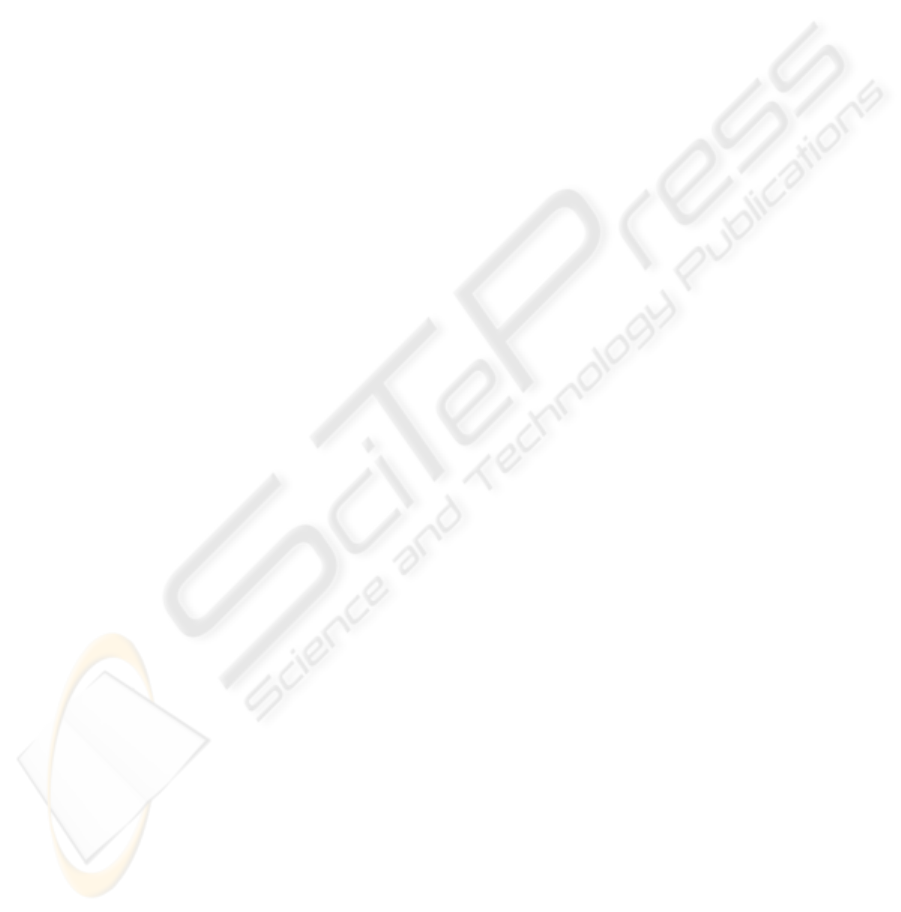
This model may be read in the following way: If
we observe an increase of the number of machine
settings followed by a saturation of the stock in the
time interval [t
1
, t
2
], or if we observe a drift in the
behaviour of the workstation followed by a
saturation of the stock in the time interval [t
3
, t
4
]
then we have the risk to have a breakdown with the
stop of the production line in the interval [t
5
, t
6
].
The application of the stochastic approach
corresponds to the generic level of analysis of our
project. The stochastic approach produces
behavioural models that, according to our
experience, are realistic indeed and can be used to
make prediction or diagnosis for example.
These models will be the base to the proposal of
action plans to improve the performance of the
production line in study.
They can also be used to make new studies,
define new phenomena with high abstraction levels,
etc. This phase will be described in more detail once
the implementation of the algorithms for building
phenomena will be achieved.
6 CONCLUSIONS
We have presented the results of our initial
investigation into the application of knowledge-based
techniques for the analysis of production flows.
To the best of these authors understanding, the
reasoning on the number of produced parts and the
recommendations according to the three criteria,
quality, maintenance and yield, have not been fully
addressed yet. Also, the generic vs. specific analysis
approach will make the tool very flexible and
available to use by the production staff on site (not
necessarily at ease with other possible performance
indicators) and decision makers.
We expect to detect, not only the evident causes
of problems, such as breakdowns or outages; but
also, more subtle aspects, such as fatigue or lack of
training of an operator at a given workstation, and
relations between phenomena with the use of the
stochastic approach (Le Goc et al., 2006).
Today our works are mainly directed towards the
knowledge acquisition stage at our industrial partner,
an important automotive parts provider. We begin
measuring the indicators described in section 3.1 for
his production lines and we will be able, in the near
future, to begin the validation of the knowledge
bases we are building, and to progress into the
definition of phenomena. These data will be used to
implement the graphical tools and the algorithms for
generation of phenomena (Zanni and Bouché, 2008).
This first step achieved we will apply a
stochastic approach on logs of phenomena to obtain
breakdown models and action plans.
Last step of the project will be the definition of a
simulator used to compute the effects of the action
plan, to search means to improve the production.
REFERENCES
Balakrishnan K., Honavar V. (1998). Intelligent diagnosis
systems. Journal of Intelligent and Robotics Systems
40, 207–232.
Benayadi N., M. Le Goc, and P. Bouché (2006).
Discovering Manufacturing Process from Timed Data:
the BJT4R Algorithm. 2nd international workshop on
Mining Complex Data (MCD'06) of the 2006 IEEE
International Conference on Data Mining (ICDM'06),
Hong Kong.
Ishikawa K. (1982). Guide to Quality Control. Unipub /
Quality Resources.
Le Goc M., (2004). The discrete event concept as a
paradigm for the perception based diagnosis of
sachem. Journal of Intelligent Systems 8(3/4), 239–
290.
Le Goc M., (2004). SACHEM, a Real Time Intelligent
Diagnosis System based on the Discrete Event
Paradigm. Simulation, The Society for Modeling and
Simulation International Ed., vol. 80, n° 11, pp. 591-
617.
Le Goc M., Bouché P., Giambiasi N. (2006). Temporal
Abstraction of Timed Alarm Sequences for Diagnosis,
in: the proceedings of COGIS’06, COGnitive systems
with Interactive Sensors, Paris, France.
Tempelbeier H., Burger M. (2001). Performance
evaluation of unbalanced flow lines with general
distributed processing times, failures and imperfect
production. IEE Transactions 33(4), 419–446.
Van Bracht, E. (1995). Performance analysis of a serial
production line with machine breakdowns. In: IEEE
symposium on emerging technologies and factories
automation. Paris, France.
Xie, X. (1993). Performance analysis of a transfer line
with unreliable machines and finite buffers. IEE
Transactions 25(1), 99–108.
Zanni C., Barth, M., Drouard, L. (2007). A Knowledge-
Based Tool for Performance Analysis of Production
Flows. IFAC MCPL 2007 – The 4th International
Federation of Automatic Control Conference on
Management and Control of Production and Logistics,
Sibiu, Rumania.
Zanni C. and Bouché P., “A Global Method for Modelling
and Performance Analysis of Production Flows” in
EUROSIM/UKSIM 2008 10th International
Conference on Computer Modellind and Simulation,
p740-745, Emmanuel College, Cambridge, England,
1-3 April 2008.
A STOCHASTIC APPROACH FOR PERFORMANCE ANALYSIS OF PRODUCTION FLOWS
419