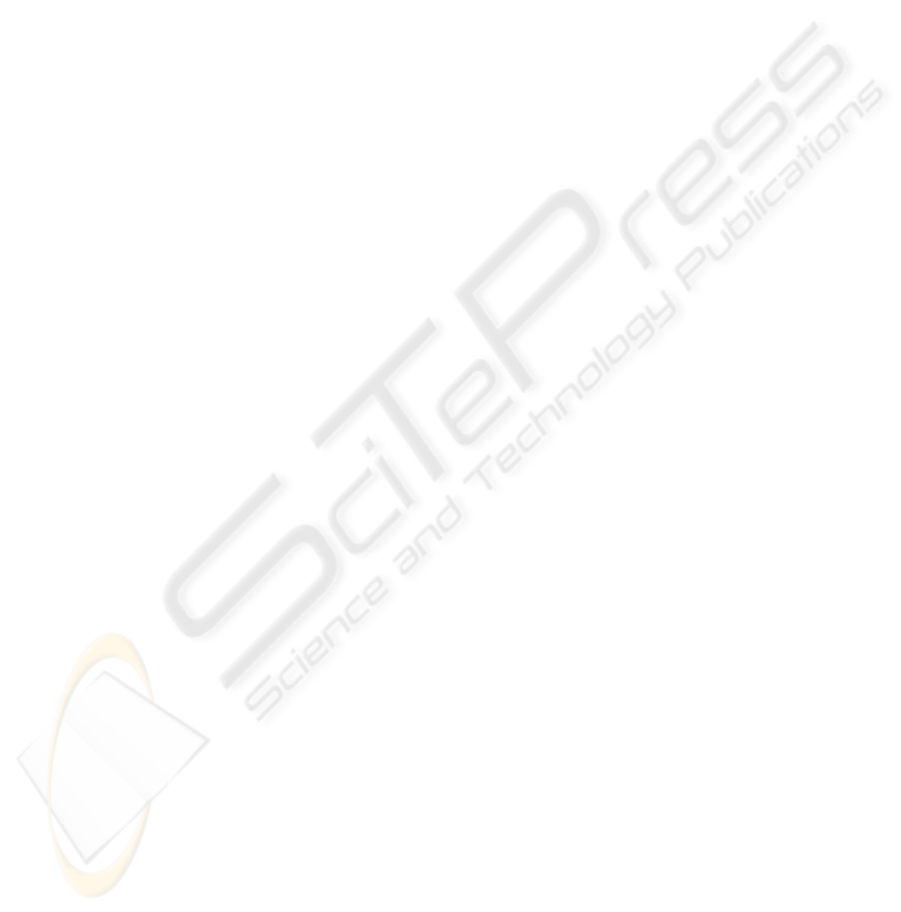
As will be highlighted in the conclusions, this is,
in our opinion, a very pleasant result, because the
manual extraction of a small set of positive
examples guarantee very good results even in light
conditions that were not well represented or not
considered at all. This makes the proposed approach
sometimes preferable with respect to methods that
are more accurate but also more demanding in user
intervention.
5 DISCUSSION AND
CONCLUSIONS
Experimental results demonstrate the capability of
the proposed approach to recognize the ball in image
soccer sequences acquired in different light
conditions.
Ball recognition performance are comparable to
those obtained in previous works by using
appearance based methods involving more complex
training procedures.
Differently from the other appearance based
methods that can be found in the literature, the
proposed one does not require, nevertheless, a long
and tedious phase to build different training sets to
manage different light conditions. Moreover it does
not require any negative training set, avoiding the
difficulties relative to the balancing of the number of
negative and positive examples that occurs when, as
in the soccer context, negative examples abound
(player’s socks, pants or shirts, advertising posters,
etc.).
The usefulness of such approach allows to use
the method in real systems with little user
intervention during the setup phase of the system
installation. Last but not least, the fact that it poses
less emphasis in the acquisition of negative
examples and the balancing with the positive ones,
means that it is less prone to errors when dealing
with previously unknown external objects.
In the reported experiments only one set of 17
ball images acquired in an evening match, was used
to performs ball recognition in any lighting
condition. This is a very pleasant characteristic for a
ball recognition system considering that ball texture
is not uniform and moreover ball appearance can
change also depending on the stadium.
In conclusion, the proposed approach seems to be a
proper trade off between performance, portability
and easiness to start up. Future work will be
addressed to improve classification performance
both using newest vision tools able to avoid
saturation effects on ball surface and introducing
different keypoint matching strategies.
REFERENCES
Atherton, T. J., Kerbyson, d. J., 1999. Size invariant circle
detection. Image and video Computing.
D’Orazio, T., Guaragnella, C., Leo, M., Distante, A.,
2004. A new algorithm for ball recognition using
circle Hough Transform and neural classifier. Pattern
Recognition, 37, 393-408.
Jones, M., Poggio, T., 1997. Model based Matching by
Linear Combinations of Prototypes. Proceedings of
Image Understanding workshop.
Leo, M., D’Orazio, T., Distante, A., 2003. Independent
Component Analysis for Ball Recognition in Soccer
Images. Proceedings of the Intelligent Systems and
Control.
Lowe, D. G., 2004. Distinctive Image Features from
Scale-Invariant Keypoints. International Journal of
Computer Vision, 60, 2, 91-110.
Mohan, A., Papageorgoiu, C., Poggio, T., 2001. Example-
based Object Detection in Images by Components.
IEEE Transactions On Pattern Analysis and Machine
Intelligence, 23, 4, 349-361.
Murase, H., 1995. Visual Learning and Recognition of 3-
D Objects from Appearance. International Journal of
Computer Vision, 14, 5-24.
Papageorgiou, C., Oren, M., Poggio, T., 1998. A general
framework for Object Detection. Proceedings of the
International Conference for Computer Vision.
Ren, J., Orwell, J., Jones, G. A., Xu, M., 2004. A general
framework for 3d soccer ball estimation and tracking,
ICIP 2004, 1935-1938.
Rowley, H., Baluja, S., Kanade, T., 1998. Neural
Network-Based Face Detection. IEEE Trans. On
Pattern analysis and Machine Intelligence, 20, 1, 23-
38.
Tong, X. F., Lu H. Q., Liu Q. S., 2004. An effective and
fast soccer ball detection and tracking method. Pattern
Recognition, ICPR 2004, Proceedings of the 17th
International Conference, 4, 795-798.
Yu, X., Leong, H.W., Xu C., Tian, Q., 2006. Trajectory-
based ball detection and tracking in broadcast soccer
video. IEEE Transactions on Multimedia, 8, 6, 1164-
1178.
ICEIS 2008 - International Conference on Enterprise Information Systems
212