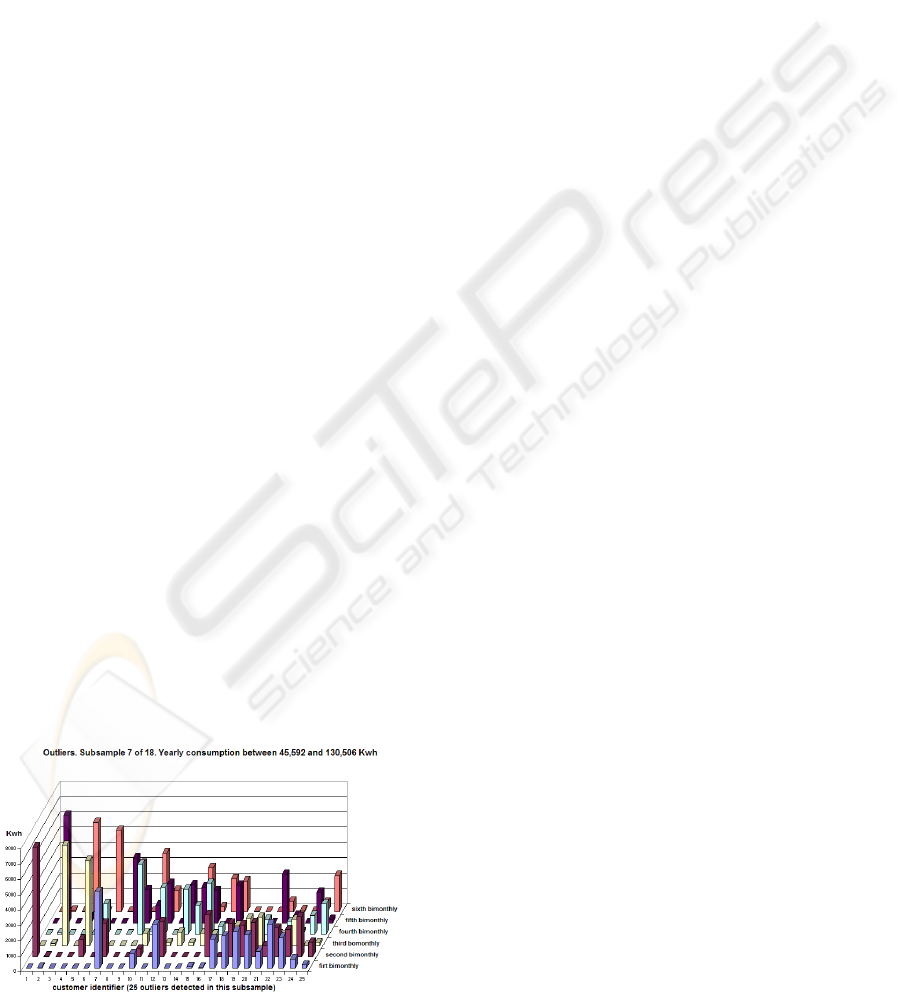
6 SECOND EXAMPLE: LODGING
SECTOR
This new example analyzes 4047 customers from the
lodging sector in Andaluca (Spain). They have a
yearly electric consumption between 0 and 12 ∗ 10
4
Kwh and an extensive contract power range. This in-
consistent sample is divided into 18 subsamples with
similar yearly consumption. Then, general methodol-
ogy is applied, independently, to each one of subsam-
ples.
Customers classified as outliers, based on each
threshold of each subsample, are analyzed in order to
classify them as:
• group 1: Possibly incorrect or fraudulent (due, for
example, to an anomaly in measurement equip-
ment or a fraudulent loss of invoiced energy)
• group 2: Possibly correct, different from the re-
maining data, but not fraudulent and without mea-
surement errors.
For example, the consumption patterns of outliers,
referring to the subsample 7 of 18, are shown in Fig-
ure 3.
In the lodging sector, the use of new sources of
information, as the power factor or the ’quality’ of
the contract, are necessary to distinguish group one
and group two. The experienced Endesa staff has
checked the general database information, referring
to the group of selected customers (6 private cus-
tomers and 35 lodging sector customers, see Table 1)
by means of a manual task. A specific inspection cam-
paign is included in this selected subgroup.
7 DISCUSSION AND RESULTS
The nature of the problem suggests an unsupervised
mining method. There is no evidence of the num-
ber of anomalies or fraud in customer data bases, be-
Figure 3: Some outliers consumption patterns in lodging
sector.
cause all customers are not inspected. Thus, there is
no evidence of the consumption range on anomalous
or fraudulent customers percentage.
This methodology is general and not bound to a
particular set variables or customer type. The whole
input information needed is taken exclusively from
the general customers’ database. The methodology
has been applied to two different types of users (see
Table 1), and it is now being integrated in a global
customer service, described below:
1. First step. In the proposed mining method, a cus-
tomer’s consumption is compared with the other
customers in the same sample. Similar consump-
tion habits are expected. Only data of bills are
used. We have selected the most relevant outliers
in both samples.
2. Second step. In this point we use contract
database and other data informations, different of
bills (i.e.,read consumption data). The method
supposes that a customer’s consumption habits are
similar under the period of study. We reject, in this
step, customers with a high number of unreliable
readings, customers who have initiated, changed
or canceled their contract in the period of study
and simple abnormalities so obvious: customers
with zero or very low consumption.
3. Third step. Endesa staff have analyzed and in-
spected the ’relevant’ customers. Customers that
Endesa staff are often interested in, include cus-
tomers with long-term high consumption and a
geographical criteria.
In this study, the (customers detected, selected and
inspected)/(anomalous customers) percentage had
reached up to 50%.
The confidence level is high, but the support level,
the percentage of transactions from a transaction data
base that the study satisfies, should be improved. So,
one of the main task in our future research lines is
to analyze and include new sources of information (as
the power factor) in our model. On the other hand, the
customer consumption variability appears as interest-
ing input to current data mining tools, as Bayesian
networks, decision trees, neural networks and other
supervised methods (Kirkos et al., 2007), (Editorial,
2006).
ACKNOWLEDGEMENTS
We would like to thank the initiative and collabora-
tion of Endesa, in particular Tom
´
as Bl
´
azquez, Ignacio
Cuesta, Jes
´
us Ochoa, Miguel Angel L
´
opez and Fran-
cisco Godoy.
A DATA MINING METHOD BASED ON THE VARIABILITY OF THE CUSTOMER CONSUMPTION - A Special
Application on Electric Utility Companies
373