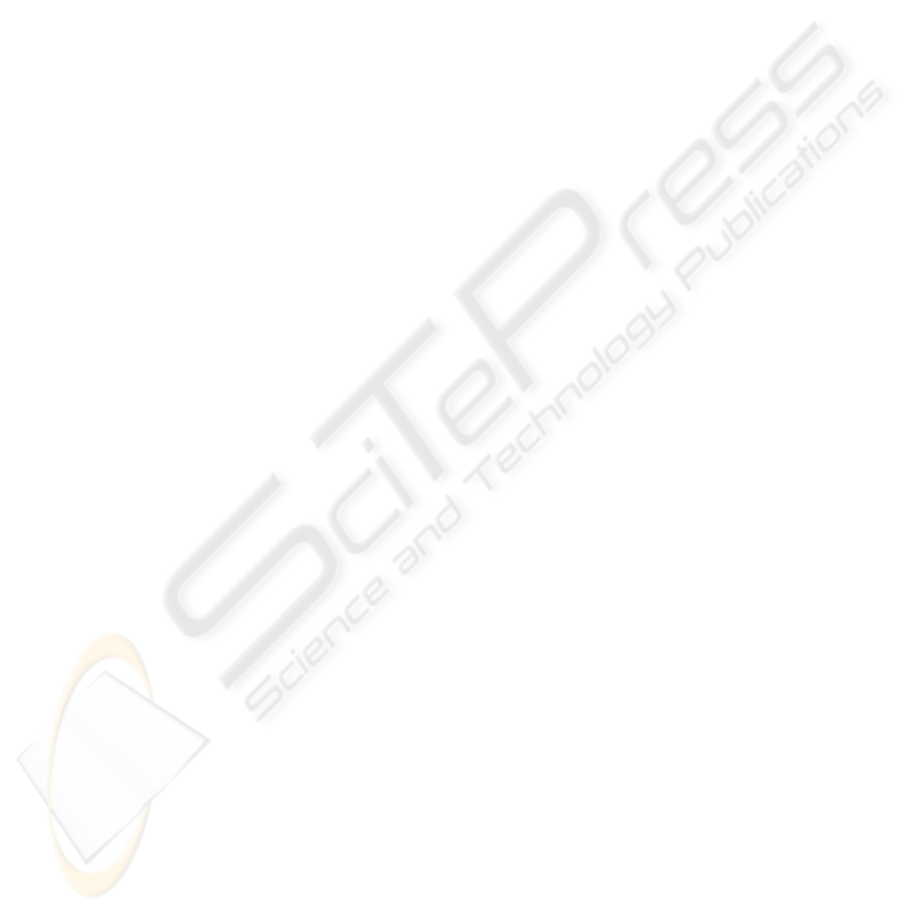
class A which are shared with class B. The
DeMorgan'sLaw Class may have the property
nonCommonInstance to represent the complement of
the common instances between the input classes.
Of the
Auxiliary Classes, the first class
B
=+ , if the patient is suffering from
sneezing – symptom of common cold – and from
nasal congestion – symptom of both common cold
and acute sinusitis – then the patient is infected by
common cold for sure, while more investigations are
required to decide whether he/she infected by acute
sinusitis as well. The second class of the Auxiliary
Classes
,
A
AA
=+
−
, states that if the
patient is suffering from sneezing – symptom of
common cold – and from pain and swelling around
eyes – symptom of acute sinusitis but not of
common cold – then the patient is infected either by
common cold or acute sinusitis or both diseases.
The third
Auxiliary Class,
BCACABA +=++ ))((
, indicates that if the
patient is suffering from common cold – disease A –
and sometimes suffering from acute sinusitis or
pneumonia, so the patient for sure suffering from
bad breath.
4 CONCLUSIONS
In this paper, we introduced new ontology classes.
The minusOf class is used for example to represent
the symptoms of a disease except those shared with
some other disease which implies that the patient is
infected by one but not the other disease. The
DeMorgan’s Law class is utilized to check the
availability and non-availability of common
symptoms of more than one disease, which implies
that the patient is not infected by all diseases causing
the shared non-available symptoms. Finally, three
auxiliary classes are defined to represent various
symptoms’ availability.
The
minusOf and DeMorgan's Law classes
introduced in this paper will play prime role on
easing the representation of complex knowledge,
especially medical knowledge, and the knowledge
that requires such sort of complex classes. The three
auxiliary classes defined are meant to ease the
querying process by deducing the common instances
and individuals of different classes.
In the future more of such specialized classes may
be introduced in order to enhance OWL’s level to
represent more complex knowledge. Also, similarity
may be drawn with implementing combination of
logic gates that subsume certain knowledge,
consequently easing the processing of related data
sets.
REFERENCES
Bennacer, N. Bourda, Y. Doan, B. "Formalizing for
querying learning objects using OWL", Supelec,
France Sept. 2004; ISBN: 0-7695-2181-9
M. Ashburner, C. A. Ball, J. A. Blake, D. Botstein, H.
Butler, J. M. Cherry, A. P.Davis, K. Dolinski, S. S.
Dwight, J. T. Eppig, M. A. Harris, D. P. Hill, L. Issel-
Tarver, A. Kasarskis, S. Lewis, J. C. Matese, J. E.
Richardson, M. Ringwald, G. M.Rubin, and G.
Sherlock. Gene ontology: tool for the unification of
biology. the gene ontology consortium. Nat Genet,
25(1):25–9, 2000. 1061-4036 Journal Article.
Martin Hepp, Jos de Bruijn GenTax: A Generic
Methodology for Deriving OWL and RDF-S
Ontologies from Hierarchical Classifications,Thesauri,
and Inconsistent Taxonomies Int’l Journal on
Semantic Web and Information Systems (IJSWIS), ,
pp. (2006b)
Jarrar, M., Demey, J., Meersman, R.: On Using
Conceptual Data Modeling for Ontology Engineering.
In Spaccapietra, S., March, S., Aberer, K., (Eds.):
Journal on Data Semantics (Special issue on Best
papers from the ER, ODBASE, and COOPIS 2002
Conferences). LNCS, Vol. 2800, Springer, pp.:185-
207. October 2003.
WEBIST 2008 - International Conference on Web Information Systems and Technologies
240