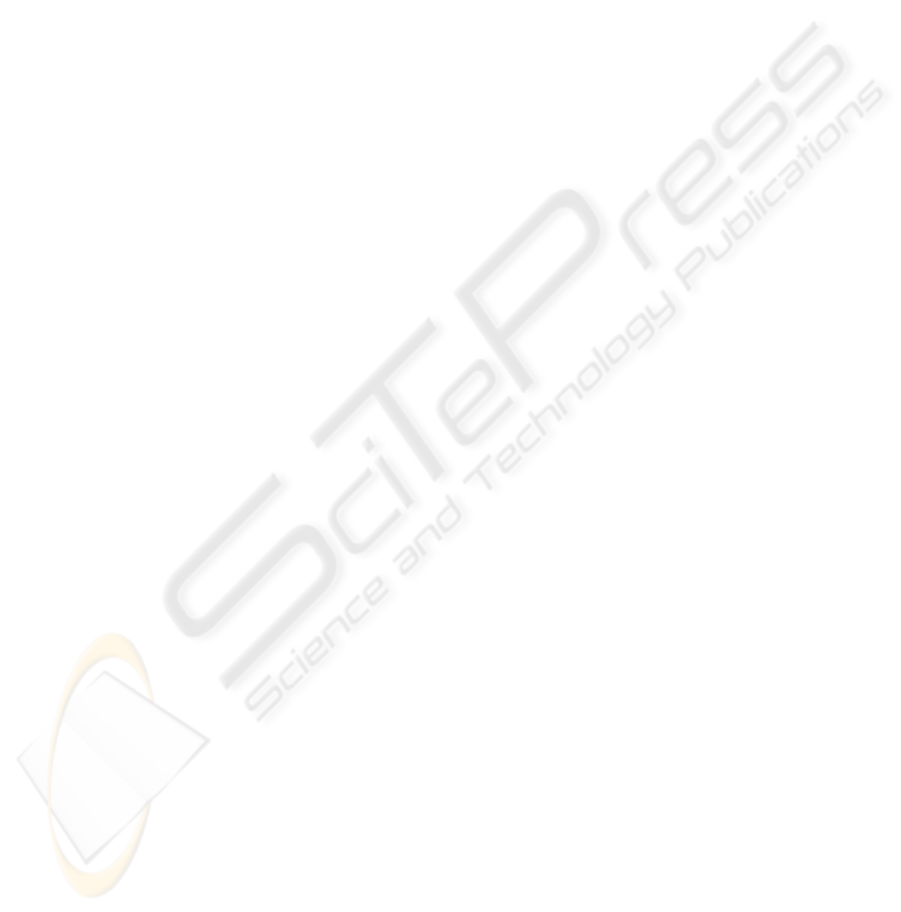
effectiveness of u-Learning systems. Second,
learner's characteristics, academic motivation and
flow played an important role in the effectiveness of
u-Learning. Finally, learner's interactivity factors
had positive influence on the performance of the u-
Learning systems.
6 CONCLUSION AND
DISCUSSION
Under the ubiquitous computing environment, the
learner can use any device to exchange information
with anyone anytime and anywhere. Based on the
characteristics of ubiquity, the area of u-Learning
systems has developed by an increase in
interactivity; however, there are some crucial aspects
needed for the effective launch of u-Learning. There
has been little empirical research regarding
information systems conducted up to now.
Like other empirical studies, this study has several
limitations. First, the questionnaire approach is not
free of subjectivity from the respondent. Second, the
generalizability of our study is subject to debate.
Finally, our study was conducted with a snapshot
research approach. More effort is needed to evaluate
the validity of our findings.
REFERENCES
Balawati, T., “Increasing student persistence in Indonesian
post-secondary distance education”,
Distance
Education
, Vol. 19, No. 1, 1998, pp. 60-71.
BenMoussa, C., “Workers on the move: New
opportunities through mobile commerce”,
UKAIS
Conference
, Vol. 16, 2003, pp. 22-23.
Biner, P. M., R. S. Dean, and A. E. Mellinger, “Factors
underlying distance learner satisfaction with
televised college-level courses”,
The American
Journal of Distance Education
, Vol. 8, 1994. pp.60-
71.
Burgoon, J. K., J. A. Bonito, Bengtsson B., A. Ramirez Jr.,
N. E. Dunbar and N. Miczo, “Testing the
interactivity model: Communication process Partner
assessments and the quality of collaborative work”,
Journal of Management Information Systems, Vol.
16, No. 3, 2000, pp. 33-56.
Chute, A. G., M. M. Thompson and B. W. Hancock,
The
McGraw-Hill handbook of distance learning,
New
York: McGraw-Hill, 1999.
Csikszenntmihalyi, M., Optimal experience:
Psychological of flow in consciousness.
Cambridge
University Press, 1988.
Coldeway, D. O., “Methodological issues in distance
educational research”,
The American Journal of
Distance Education,
Vol. 5, No 2, 1988, pp. 47-52.
Coutaz, J., J. L. Crowley, S. Dobson and D. Garlan,
“Context is Key”, Communications of the ACM, Vol.
48, No. 3, 2005, pp. 49-53.
Chen, L. D., “Consumer Acceptance of Virtual Stores: A
Theoretical Model and Critical Success Factors for
Virtual Stores”,
Doctoral Thesis, The University of
Memphis, 2000.
Eager, J. F., R. D. Blackwell, and P. W. Miniard,
Customer Behavior, 6th Ed., Chicago: The Dryden
Press, 1990.
Fortin, D. R. and R. R. Dholakia, “Interactivity and
vividness effects on social presence and involvement
with a web-based advertisement”, Journal of
Business Research
, Vol. 58, 2005, pp. 387-396.
Ghani, J. A., R. Supnick and P. Rooney, “The Experience
of Flow in Computer Mediated and in Face-to-Face
Groups”, in 12th internet,
Conference on
Information Systems
, 1991, pp. 16-18.
Gibson. P. M., “Generalized doubly stochastic and
permutation matrices over a ring”, Linear Algebra
Appl.
, Vol. 30, 1980, pp. 101-107.
Harasim, L.,
Online education: Perspectives on a new
environment,
In L. Harasim, eds., New York:
Praeger Publisher, 1990.
Hair, J. F., R. E. Anderson, R. L. Tatham and W. C. Black,
Multivariate data analysis(4th ed.). Englewood
Cliffs.: Prentice-Hall, 1995.
Heeter, C., Implications of New Interactive Technologies
for Conceptualizing Communication, in Media Use
in the Information Age: Emerging Patterns of
Adoption and Computer Use
, J. L. Salvaggio and J.
Bryant, eds., Hillsdale: Lawrence Erlbaum
Associates, 1989.
Hicks, W. D. and R. J. Klimosky, “Entry into Training
Outcomes: A Field Experiment”,
Academy of
Management Journal
, Vol. 30, 1987, pp. 542-552.
Hoffman, D. L. and T. P. Novak, “Marketing in
Hypermedia Computer-Mediated Environments:
Conceptual Foundations”,
Journal of Marketing, Vol.
60, 1996, pp. 50-68.
Hult, G. T. and D. Ketchen, “Does market orientation
matter?: a test of the relationship between positional
advantage and performance”,
Strategic Management
Journal,
Vol. 22, No. 9, 2001, pp. 899-906.
Jarvenpaa, S. J. and P. A. Todd, “Consumer reactions to
eletronic shopping on the world wide web”,
International Journal of Electronic Commerce, Vol.
1, No. 2, 1997, pp. 59-88.
Jonassen, D., “Instructional design models for well-
structured and ill-structured problem solving
learning outcomes”,
ETR&D, Vol. 45, No. 1, 1997.
Junglas, I. A. and R. T. Watson, “U-Commerce: An
Experimental Investigation of Ubiquity and
Uniqueness”,
24th ICIS, 2003, pp. 414-426.
Kalakota, R. and M. Robinson,
M Business, McGraw-Hill
Company, 2002.
Kannan, P., A. Chang and A. Whinston, “Wireless
Commerce”,
Marketing Issues and Possibilities,
34th HICCS,
Vol. 9, No. 9, 2001, pp. 15-21.
Khan, B. H., Factors to consider when evaluating a web-
based instruction course; A survey, Khan, B. H.
FACTORS INFLUENCING THE LEARNING PERFORMANCE OF u-LEARNING SYSTEMS
357