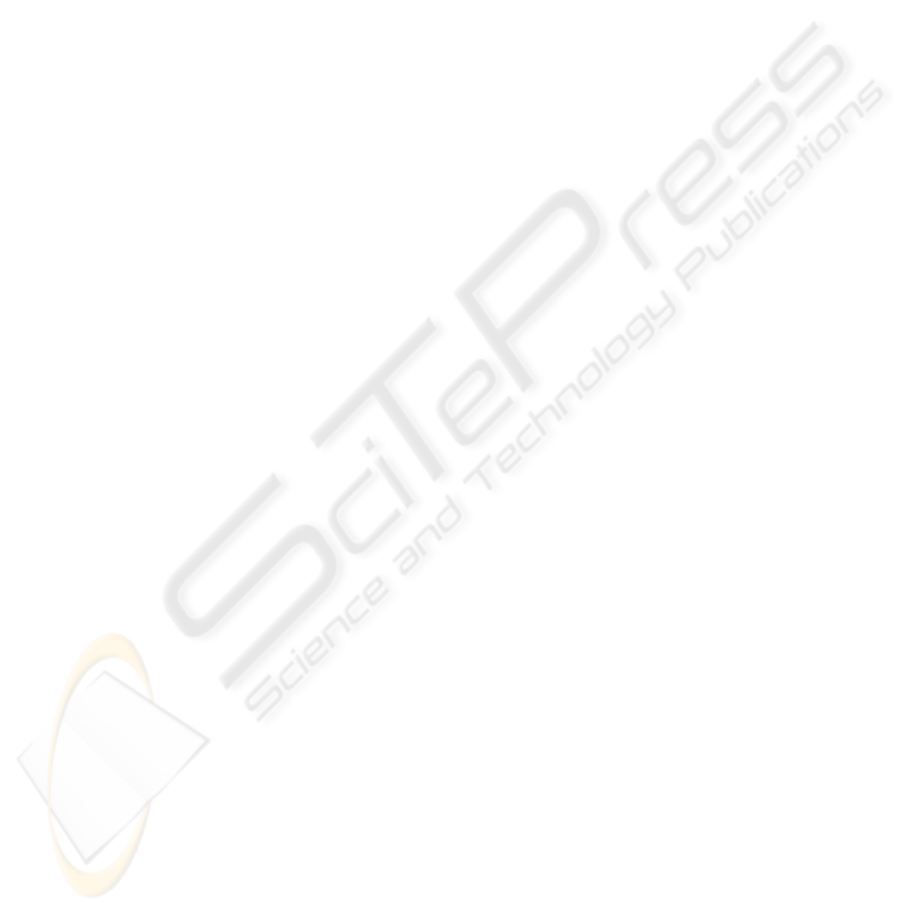
approach. Accordingly, [7] discuss current solutions to RFID security and privacy in
supply chains. The authors propose a security concept which exploits randomized
read access control and thus prevents hostile tracking and man-in-the-middle attacks
that is also suitable for RFID systems with a large number of tags. For future pilot
implementations of our approach we will consider similar security mechanisms.
Our core assumption is that knowing the previous and possibly the current location
of items in the supply chain can help determine their authenticity [8]. This is also
exploited in [9] which explores location-based product authentication in a situation
where only the past locations of products that flow in a supply chain are known. The
solution presented there transforms location-based authentication into a pattern
recognition problem and investigates different solutions based on machine-learning
techniques. The proposed solutions are also studied with computer simulations that
model the flow of genuine and counterfeit products in a generic pharmaceutical
supply chain. The results suggest that machine-learning techniques could be used to
automatically identify suspicious products from the incomplete location information.
However, the level of security of the studied methods, in terms of probability to detect
the clones, is relatively low, nevertheless we draw from the method of conducting a
respective simulation study from their work.
5 Outlook
What we have presented so far is a new approach for detecting counterfeits in an
RFID enabled supply chain using proximity information of items that can be gathered
by EPCIS or similar services. We have presented appropriate data structures and two
different algorithms that implement the concept of proximity based authentication.
We have illustrated the concept with a simple supply chain simulation that
demonstrated the potential benefits of our approach.
At the current point in time, our work is preliminary, since we have made quite a
few assumptions that we still have to prove. For instance, we let the number of checks
in the supply chain increase by a certain response factor that should reflect
countermeasures. We must now validate and check in how far this is really the case in
real anti counterfeiting activities, and most importantly, we must access how such a
response factor would typically differ in various industries. Correspondingly, many of
the simulation parameters presented in
Table 1 are not yet based on real industry
experiences, mostly because of the apparent difficulties to obtain real world data.
The most fundamental assumption we made, however, is that counterfeits really
enter the supply chain in close proximity to each other. Although it makes sense to
expect this to be the case, we cannot currently prove it. We do expect the emergence
of RFID enabled supply chains to provide better transparency and tracing of goods in
the mid term, so that potentially more data will become available on the inclusion
patterns of counterfeits for different supply chains.
In the short term, we will prototypically apply and refine our algorithms and other
anti counterfeiting applications in the context of the EU funded research project SToP
113