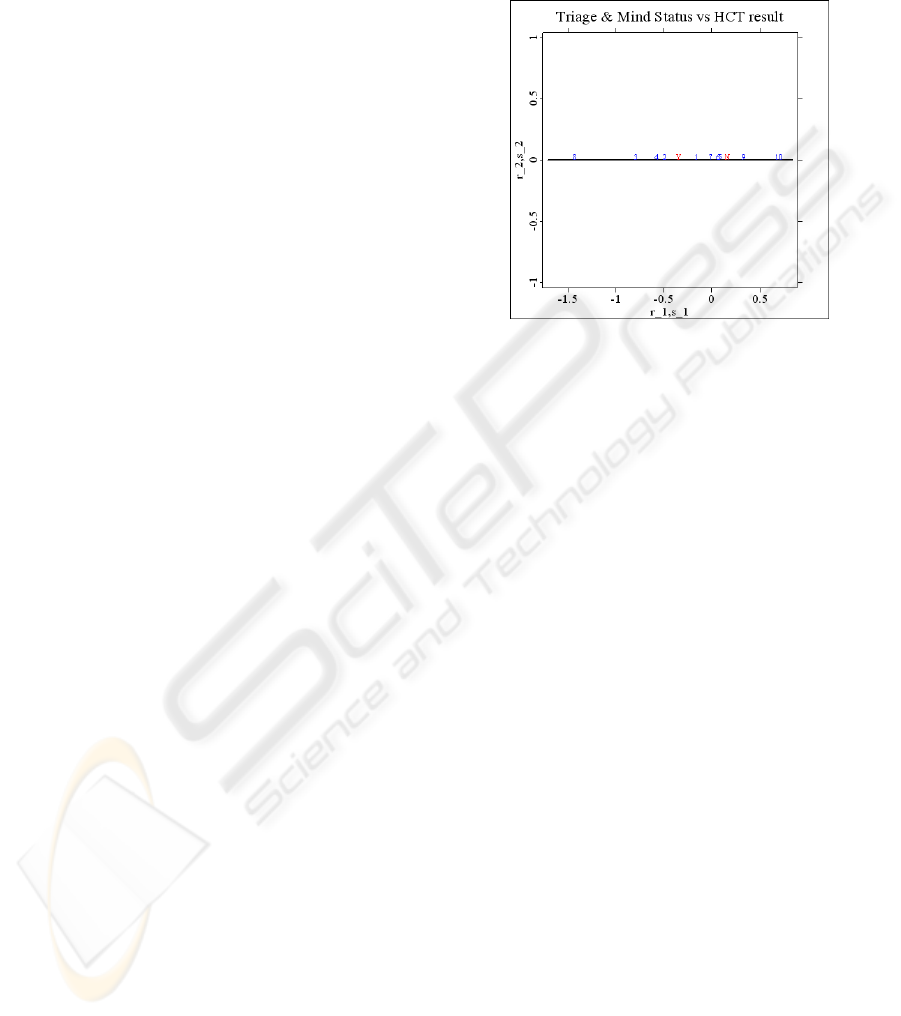
problem, the single rule of ( if A5=2, D=2 )is
applied to the remainder.
It is worth noting that the single variable rule, the
shortest Euclidean distance between the levels of the
independent and the response variables is chosen as
the classification rule; whereas, in the two-variable
rule, the levels of the composite formed by the two
variables on each side of the response variable is
chosen as the composite rule. These principles are
true because in the single-variable case, once the
main classification rule has been set, its complement
(congruent) is automatically determined. For
example, in the case of A5 and D, as shown in
Figure 2, once the main rule, if (A5=2) then D=2, is
set, its complement, if (A5 ≠ 2) then D=1, is
automatically determined. Note that (A5≠ 2) means
that (A5=1 or 3). Therefore, once the destiny of
(A5=2) is determined as D=2, the other choices of
A5 have a pre-determined result. Thus, a reasonable
choice for the classification rule can be based on the
shortest linkage between the levels from the
independent and the response variables.
If the same argument is followed in the two-
variable case, then there is only one level in the
composite variable A9 which can be associated with
one of the two levels of D. The other values of A9
will be assigned with the alternative value of D, a
procedure which does not make good sense since the
other values are not necessarily exclusive with the
chosen value in the main rule. To clarify this point,
an illustrative example is given as follows. If the
shortest distance between level 5 of A9 and level 2
of D is chosen as the classification rule, then by the
same argument in single-variable, level 5 of A9
should be associated with level 2 of D and other
values (these include level 9, of course) of A9
should be with level 1 of D. However, Figure 5
clearly shows that level 9 of A9 should be associated
with level 2 of D since it is closely associated with
level 2 of D by the interpretation of correspondence
analysis (Hardle and Simar, 2003). Thus, the only
reasonable classification rule is to divide the levels
of the composite variable into three regions with the
levels of D as the demarcation points. With the
levels in the middle region undecided, the levels in
the left region are associated with the left
demarcation point; whereas, the levels in the right
region are assigned to the right extremity. Note also
that the levels in the middle region can be classified
later by the rule derived from the single variable.
The optimum correct classification rate by these
two-variable classification rules in addition to the
single rule is 0.76562, with
12
n =2 and
21
n =43. A
slightly better result is achieved than from the single
variable rule where the correct classification rate is
0.75521, with
12
n =0 and
21
n =47.
Figure 5: Biplot of variables A9 and D.
By examining the two misclassifications of
12
n ,
one finds an additional rule to eliminate
12
n : When
(A3=1, A4>=3, A6=3) then D=2. This means that
when the triage level is 1, the mental status is ‘to
pain’ or ‘coma’, and the diastolic blood pressure is
above 110 mm Hg (very serious high blood
pressure), the patient should not be administered
HCT because the situation is probably too dangerous.
This is a special provision under the rule of stating
that when (A3=1, A4>=3) then D=1, thereby
indicating the importance of abnormally high
diastolic blood pressure, a strong indicator to
overrule the HCT decision under serious health
conditions.
At this point, the correct classification rate is
0.77604, with
12
n =0 and
21
n =43. Note that
21
n
means the number of misclassified members,
thereby these members are treated as not
administering HCT (D=2) when in fact they need for
administering HCT (D=1). Misclassifying D=1 as
D=2 is more serious than that of D=2 as D=1 since
the penalty for the former error is life or death;
whereas, the consequence of the latter is merely a
waste of CT resource utilization. Note that of 192
patients only 48 patients were classified as D=1;
moreover, of these 48, the classification was correct
only five times. Since correct classification rate for
the 48 patients was very low, it is worthwhile to
investigate why
21
n cannot be reduced. By
examining the sorted data of
21
n =43, one notices
M
283
MULTIVARIATE TECHNIQUE FOR CLASSIFICATION RULE SEARCHING - Exemplieied by CT Data of Patient