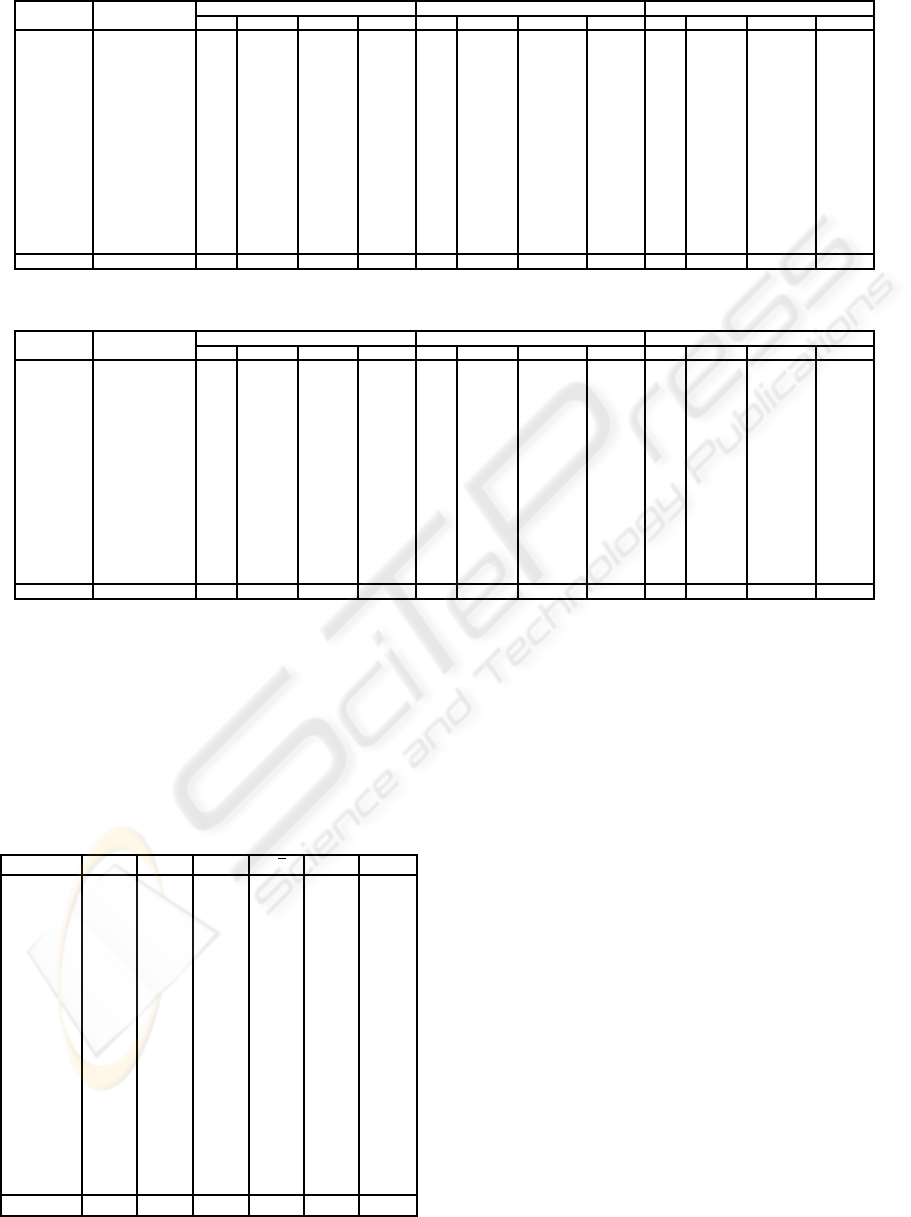
Table 3: Clustering Sampling predictive accuracy comparison (1/2).
Datasets Inst,Attrib, 1% 3% 5%
Classes k acc(%) T(s) red(%) k acc(%) T(s) red(%) k acc(%) T(s) red(%)
Adult 30162,14,2 49 83.12 899.0 88.51 73 83.19 2635.8 66.32 9 82.84 3685.8 52.90
Chess 3196,36,2 1 96.62 3.4 97.00 1 96.62 14.6 87.10 1 96.62 20.9 81.54
German 1000,20,2 9 74.08 0.6 92.11 1 72.10 1.4 81.58 1 72.10 2.1 72.37
Hdigit 10992,16,10 1 99.35 23.5 97.71 1 99.35 62.4 93.93 1 99.35 96.8 90.58
Hypo 3163,25,2 2 96.79 11.3 88.31 2 96.79 25.6 73.53 1 97.39 62.3 35.57
Isegm 2310,19,7 1 97.45 1.6 95.93 1 97.45 3.7 90.59 1 97.45 6.0 84.73
Landsat 6435,36,6 10 90.24 23.5 95.95 1 90.35 58.1 89.99 8 90.41 102.1 82.41
LetterR 20000,16,26 1 95.98 50.2 98.57 3 95.65 146.0 95.85 1 95.98 231.9 93.40
Mushr 8124,22,2 1 100 12.6 97.37 1 100 27.3 94.30 1 100 46.1 90.38
Nurse 12960,8,5 1 97.99 9.0 98.54 1 97.99 23.3 96.21 1 97.99 44.7 92.73
Sflare 1066,12,6 1 72.23 0.1 98.08 1 72.23 0.2 96.15 12 74.19 0.4 92.31
Shuttle 5800,9,6 2 99.55 6.2 96.66 2 99.55 20.6 88.92 9 99.28 31.6 83.00
Sick 3772,29,2 1 96.23 26.1 83.23 1 96.23 68.1 56.23 1 96.23 115.8 25.58
Splice 3190,61,3 223 92.85 11.4 93.96 581 93.67 28.4 84.94 581 93.67 69.7 63.04
Wavef 5000,40,3 34 84.06 35.1 89.19 33 84.12 53.1 83.65 22 83.42 87.7 73.00
Yeast 1484,8,10 4 55.71 0.4 95.45 20 58.69 0.8 90.91 32 58.46 1.3 85.23
Average 89.52 69.6 94.16 89.62 198.1 85.64 89.71 287.8 74.92
Table 4: Clustering Sampling predictive accuracy comparison (2/2).
Datasets Inst,Attrib, 10% 15% 20%
Classes k acc(%) T(s) red(%) k acc(%) T(s) red(%) k acc(%) T(s) red(%)
Adult 30162,14,2 121 83.11 8196.9 − 80 83.17 12074.8 − 107 83.12 14846.7 −
Chess 3196,36,2 2 96.69 45.1 60.16 3 97.10 53.0 53.18 2 96.69 86.4 23.67
German 1000,20,2 23 73.84 4.6 39.47 2 72.32 5.2 31.58 6 73.98 5.5 27.63
Hdigit 10992,16,10 1 99.35 186.5 81.85 1 99.35 249.0 75.76 1 99.35 331.4 67.74
Hypo 3163,25,2 7 97.19 105.5 − 4 97.15 168.3 − 9 97.20 194.3 −
Isegm 2310,19,7 1 97.45 8.4 78.63 1 97.45 10.8 72.52 1 97.45 14.2 63.87
Landsat 6435,36,6 5 90.86 179.7 69.04 3 90.99 270.7 53.37 1 90.35 307.9 46.96
LetterR 20000,16,26 1 95.98 447.6 87.26 1 95.98 651.9 81.45 1 95.98 879.1 74.98
Mushr 8124,22,2 1 100 121.0 74.75 1 100 222.4 53.60 1 100 331.2 30.90
Nurse 12960,8,5 1 97.99 116.1 81.12 1 97.99 207.9 66.20 1 97.99 338.7 44.93
Sflare 1066,12,6 19 74.17 0.8 84.62 19 74.17 1.1 78.85 3 72.98 1.5 71.15
Shuttle 5800,9,6 1 99.66 76.7 58.74 1 99.66 135.9 26.90 1 99.66 193.8 −
Sick 3772,29,2 5 96.24 276.7 − 1 96.23 316.4 − 1 96.23 381.1 −
Splice 3190,61,3 581 93.67 88.0 53.34 413 93.48 139.7 25.93 581 93.67 164.3 12.88
Wavef 5000,40,3 327 84.96 155.2 52.22 336 85.16 150.1 53.79 86 84.92 198.7 38.82
Yeast 1484,8,10 4 55.71 2.0 77.27 9 58.34 3.7 57.95 11 57.99 3.6 59.09
Average 89.80 625.7 50.43 89.91 916.3 31.21 89.85 1142.4 13.93
ating a set of samples so that the exhaustive approach
can be performed to estimate an adequate value for k
in feasible computational time. The difference is on
how the representatives belonging to the set of sam-
ples will be chosen. On random sampling, instead
of performing a clustering algorithm to help find-
ing these representatives, they will be selected ran-
Table 5: Other methods predictive accuracy comparison.
Databases 1-NN 3-NN 5-NN
√
n J48 Bayes
Adult 78.92 81.44 82.29 82.92 85.73 83.64
Chess 96.62 97.10 96.37 90.94 99.38 87.70
German 72.10 72.75 73.22 72.78 71.21 74.30
Hdigit 99.35 99.35 99.24 95.40 96.50 87.64
Hypo 97.39 97.20 97.29 95.71 99.28 98.48
Isegm 97.45 96.19 95.32 90.35 96.91 91.23
Landsat 90.35 90.99 90.86 86.12 86.37 82.05
LetterR 95.98 95.65 95.54 80.97 87.99 74.02
Mushr 100 100 100 98.92 100 95.75
Nurse 97.99 97.99 97.99 96.07 97.12 90.30
Sflare 72.23 72.98 73.29 73.94 74.09 74.37
Shuttle 99.66 99.48 99.36 98.43 99.84 99.13
Sick 96.23 96.28 96.24 94.39 98.72 97.15
Splice 74.42 78.12 79.69 89.37 94.13 95.40
Wavef 72.92 77.72 79.94 84.62 75.43 80.04
Yeast 52.11 55.03 56.76 58.36 55.61 57.71
Average 87.11 88.02 88.34 86.83 88.65 85.56
domly among the instances belonging to the original
database. After that, Algorithm 2 can be performed
the same way as presented on Section 3.
Proportion among classes is again respected. The
random process of choosing representatives is per-
formed on each class separately, in a way that it is
guaranteed that the reduced database has the same
class distribution as the original database.
4.1 Experimental Results
A study similar to the one introduced on Section 3.1
is presented here. The set of databases with 1000 or
more instances was employed. Also, the varied reduc-
tion rates (1%, 3%, 5%, 10%, 15%, and 20%) were
analysed.
Experimental results tables resemble the ones pre-
sented in Section 3.1. Table 6 and Table 7 are anal-
ogous to Table 3 and Table 4, respectively. They
present the accuracy results for the sampling reduc-
tion method, where the bold face values represent
the highest accuracy result for a particular database
among all reduction rates evaluated.
Again, there is a tendency in which the method
achieves greater accuracy results with smaller reduc-
ICEIS 2008 - International Conference on Enterprise Information Systems
464