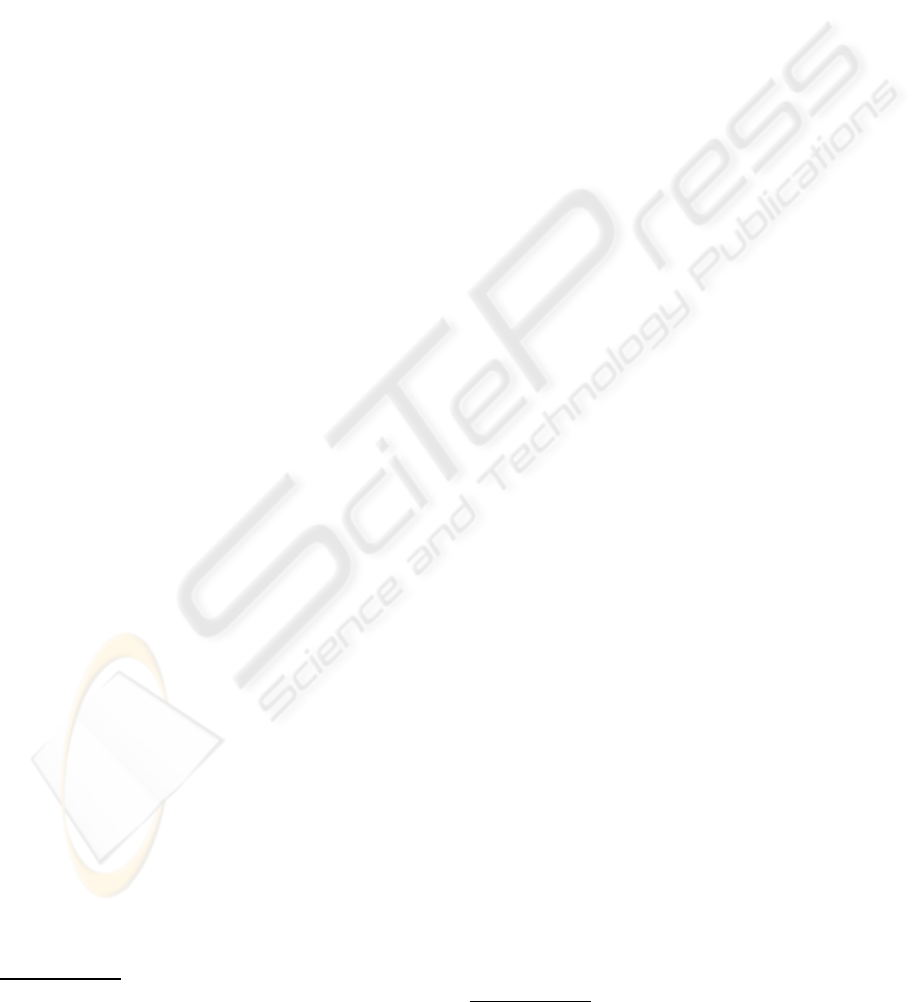
not orthogonal as assumed for now in the Information
Retrieval community. One straightforward extension
to this could be to use a similarity based on the lowest
common ancestor between two nodes (Harel and Tar-
jan, 1984) given a suffix tree (Ukkonen, 1995) based
on the specificity of topics.
In conclusion, we want to stress the point of
having non-binary and bounded similarity measures
which would also enable an easier comparison of
ESSs for a faster decision making process.
5 USING ONTOLOGIES INSTEAD
OF VECTOR SPACE FOR
MODELING EXPERTISE
In the scenario of expert profiling that we are tack-
ling, there are several ways to improve the retrieval
effectiveness using different evidences. One of such
ways is the use of semantics. As done for the web
context (Demartini, 2007), annotations can help to
identify the correct articles to consider for expertise
extraction, knowledge taxonomies can help in finding
the correct experts, and ontologies can help in disam-
biguating multi senses topics.
5.1 Using Ontologies as Expertise
Taxonomies
The expert finding task is usually performed in enter-
prises where the significant knowledge areas are lim-
ited. For this reason the expert finding system usually
adopt customized and manually built taxonomies to
model the organization’s most important knowledge
areas (Becerra-Fernandez, 2006).
In days where the big enterprises cover several
markets, the expertise areas are much more wide than
in the past. For this reason finding expert in the enter-
prise will require much more effort to manually de-
velop a universal expertise taxonomy. We propose
to use the Yago ontology (Suchanek et al., 2007),
that is, a combination of notions from WordNet
1
and
Wikipedia
2
, to model the expertise and to identify
the knowledge areas used to describe people’s knowl-
edge. In this way we can better define the expert pro-
files according to Yago. For example, knowing that
“Macintosh computer” is a subclass of “Computers”
can help the system when there are no results for the
query “Find an expert on Computer”. The system can
proceed looking for experts in the relative subcate-
gories. More, if we know that “Eclipse” is a “Java
1
http://wordnet.princeton.edu/
2
http://wikipedia.org/
tool” we can assume that an expert on Eclipse will be
an expert (with score proportional to the number of
children of the class “Java tool”) on Java tools.
5.2 Using Wordnet to Disambiguate
Expertise Topics
In the enterprise context there is one more problem to
take into account: the topic ambiguity. Multi sense
terms might represent topics of expertise. For exam-
ple, an expert on “Bank” might be expert on only one
of the several senses of this noun: slope/incline | fi-
nancial institution/organization| ridge | array | reserve
| ...
3
Using, for example, the algorithm JIGSAW (Se-
meraro et al., 2007) for word sense disambiguation
we can disambiguate between different topics of ex-
pertise. JIGSAW calculates the similarity between
each candidate meaning for an ambiguous word and
all the meanings in its context defined as words with
the same POS tag in the same sentence. The simi-
larity is calculated as inversely proportional to path
length between concepts in the WordNet IS-A hier-
archy. The assumption in this case is that the appro-
priate meaning belongs to a similar/same concept as
words in the context belong to. For example, if the
sentence “John Doe manages the Citizen Bank that
has good availability of cash.” is an evidence of the
expertise on the topic “Bank”, we can disambiguate
its sense using the context and, in this case, the mean-
ing of “cash”. The distance between all the meanings
of “Bank” and all the meanings of the nouns in the
context (defined as a window of text surrounding the
term) can be used in order to find the intended sense.
We can then add the sense “financial institution” to
the expertise profile of the candidate “John Doe”.
It is also possible to use co-occurrence statistics to
improve the quality of the profiles. If we take a user
profile we can disambiguate the topics looking at the
context in the related articles. For example, according
to the profile, the user is an expert on “Jaguar” and we
find that in the articles considered in his profile the
word “Car” often co-occur with the word “Jaguar”.
In this way we add the topic “Car” to the expertises of
the user always with the final goal of disambiguation.
When performing profile extension or relevance
feedback, we should anyway pay attention to cases of
expertise drift where a candidate “can have several or
many unrelated areas of expertise” as shown in (Mac-
donald and Ounis, 2007a).
3
from WordNet 3.0
COMPARING PEOPLE IN THE ENTERPRISE
457