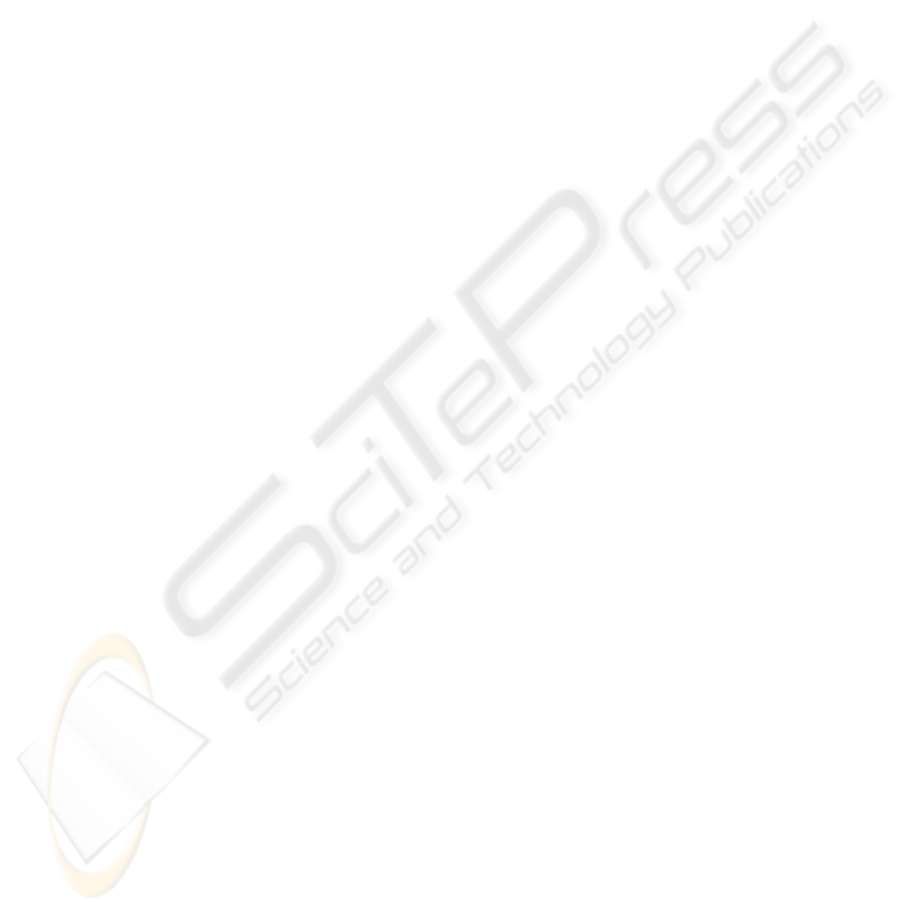
fractal dimension of cubic method and the factor
“desire to purchase quality products” (t = 4.11, df =
8, p < .01).
Table 6 shows a high correlation between the first
factor (desire to purchase quality products) and both
the planar and cubic fractal dimensions, indicating a
negative correlation between the second factor (soft
feeling) and the fractal dimension.
3.5 Evaluation of the Result
The canned beer used to compare labels omits the
one of a famous beer company in Japan. Therefore,
this investigation was done by using the local beer
not notorious.
Result of examining hot seller of beer by the Internet
later. The 33rd canned beers with high fractal
dimension of Fig. 3 were a local beer hot selling
rankings and 1st place.
(http://www.rakuten.co.jp/yonayona/111011/718975/#
tp24)
The 42nd was popular ability No.1.
(http://store.shopping.yahoo.co.jp/yoho-
yonayona/b7dab0e6c2.html)
Moreover, popularity was high, and the 15th and
22nd canned beers had been won the championship
in the contest of the beer.
(http://www.rakuten.co.jp/yagishoten/425762/448054/)
The canned beer with low fractal dimension was not
displayed in sales information in the Internet.
The beer is drunk all over the world. The SD
evaluation that we used is thought to be different in
the culture and the climate. This testee is a young
person in Japan. 45 kinds of canned beers used to
experiment are not brands known well in Japan.
Therefore, the testee had not seen the label of
local beer. In a word, the testee who did not have the
experience of drinking each beer was chosen.
It can be thought that the fractal dimension is related
to the buying intention from the above-mentioned
result.
4 DISCUSSION
Biological information exhibits a chaotic behavior,
and until now, it has been difficult to explain human
information processing using subjective methods
(Miao, Shimoyama, Shimizu, Oyama-Higa,2006),
(Barnsley, M. B., 1988) . In other words, delicate
human information processing, in particular, cannot
be analyzed in detail by traditional linear analytical
methods. By using nonlinear and complexity
methods of facial fractal analysis, our findings
suggest that information processing in human
recognition might be explained and characterized by
fractal dimensions.
We demonstrated that higher fractal dimensions
of canned beer packaging led to an increased
impression of high quality and a greater desire to
purchase. Moreover, the analysis showed that low
fractal dimensions of canned beer packages
contributed to a soft feel. The analysis results
indicated a tendency for the third factor, “light feel,”
to be perceived for low fractal dimensions.
From the calculations of fractal dimensions for
canned beer package photographs and the results of
the SD method, a high correlation was found based
on Pearson's correlation coefficients for the
descriptors “would like to buy,” “delicious-looking,”
and “premium.” Since a significant difference was
detected between the high fractal group and the low
fractal group for the terms “would like to buy,”
“delicious-looking,” and “premium," canned beer
photographs with high fractal dimensions should be
perceived as being more desirable to buy, more
delicious-looking, and of higher premium quality.
Although traditionally, resorting to subjective
assessments such as the SD method used in this
experiment would have been necessary to measure
the good or bad aspects of product packaging, these
results showed the feasibility of calculating the
fractal dimension of such product packaging to
obtain an objective index of such aspects.
Further research is required to gather data on a
variety of product types and a broader range of ages
and subjects most appropriately suited to the
products being examined.
ACKNOWLEDGEMENTS
We thank Mr. Yu Toishi of the Oyama laboratories,
Kwansei Gakuin University which cooperated with
making of experimental data of packaging.
REFERENCES
Barnsley, M. B., 1988. The book, Fractals Everywhere.
Academic Press Inc., London.
Nagamachi, M., 1995. The story of sensitive engineering,
Tokyo, Japan Standards Association.
Jun, S., & Kamahori, F. 2003. Study about food and a
purchase action of life armamentarium of a university
student. Design Studies, 50(5), pp. 31–39.
Mandelbrot, B. 1977. The book, Fractals: Form, Chance
and Dimension. W. H. Freeman & Co., San Francisco.
RELATIONSHIP BETWEEN FRACTAL DIMENSION AND SENSITIVITY INDEX OF PRODUCT PACKAGING
353