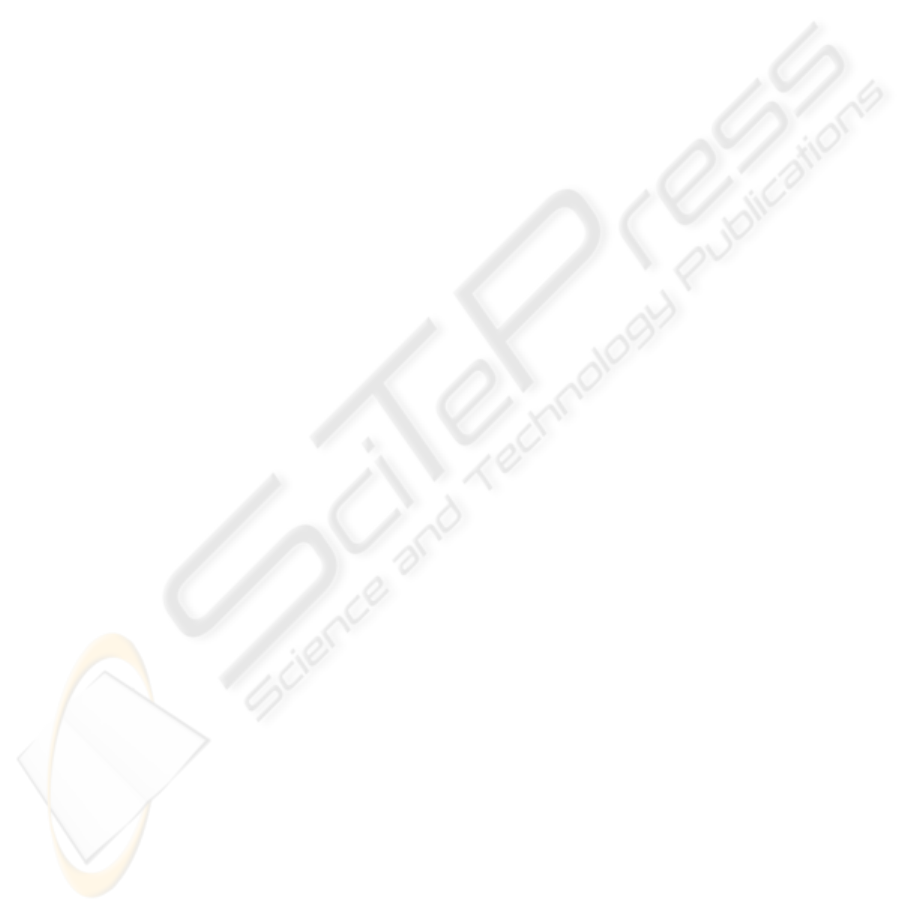
MULTIPLICATIVE NEURAL NETWORK WITH SWARM
INTELLIGENCE FOR MULTICARRIER TRANSMITTER
Nibaldo Rodriguez, Claudio Cubillos
School Informatic, Pontifical Catholic University of Valparaiso, Av. Brasil 2241, Chile
Orlando Duran
School Mechanic, Pontifical Catholic University of Valparaiso, Av. Brasil 2241, Chile
Keywords:
Neural Networks, Swarm intelligence, Multicarrier Communications.
Abstract:
In this paper, we propose a novel effective distortion compensation scheme suitably developed to reduce
nonlinearities of the traveling wave tube amplifier (TWTA) in orthogonal frequency division multiplexing
(OFDM) systems at the multicarrier transmitter side. This compensator is developed in order to combine in
the most effective way the capability of the quantum particle swarm optimization technique and multiplicative
neural networks. The compensator effectiveness has been tested through computer simulations. The improve-
ments in the reduction of constellation warping and enhanced performance in terms of the bit error rate (BER)
are offered for the TWTA with an input back-off level of 0 dB.
1 INTRODUCTION
Multicarrier Transmitter based on orthogonal fre-
quency division multiplexing (OFDM) appears to be
an attractive transmission scheme in order to over-
come the impairments of wireless broadband chan-
nels. Due to this fact, various standards for wireless
communications using OFDM signals. However, the
main drawback of OFDM signal is the large enve-
lope fluctuations, making the system sensitive to the
nonlinearities introduced by the traveling wave tube
amplifier (TWTA) (A.M., 1981), which cause spec-
tral regrowth in adjacent channels and deformation
of the signal constellation. To reduce nonlinear dis-
tortions, it is necessary to operate the TWTA with a
large power back-off level. However, such amplifica-
tion schemes posses a low power efficiency since the
maximum power efficiency is only attained when the
TWTA is operated near its saturation point. Hence,
TWTA compensation techniques are often necessary
to achieve a trade-off between the linear amplifica-
tion and high power efficiency. Many compensation
schemes based on artificial neural network (Abdulka-
der F., 2002), (Rodriguez N. and R., 2003) to reduce
nonlinearities and their effects have been proposed in
the recent literature.
In most existing techniques, complex models’
input-output measured signals are initially converted
to either a polar or rectangular representation and
then two separate and uncouple real-valued mod-
els are used to estimate the amplitude and phase
output as a function of the input power amplitude.
The real parameters of the two models were ob-
tained during a training procedure based on back-
propagation algorithm and gradient descent method,
but the main disadvantage of these compensation
schemes is their slow convergence speed and ele-
vated requirements of computing resources. Parti-
cle Swarm Optimization (PSO) was originally pro-
posed by J. Kennedy as a simulation of social be-
havior of bird flock, and was initially introduced as
a heuristic optimization method in 1995 (Kennedy,
1995). Recently Sun et al. (Sun J. and W., 2004) pro-
posed a global convergence-guaranteed search tech-
nique called quantum-behaved particle swarm opti-
mization algorithm (QPSO), whose performance is
superior to the standard PSO. The proposed QPSO
algorithm, kept to the philosophy of PSO, is based
on Delta potential well and depicted only with the
position vector without velocity vector, which is a
simpler algorithm. The results show that QPSO per-
forms better than standard PSO on several benchmark
test functions and is a promising algorithm due to its
global convergence guaranteed characteristic. In this
575
Rodriguez N., Cubillos C. and Duran O. (2008).
MULTIPLICATIVE NEURAL NETWORK WITH SWARM INTELLIGENCE FOR MULTICARRIER TRANSMITTER.
In Proceedings of the Tenth International Conference on Enterprise Information Systems - AIDSS, pages 575-578
DOI: 10.5220/0001716305750578
Copyright
c
SciTePress