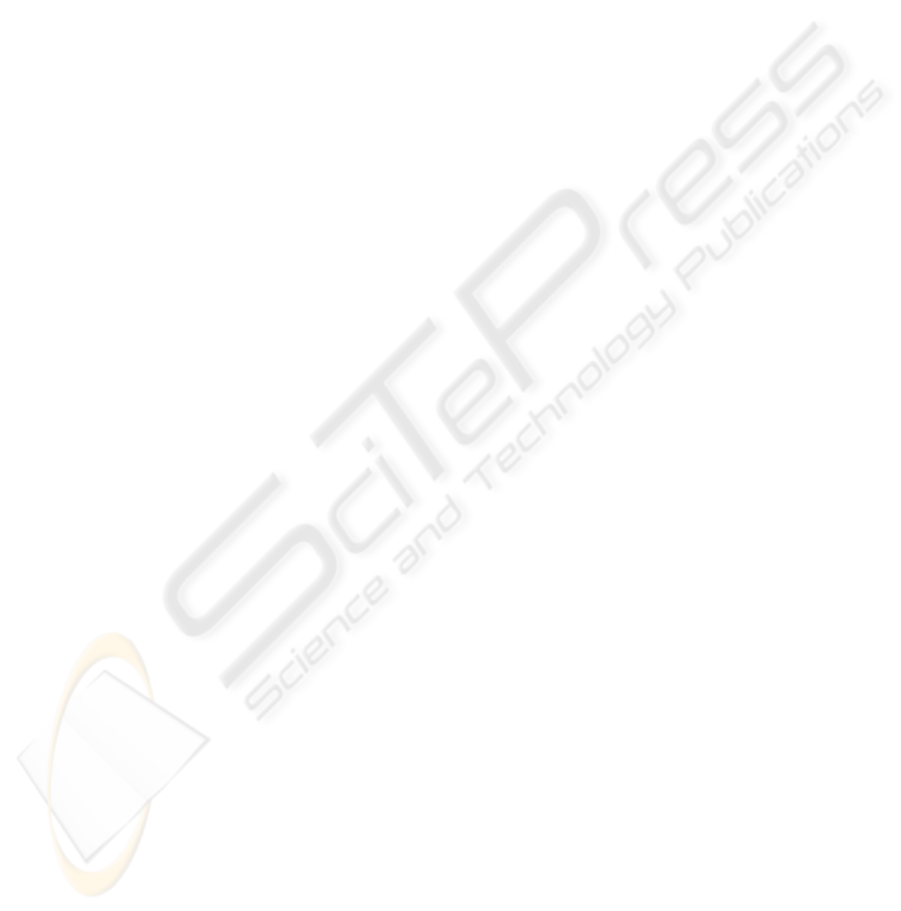
of unique valid starters) and singletons (0.017% of
unique valid singletons), and fifty frequent attrac-
tors (0.017% of unique valid attractors) accounted for
about 20% of total occurrences. One hundred starters
(0.086% of unique valid starters) and one thousand at-
tractors (0.35% of unique valid attractors) constituted
about 45% and 48% of total occurrences, respectively.
Analogously, one hundred twenty singletons (0.21%
of unique valid singletons) compounded to about 37%
of total occurrences.
Knowledge workers were generally more familiar
with the starting navigation points rather than the tar-
gets. Smaller number of starters repeats substantially
more frequently than the adequate number of attrac-
tors. That is, the users knew where to start and were
familiar with the navigational path to the target (in-
stead of just utilizing shortcuts such as bookmarks).
In and out degrees of frequent starters are also signif-
icantly higher than those of attractors (see Figures 1
and 2). The frequent starters have in and out degrees
between 5000 and 20000, whereas the frequent attrac-
tor in degrees are between 1000 and 6800, and out
degrees between 1000 and 3400.
Complex networks of knowledge worker browsing
behavior differ from the web topology constituted by
links. Hubs in the web topology are the pages with
large number of incoming and outgoing links. Behav-
ioral hubs are the navigation points that have large in
and out degrees — resulting from the user traversal
patterns. It has been discovered that the behavioral
hubs in the knowledge worker navigation space did
not substantially match the link hubs. High out de-
grees of behavioral hubs (reaching almost 7000) also
significantly exceed the number of links on the served
pages at any given time.
5 CONCLUSIONS
We introduced a novel analytic framework for explo-
ration and modeling of human browsing behavior in
electronic environments. It utilizes a temporal seg-
mentation of browsing activity. The framework was
applied to browsing behavior analysis of the knowl-
edge workers on a large enterprise information sys-
tem. Numerous vital behavioral features have been
revealed. Knowledge worker browsing behavior con-
centrated on the navigational starters. They remem-
bered the starting point and recalled the navigational
path to the target. The knowledge workers effectively
utilized only a small amount of available resources.
A large number of resources have been occasionally
accessed.
Topology of knowledge worker traversal path-
ways resembles complex networks. However, the be-
havioral complex network differs from the hypertext
link network. The traversal hubs do not identically
correspond to the link hubs. Significant long tail char-
acteristics of the essential navigation points have been
exposed both in terms of frequencies as well as in and
out degrees.
REFERENCES
Adomavicius, G. and Tuzhilin, A. (2005). Toward the
next generation of recommender systems: A survey
of the state-of-the-art and possible extensions. IEEE
Transactions on Knowledge and Data Engineering,
17:734–749.
Agichtein, E., Brill, E., and Dumais, S. (2006). Improv-
ing web search ranking by incorporating user behav-
ior information. In Proceedings of The 29th SIGIR,
pp. 19–26, Seattle, Washington, USA.
Barabasi, A.-L. (2005). The origin of bursts and heavy tails
in human dynamics. Nature, 435:207–211.
Baraglia, R. and Silvestri, F. (2007). Dynamic personaliza-
tion of web sites without user intervention. Commu-
nications of the ACM, 50:63–67.
Bedue, C., Baeza-Yates, R., Ribeiro-Neto, B., Ziviani, A.,
and Ziviani, N. (2006). Modeling performance-driven
workload characterization of web search systems. In
Proceedings of CIKM, pp. 842–843, Arlington, USA.
Caldarelli, G. (2007). Scale-Free Networks: Complex Webs
in Nature and Technology. Oxford University Press,
Cambridge, UK.
Catledge, L. and Pitkow, J. (1995). Characterizing browsing
strategies in the world wide web. Computer Networks
and ISDN Systems, 27:1065–1073.
Dezso, Z., Almaas, E., Lukacs, A., Racz, B., Szakadat, I.,
and Barabasi, A.-L. (2006). Dynamics of information
access on the web. Physical Review, E73:066132(6).
Downey, A. (2005). Lognormal and pareto distributions in
the internet. Computer Communications, 28:790–801.
G
´
eczy, P., Akaho, S., Izumi, N., and Hasida, K. (2007). Us-
ability analysis framework based on behavioral seg-
mentation. In Psaila, G. and Wagner, R., Eds., Elec-
tronic Commerce and Web Technologies, pp. 35–45,
Springer-Verlag, Heidelberg.
Jin, R., Si, L., and Zhai, C. (2006). A study of mixture mod-
els for collaborative filtering. Information Retrieval,
9:357–382.
Leskovec, J., Kleinberg, J., and Faloutsos, C. (2005).
Graphs over time: Densification laws, shrinking di-
ameters and possible explanations. In Proceedings of
KDD, pp. 177–187, Chicago, Illinois, USA.
Moe, W. (2003). Buying, searching, or browsing: Differen-
tiating between online shoppers using in-store naviga-
tional clickstream. Journal of Consumer Psychology,
13:29–39.
ICEIS 2008 - International Conference on Enterprise Information Systems
238