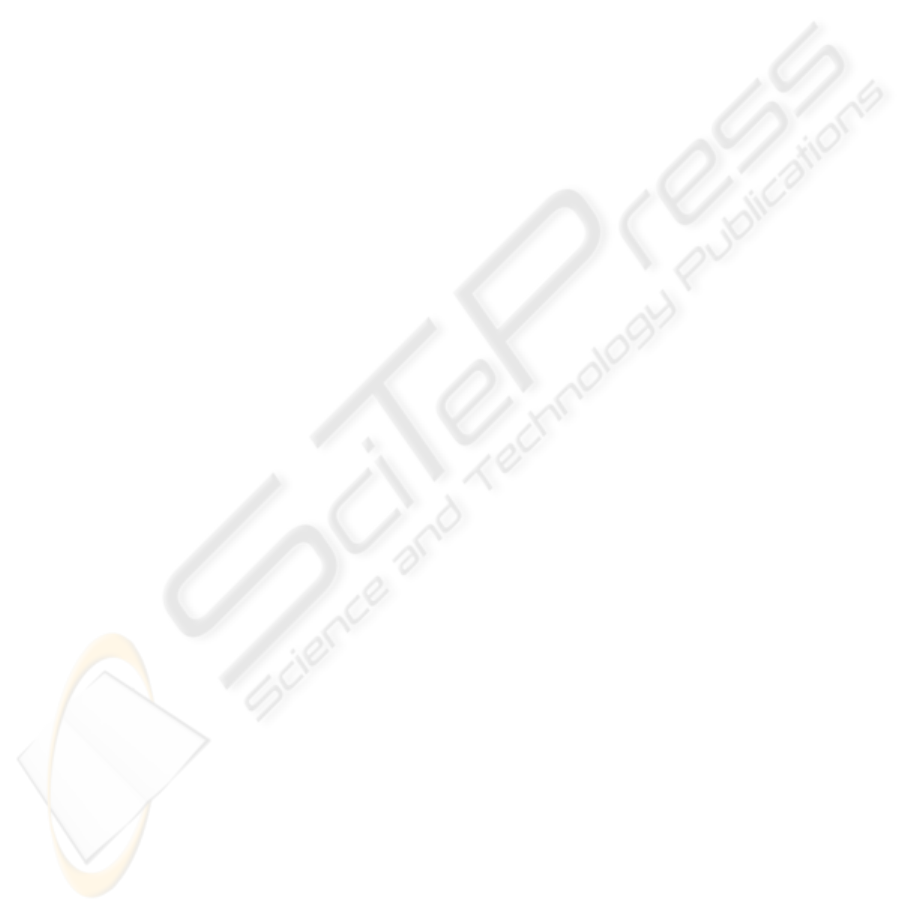
http://www.webanalyticsassociation.org/. Web Ana-
lytics Association.
Burton, M. C. and Walther, J. B. (2001). The value of web
log data in use-based design and testing. Computer-
Mediated Communication
, 6(3).
Chi, Y., Yang, Y., and Muntz, R. R. (2004). Hybridtreem-
iner: An efficient algorithm for mining frequent
rooted trees and free trees using canonical forms. The
16th International Conference on Scientific and Sta-
tistical Database Management (SSDBM ’04), 00:11.
Cooley, R., Mobasher, B., and Srivastava, J. (1999). Data
preparation for mining world wide web browsing pat-
terns. Knowledge and Information Systems, 1(1):5–
32.
Draheim, M.-D., Hanser, C., and von Seckendorff, C.
(2005). E-business case studies web log analysis: test-
berichte.de seminar paper.
Hirate, Y. and Yamana, H. (2006). Sequential pattern min-
ing with time intervals. In Ng, W. K., Kitsuregawa,
M., Li, J., and Chang, K., editors, The 10th Pacific-
Asia Conference on Knowledge Discovery and Data
Mining (PAKDD ’06), volume 3918 of Lecture Notes
in Computer Science, pages 775–779. Springer-Verlag
New York, Inc.
Hofgesang, P. I. (2006). Relevance of time spent on web
pages. In WebKDD 2006: KDD Workshop on Web
Mining and Web Usage Analysis, in conjunction with
the 12th ACM SIGKDD International Conference on
Knowledge Discovery and Data Mining (KDD ’06).
Inokuchi, A., Washio, T., and Motoda, H. (2000). An
apriori-based algorithm for mining frequent substruc-
tures from graph data. In The 4th European Confer-
ence on Principles of Data Mining and Knowledge
Discovery (PKDD ’00), pages 13–23. Springer-Verlag
New York, Inc.
Iv
´
ancsy, R. and Vajk, I. (2006). Frequent pattern mining in
web log data. Acta Polytechnica Hungarica, Journal
of Applied Science at Budapest Tech Hungary, Special
Issue on Computational Intelligence, 3(1):77–90.
Kuramochi, M. and Karypis, G. (2001). Frequent subgraph
discovery. In The 2001 IEEE International Confer-
ence on Data Mining (ICDM ’01), pages 313–320.
IEEE Computer Society.
Liu, B. (2006). Web Data Mining: Exploring Hyperlinks,
Contents, and Usage Data (Data-Centric Systems and
Applications). Springer-Verlag New York, Inc.
Nakayama, T., Kato, H., and Yamane, Y. (2000). Discover-
ing the gap between web site designers’ expectations
and users’ behavior. Comput. Networks, 33(1-6):811–
822.
Nijssen, S. and Kok, J. N. (2004). A quickstart in frequent
structure mining can make a difference. In Tthe 10th
ACM SIGKDD International Conference on Knowl-
edge Discovery and Data Mining (KDD ’04), pages
647–652. ACM.
Pei, J., Han, J., Mortazavi-Asl, B., Pinto, H., Chen, Q.,
Dayal, U., and Hsu, M.-C. (2001). PrefixSpan mining
sequential patterns efficiently by prefix projected pat-
tern growth. In The 17th International Conference on
Data Engineering (ICDE ’01), pages 215–226. IEEE
Computer Society.
Raymond, K. and Hendrik, B. (2000). Web mining re-
search: A survey. SIGKDD Explor. Newsl., 2(1):1–15.
Recupero, D. R. and Shasha, D. (2007). GraphClust.
http://cs.nyu.edu/shasha/papers/GraphClust.html.
Srikant, R. and Agrawal, R. (1996). Mining sequential
patterns: Generalizations and performance improve-
ments. In Apers, P. M. G., Bouzeghoub, M., and Gar-
darin, G., editors, The 5th International Conference
on Extending Database Technology (EDBT), volume
1057, pages 3–17. Springer-Verlag New York, Inc.
Srivastava, J., Cooley, R., Deshpande, M., and Tan, P.-N.
(2000). Web usage mining: Discovery and applica-
tions of usage patterns from web data. SIGKDD Ex-
plorations, 1(2):12–23.
Su, J.-H. and Lin, W.-Y. (2004). CBW: An efficient algo-
rithm for frequent itemset mining. The 37th Hawaii
International Conference on System Sciences (HICSS
’04), 3:30064.3.
Tec-Ed (1999). Assessing web site usability from server log
files.
Termier, A., Rousset, M.-C., and Sebag, M. (2004).
DRYADE: A new approach for discovering closed fre-
quent trees in heterogeneous tree databases. In The
4th IEEE International Conference on Data Mining
(ICDM ’04), pages 543–546. IEEE Computer Society.
Termier, A., Tamada, Y., Numata, K., Imoto, S., Washio, T.,
and Higuchi, T. (2007). DIGDAG, a first algorithm
to mine closed frequent embedded sub-DAGs. In The
5th International Workshop on Mining and Learning
with Graphs (MLG ’07).
Uno, T., Kiyomi, M., and Arimura, H. (2004).
LCM ver. 2: Efficient mining algorithms for fre-
quent/closed/maximal itemsets. In Proceedings of the
IEEE ICDM ’04 Workshop on Frequent Itemset Min-
ing Implementations (FIMI ’04).
Xia, Y. and Yang, Y. (2005). Mining closed and maximal
frequent subtrees from databases of labeled rooted
trees. IEEE Transactions on Knowledge and Data En-
gineering, 17(2):190–202.
Yan, X. and Han, J. (2002). gspan: Graph-based substruc-
ture pattern mining. In IThe 2002 IEEE International
Conference on Data Mining (ICDM ’02), pages 721–
724. IEEE Computer Society.
Yan, X. and Han, J. (2003). Closegraph: mining closed
frequent graph patterns. In The 9th ACM SIGKDD In-
ternational Conference on Knowledge Discovery and
Data Mining (KDD ’03), pages 286–295. ACM.
Yang, Z., Wang, Y., and Kitsuregawa, M. (2005). LAPIN:
Effective sequential pattern mining algorithms by last
position induction. Technical report, Tokyo Univer-
sity.
Zaki, M. J. (2001). SPADE: An efficient algorithm
for mining frequent sequences. Machine Learning,
42(1/2):31–60.
Zaki, M. J. (2002). Efficiently mining frequent trees in a
forest. In The 8th ACM SIGKDD International Con-
ference on Knowledge Discovery and Data Mining
(KDD ’02), pages 71–80. ACM.
WEBIST 2008 - International Conference on Web Information Systems and Technologies
320