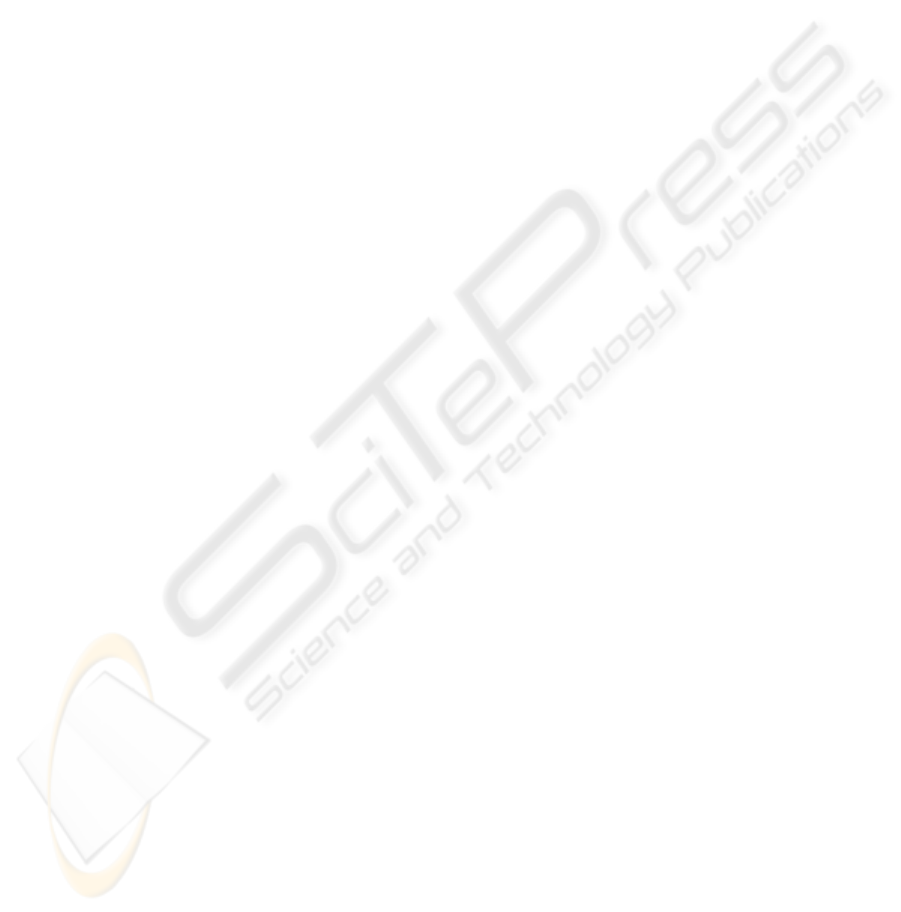
fixed distance ∆ of 120cm on both axes. Such choice for ∆ was driven by the need to
find a suitable compromise between the accuracy required by common indoor location
services, that would ask for denser tags grids, and the operational costs of the location
infrastructure, that increase when deploying thicker tag distributions.
The portable RFID reader, an Identec i-Card3 PCMCIA reader, was mounted on
a laptop PC programmed to run LEPI and was moved on 32 positions, spanning the
whole grid. The i-Card3 reader can generate interrogations with different values of RF
transmission power, ranging between −60dbm and −10dbm. In our implementation of
LEPI, we started to send interrogation messages at the lowest available transmission
power and increased it with a step of 1dbm a time, until either the predefined number k
of near tags was detected or the highest transmission power was reached, as expressed
in the LEPI formulation.
As for LANDMARC operation, we used two i-Port3 fixed RFID readers [11], that
drove 8 antennas positioned at the edges of the grid of Fig.1. Differently from LEPI ap-
proach, LANDMARC is a tag-oriented location approach, thus we performed a further
sequence of interrogations, by placing a RFID tag at the same positions in the grid we
had previously selected to run LEPI, and leaving unaltered the remaining setup.
The positioning accuracy is assessed by determining the location error, defined as
the Euclidean distance between the actual target location, i.e. the position of the RFID
reader, and the one returned by the LEPI algorithm.
The first analysis we carried out is a comparison between LEPI and LANDMARC
approaches, whose results are shown in Fig. 3. The reported curves represent the exper-
imental cumulative distributions of the location errors of LEPI and LANDMARC algo-
rithms, when k = 4 reference tags are selected as near tags and center of mass is used as
the averaging function among near tags. We can observe that, under this configuration,
LEPI attains lower location errors both on the average, with errors of about 130cm ,
and with reference to higher percentages of experiments, like 90% of measurements,
where errors not greater than 200cm are accomplished. Differently, LANDMARC ex-
periences far higher errors, that compromise the use of the estimated positions. Such
results, attained indoors for LANDMARC approach, are consistent with our previous
analysis [2] and testify to the limitations of location methods based on direct RF power
measurements in indoor scenarios. LEPI approach, that leverages on the detection of
incremental proximity information, seems more capable of filtering out ”false near”
reference tags.
A further analysis can be conducted about the optimal number k of near tags that
shall be chosen by LEPI as the closest to the target location. In Fig. 4 we report the ex-
perimental cumulative distribution of location errors obtained when varying k between
1 and 5 and using the center of mass as the weighting function among the selected near
tags. Although the curves intersect in some points within the area of low probabilities,
we still can distinguish an interesting behavior with reference to higher probabilities,
that correspond to a high degree of confidence on the experimental result. If, for in-
stance, we focus on a probability of 0.9, we can notice that the use of either 3, 4 or even
5 near tags results to be effective in terms of location performance, differently from
the cases of either k = 1 or k = 2. In other words, trusting in more reference tags to
estimate the target location seems to allow for a reduction of positioning errors. We in-
49