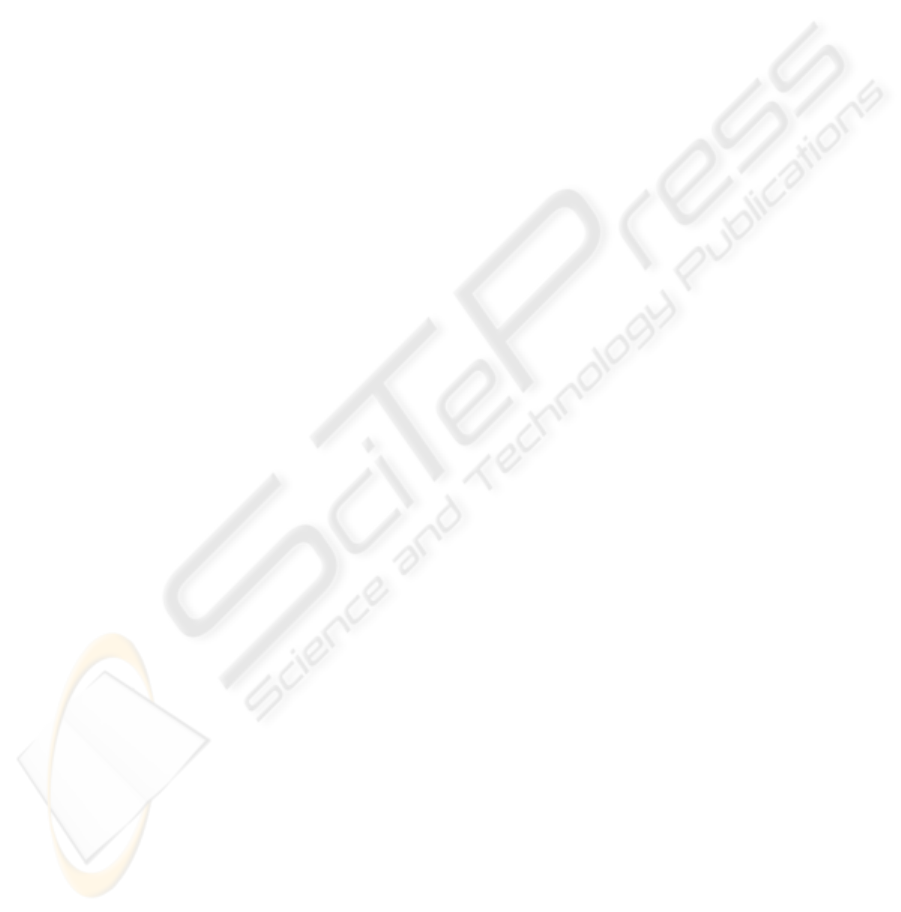
6 Conclusions
The TTS algorithm described in this paper is an iterative process that offers a great
potential for analysing transcribed meetings involving a multi-party conversation. The
study has extended the use of cosine similarity measure to transcribed texts and im-
proved the performance of lexical chaining methods and text segmentation algorithms
by including complex semantic relations and speech specific cue phrases.
Although the evaluation results highlighted the effectiveness of TTS compared to
TextTiling and C99, there are few limitations related to the issue of compound words
and the POS tagging system used. The identification algorithm of compound words
developed in this study, has given, in some situations, unsatisfactory results, as not all
the compound words were the result of combined nouns. Also some compound words
in the corpus such as ‘high voltage line’ and ‘natural language processing’ were not
automatically identified, partly due to the limitation of WMATRIX. Future work will
attempt to resolve these problems.
References
1. Beeferman, D., Berger, A. and Laffety, J.: Text Segmentation Using Exponential Models,
Proceedings of the Proceedings of EMNLP-2 (1997).
2. Beeferman, D., Berger, A. and Laffety, J.: Statistical Models for Text Segmentation, Ma-
chine Learning, Special Issue on Natural Language Processing, Vol. 34, No. 1-3,
(1999)177-210.
3. Bengel, J., Gauch, S., Mittur, E. and Vijayaraghavan, R.: Chattrack: Chat Room Topic
Detection Using Classification, Proceedings of the The 2nd Symposium on Intelligence and
Security Informatics (ISI-2004), Tucson, Arizona, (2004) 266-277.
4. Bilan, Z. and Nakagawa, M.: Segmentation of On-line Handwritten Japanese Text of Arbi-
trary Line Direction by a Neural Network for Improving Text Recognition Proceedings of
the Proceedings of the Eighth International Conference on Document Analysis and Recog-
nition, (2005)157 - 161.
5. Chibelushi, C.: Text Mining for Meeting Transcripts Analysis to Support Decision Man-
agement, PhD thesis, Staffordshire University (2008).
6. Chibelushi, C., Sharp, B. and Salter, A.: Transcripts Segmentation Using Cosine Similarity
Measure, In: B. Sharp (ed.), Proceedings of the Proceedings of 2nd International Work-
shop on Natural Language Understanding and Cognitive Science (NLUCS2005) Collo-
cated with ICEIS-2005, Miami, USA (2005).
7. Choi, F., Wiemer-Hastings, P. and Moore, J.: Latent Semantic Analysis for Text Segmenta-
tion, Proceedings of the Proceedings of the 6th Conference on Empirical Methods in Natu-
ral Language Processing, (2001)109 - 117.
8. Choi, F. Y. Y.: Advances in domain independent linear text segmentation, Proceedings of
the Proceedings of NAACL00, Seattle (2000).
9. Halliday, M. and Hasan, R.: Cohesion in English, Longman, London (1976).
10. Hearst, M.: Multi-paragraph Segmentation of Expository Text, Proceedings of the Proceed-
ings of the 32nd Annual Meeting of the Association for Computational Linguistics, Las
Cruces, New Mexico, (1994)9-16.
11. Hirschberg, J. and Litman, D.: Empirical studies on the Disambiguation of Cue Phrases,
Computational Linguistics, Vol. 19, No. 3, (1993) 501-530.
112