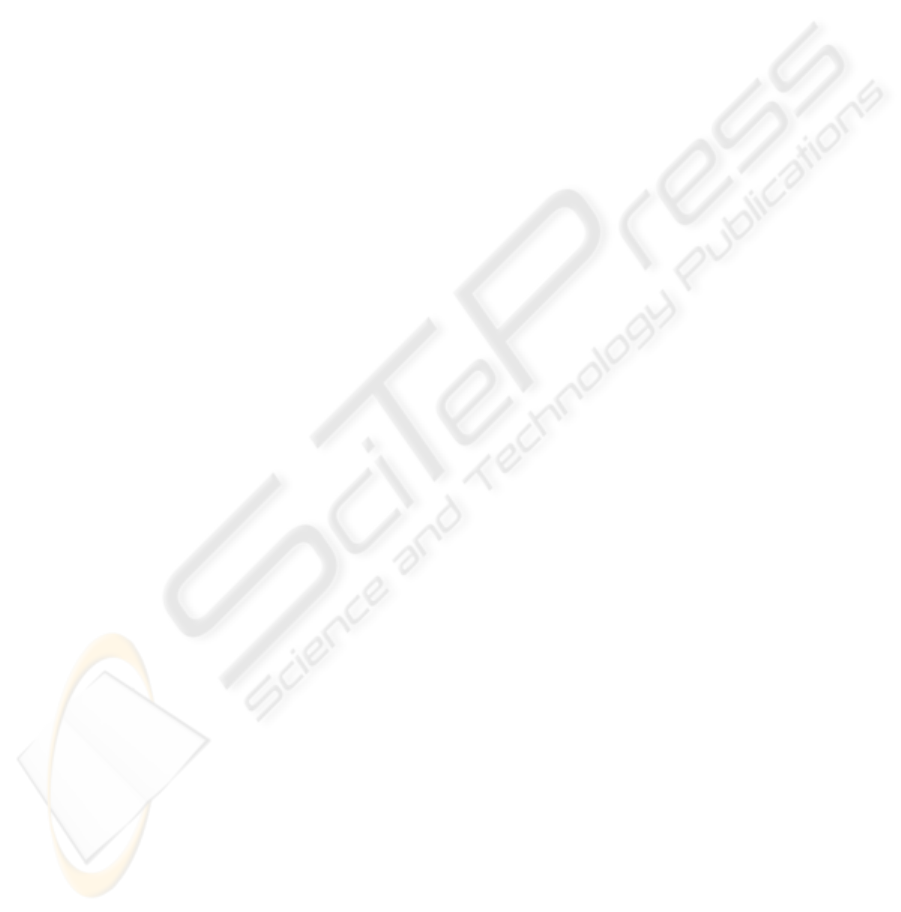
that the recall is much better for the histogram
representation. A better recall is indeed expected to
come together with a reduced precision (accuracy).
In general in ontology learning the recall is more
important than precision. For a domain expert it is
better to delete many spurious (wrong) hyponym
attachments than to miss other correct attachments
because of a reduced recall.
5 CONCLUSIONS AND
FURTHER WORK
We have presented an unsupervised top-down neural
network based approach and framework for
taxonomy enrichment. The experimental results
obtained in the “4 universities” domain are
encouraging, especially when the terms extracted
from the corpus are represented with a reduced
dimensionality, as document category histograms.
Moreover, the best enrichment results have been
achieved when we chose the average vector to
represent every concept in the given initial
taxonomy.
Our framework can also be used as a tool to
assist a domain expert in building an ontology. From
this point of view, the recall is more important than
the accuracy. The domain expert has to manually
prune out the wrong hyponym attachments. As
further work to better evaluate our taxonomy
enrichment framework we will rely more on existing
thesauri like WordNet for evaluating the quality of
the hyponym attachments.
ACKNOWLEDGEMENTS
This work was supported by the grant TD-416
(520/2007) from the National Research Council of
the Romanian Ministry of Education and Research.
REFERENCES
Alfonseca, E., Manandhar, S., 2002. Extending a lexical
ontology by a combination of distributional semantics
signatures. In A. Gómez-Pérez, V.R. Benjamins
(Eds.), 13th International Conference on Knowledge
Engineering and Knowledge Management, LNAI.
Springer, pp. 1-7.
Alfonseca E., Manandhar, S., 2002. An unsupervised
method for general named entity recognition and
automated concept discovery. In 1st International
Conference on General WordNet.
Buitelaar, P., Cimiano, P., Magnini B., 2005. Ontology
learning from text: an overview. In P. Buitelaar, P.
Cimiano, B. Magnini (Eds.), Ontology Learning from
Text: Methods, Evaluation and Applications, Frontiers
in Artificial Intelligence and Applications Series. IOS
Press, pp. 1-10.
Chifu, E.Ş., Leţia, I.A, 2006. Unsupervised ontology
enrichment with hierarchical self-organizing maps. In
I.A. Leţia (Ed.), IEEE 2nd International Conference
on Intelligent Computer Communication and
Processing, pp. 3-9.
Cimiano, P., Völker, J., 2005. Towards large-scale, open-
domain and ontology-based named entity
classification. In RANLP’05, International Conference
on Recent Advances in Natural Language Processing,
pp. 166-172.
Craven, M., DiPasquo, D., Freitag, D., McCallum, A.,
Mitchell, T., Nigam, K., Slattery, S., 2000. Learning to
construct knowledge bases from the World Wide Web.
Artificial Intelligence 118, pp. 69-113.
Cunningham, H., Maynard, D., Bontcheva, K., Tablan, V.,
2002. GATE: a framework and graphical development
environment for robust NLP tools and applications. In
40th Anniversary Meeting of the ACL.
Dittenbach, M., Merkl, D., Rauber, A., 2002. Organizing
and exploring high-dimensional data with the Growing
Hierarchical Self-Organizing Map. In L. Wang, et al.
(Eds.), 1st International Conference on Fuzzy Systems
and Knowledge Discovery, vol. 2, pp. 626-630.
Fellbaum, Chr. (Ed.), 1998. WordNet: An Electronic
Lexical Database, MIT Press. Cambridge, Mass.
Hearst, M.A., Schütze, H., 1993. Customizing a lexicon to
better suit a computational task. In ACL SIGLEX
Workshop on Acquisition of Lexical Knowledge from
Text, 1993.
Kohonen, T., Kaski, S., Lagus, K., Salojärvi, J., Honkela,
J., Paatero, V., Saarela, A., 2000. Self-organization of
a massive document collection. IEEE Transactions on
Neural Networks 11, pp. 574-585.
Maedche, A., Pekar, V., Staab, S., 2003. Ontology
learning part one: on discovering taxonomic relations
from the Web. In N. Zhong, et al. (Eds.), Web
Intelligence, LNCS. Springer, pp. 301-321.
Widdows, D., 2003. Unsupervised methods for developing
taxonomies by combining syntactic and statistical
information. In
HLT-NAACL Conference, pp. 197-204.
Witschel, H.F., 2005. Using decision trees and text mining
techniques for extending taxonomies. In Learning and
Extending Lexical Ontologies by using Machine
Learning Methods, Workshop at ICML-05, pp. 61-68.
WEBIST 2008 - International Conference on Web Information Systems and Technologies
252