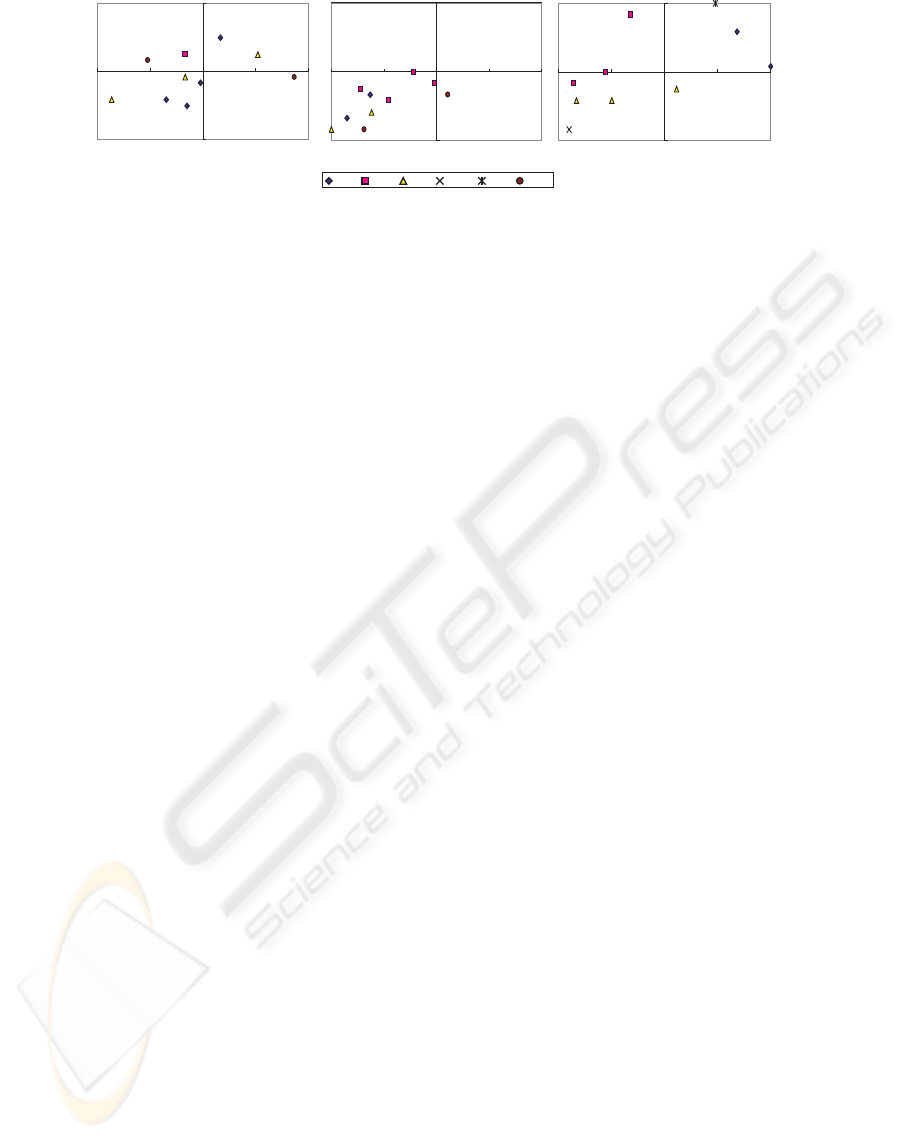
㪄㪈
㪇
㪈
㪄㪈 㪄㪇㪅㪌 㪇 㪇㪅㪌 㪈
㪄㪈
㪇
㪈
㪄㪈 㪄㪇㪅㪌 㪇 㪇㪅㪌 㪈
㪄㪈
㪇
㪈
㪄㪈 㪄㪇㪅㪌 㪇 㪇㪅㪌 㪈
㪤㪼㫊㫊㪸㪾㪼㩷㪈㪏㪄㪉㪎
㪤㪼㫊㫊㪸㪾㪼㩷㪉㪏㪄㪊㪎
㪤㪼㫊㫊㪸㪾㪼㩷㪊㪏㪄㪋㪎
Figure 3: Message feature maps for the messages 18th-27th (left), 28th-37th (middle), and 38th-47th (right).
4 CONCLUSIONS
In this paper, we proposed a message feature map,
which was a visualization for plotting discussion mes-
sages on a plane with two axes. We also introduced
two metrics; centrality and novelty. This map gave us
an intuitive understanding and quick grasp of discus-
sion status. We showed the experimental results with
using the data collected in a real on-line focus group
discussion, and presented some scenarios for facilita-
tion support usages by the message feature maps.
Our future works include to build a message tran-
sition model which tells how message status transit on
the message feature map, and to simulate discussion
for a given set of participants. Discussion simulation
will be a very useful tool for discussion planning -
determining the discussion goal, grouping the people,
building strategic facilitation scenarios.
5 RELATED WORKS
Several tools for on-line focus group
discussions have been introduced for
these years (Zoomerang Online Focus
http://info.zoomerang.com/prodserv/onlinefocus.htm,
GMI Focus Group Software https://www.gmi-
mr.com/net-mr/online-focus-groups.php, and e-
Focusgroups http://www.efocusgroups.co.uk/). For
example, MarketTools, Inc. has launched Zoomerang
Online Focus, which is a web-based solution that
helps marketers conduct focus group research on-
line. One of its selling points is that it provides
highly skilled and trained facilitators (, which they
call moderators). Our work aims to have profitable
on-line focus group discussions without depending
on facilitators’ skills.
Various methods have been proposed for finding
important terms from text (key phrases (Witten et al.,
1999), topic words (Lawrie et al., 2001)). Some
works have focused on finding key persons in text-
based communication (Kamimaeda et al., 2005; Re-
ich et al., 2002), and on exploring social networks
of network-based communication (Zhou et al., 2006).
These are very effective and high quality analyses,
which give us deep insight into each aspect. In or-
der to analyze in various aspects, they need to use
multiple analyses and compare them. This might be
time-consuming work for facilitators. Our goal is to
propose methods or tools which give us multi infor-
mative analysis on a single output.
ACKNOWLEDGEMENTS
We would like to thank to Hakuhodo Inc. for their
project collaboration. This work was sponsored by
the Air Force Office of Scientific Research, Air Force
Materiel Command, USAF (AF9550-06-1-0096 and
AF9550-06-1-0370).
REFERENCES
Imafuji Y., N., Llor`a, X., Goldberg, D. E., Y., W., and D., T.
(2007). Delineating topic and discussant transitions in
online collaborative environments. In Proceedings of
9th International Conference on Enterprise Informa-
tion Systems (ICEIS 2007).
Kamimaeda, N., Izumi, N., and Hasida, K. (2005). Dis-
covery of key persons in knowledge creation based on
semantic authoring. In KMAP 2005.
Lawrie, D., Croft, W. B., and Rosenberg, A. (2001). Finding
topic words for hierarchical summarization. In SIGIR
’01: the 24th ACM SIGIR conference on Research and
development in information retrieval, pages 349–357.
Reich, J. R., Brockhausen, P., Lau, T., and Reimer, U.
(2002). Ontology-based skills management: Goals,
opportunities and challenges. Universal Computer
Science, 8(5):506–515.
Witten, I. H., Paynter, G. W., Frank, E., Gutwin, C., and
Nevill-Manning, C. G. (1999). Kea: practical auto-
matic keyphrase extraction. In DL ’99: the fourth
ACM conference on Digital libraries, pages 254–255.
Zhou, D., Manavoglu, E., Li, J., Gies, C., and Zha,
H. (2006). Probabilistic models for discovering e-
communities. In Proceedings of the 15th international
conference on World Wide Web (WWW2006).
ICEIS 2008 - International Conference on Enterprise Information Systems
566