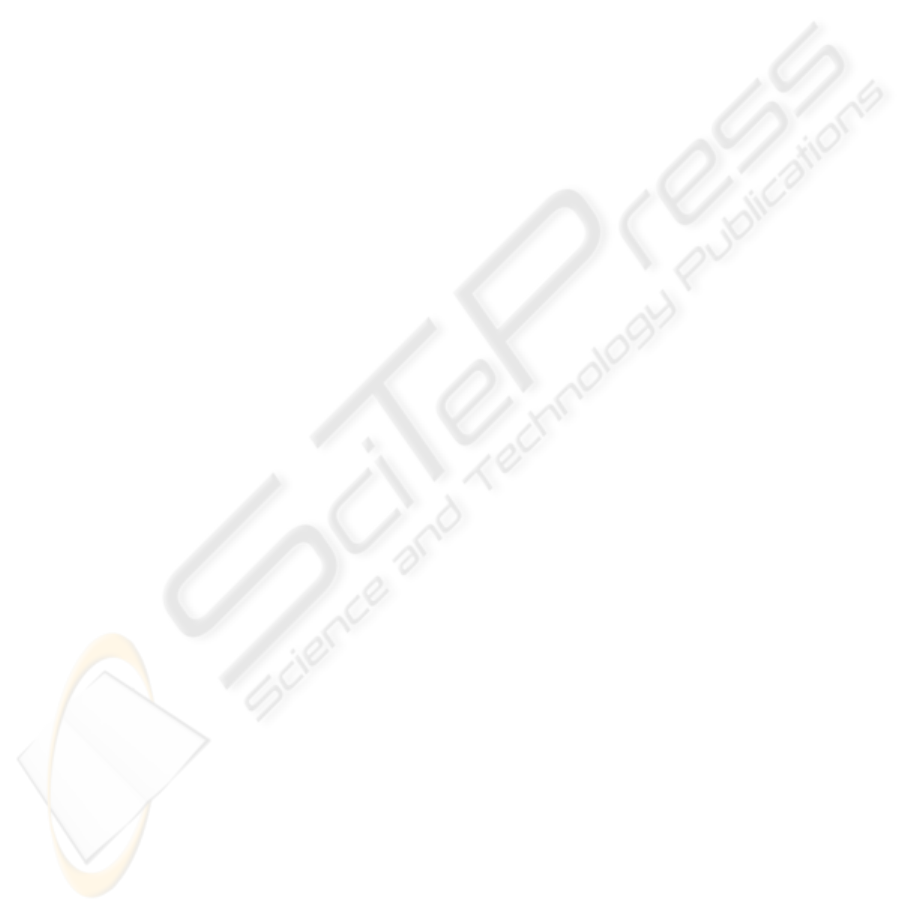
extrapolate data for accurate predictions that can
support the decision making process. This is of great
interest as data mining will facilitate a greater level
of accuracy due to its natural ability to find trends of
significance in arbitrary data. Furthermore the
reporting feature (which forms an aspect of the
visualisation aspect of BI) will require that the
trends, patterns and information discovered by the
platform be presented to the user in a manner that is
easy to use and analyse, enabling the information to
be efficiently utilised and support organisations with
decisions regarding operations and future direction
with reports that can be easily understood by any
employee, not just experts.
The reporting feature will also lend itself to
Data profiling, as these reports will enable the BI
prospector engine to collect statistics and
information about data in order to:
1. Find out whether existing data can easily be
used for other purposes.
2. Provide metrics upon data quality including
whether the data conforms to company
standards.
3. Assess the risk involved in integrating data for
new applications, including the challenges of
joins.
4. Track data quality.
5. Assess whether metadata accurately describes
the actual values in the source database.
6. Understanding data challenges early in any data
intensive project, so that late project surprises
are avoided. Finding data problems late in the
project can incur time delays and project cost
overruns.
7. Encompass an enterprise view of all data, for
uses such as Master Data Management where
key data is needed, or Data governance for
improving data quality.
Consequently, the Reporting facility will enable
platform to take snapshots of the data and statistics
be it from profiling or forecasting/prediction to
provide managers with details and performance
indicators upon which decisions can be made.
REFERENCES
Arnott, D., Pervan, G., 2007. Eight key issues for the
decision support systems discipline. In Decision
Support Systems. Available online 1 October 2007
http://www.sciencedirect.com
Finnie, G.; Barker, J., (2005) Real-time business
intelligence in multi-agent adaptive supply networks.
In e-Technology, e-Commerce and e-Service, 2005.
EEE '05. Proceedings. The 2005 IEEE International
Conference on 29 March-1 April 2005 Page(s):218 –
221
Fong, A.C.M., Hui, S.C., Jha, G., 2002. Data mining for
decision support. In IT Professional, Volume 4, Issue
2, March-April 2002 Page(s):9 – 17. IEEE Xplore.
Han J, Kamber M. 2006. Data mining: concepts and
techniques. Morgan Kaufman. Los Altos, CA, 2
nd
edition.
Hasan, H., Hyland, P., 2001. Using OLAP and
multidimensional data for decision making. In IT
Professional Volume 3, Issue 5, Sept.-Oct. 2001
Page(s):44 – 50. IEEE Xplore
Lawton, G. 2006. Making Business Intelligence More
Useful. In Computer Volume 39, Issue 9, Sept. 2006
Page(s):14 – 16. IEEE Xplore.
Lee, J. H & Park, S. C., 2005. Intelligent profitable
customers segmentation system based on business
intelligence tools. Expert Systems with
Applications, Volume 29, Issue 1, July 2005, Pages
145-152. Elsevier Science.
Luhn, H. P., 1958. A Business Intelligence System. In
IBM Journal vol. 2, no. 4, p.314. IBM Available
online: http://www.research.ibm.com/journal/
Mladenic´ D, Lavracˇ N, Bohanec M, Moyle S., 2003.
Data mining and decision support: integration and
collaboration. Kluwer Academic Publishers.
Dordrecht.
Nemati, H. R., Steiger, D. M., Iyer, S. L. Herschel, R. T.,
2002. Knowledge warehouse: an architectural
integration of knowledge management, decision
support, artificial intelligence and data warehousing.
In Decision Support Systems, Volume 33, Issue 2, June
2002, Pages 143-161. Elsevier Science.
Pedersen, T.B., Jensen, C.S., 2001. Multidimensional
database technology. In Computer, Volume 34, Issue
12, Dec. 2001 Page(s):40 - 46. IEEE Xplore
Rupnik, R., Kukar, M., Bajec, M., Krisper, M., 2006.
DMDSS: data mining based decision support system
to integrate data mining and decision support
In Information Technology Interfaces, 2006. 28th
International Conference on
2006 Page(s):225 – 230.
Simmers, C., 2004. A stakeholder model of business
intelligence. In Proceedings of the 37th Annual
Hawaii International Conference on
5-8 Jan. 2004
UCI Machine learning Repository, Available online:
www.ics.uci.edu/~mlearn/MLRepository.html
Weka Project home page at University of Waikato in New
Zealand. Available online:
http://www.cs.waikato.ac.nz/ml/weka/
Witten, I., Frank E., 2005. Data Mining: Practical
machine learning tools and techniques. Morgan
Kaufmann, San Francisco. 2
nd
Edition
Zhang, F., Yang, B., Song, W., Li, L., 2007.
Intelligent Decision Support System Based on Data
Mining: Foreign Trading Case Study
Control and Automation. In IEEE International
Conference on
May 30 2007-June 1 2007 Page(s):1487 - 1491 ICCA
2007.
ANALYSING MULTIDIMENSIONAL DATABASES USING DATA MINING AND BUSINESS INTELLIGENCE TO
PROVIDE DECISION SUPPORT
479