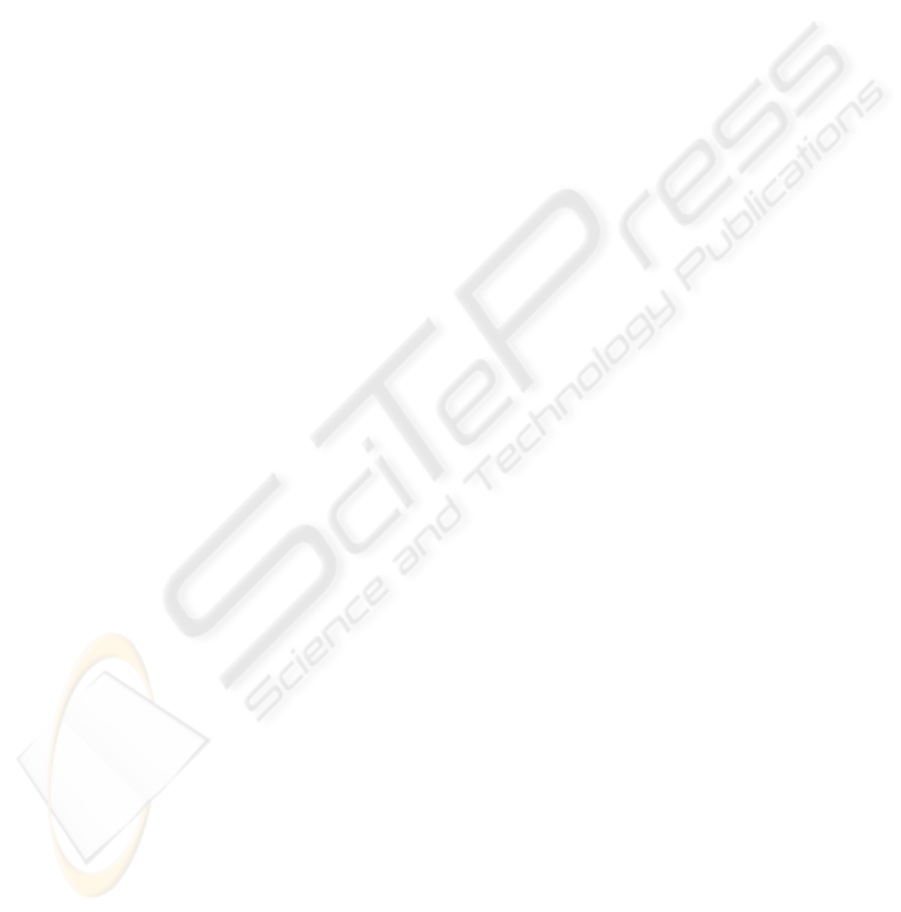
and increase the revenue on existing clients by
offering new products and services. The targeted
campaigns usually fall in the domain of analytical
Customer Relationship Management (CRM)
systems.
However, similar to the data quality
requirements specified by the Basel II Capital
Accord, CRM initiatives require that large quantities
of analytical data, containing financial and
demographic information aggregated on the
customer level are of sufficient data quality.
Therefore, these two major business drivers in
the banking community can be severely affected by
poor data quality. In this paper we will discuss the
impact of most common problems encountered, and
suggest a complete framework for improving data
quality.
2 IMPACT OF DATA QUALITY
When dealing with data quality issues while
preparing historical data for creation of internal
rating models in accordance with Basel II
guidelines, as well as targeted marketing campaigns
and retention models for CRM initiatives, one most
frequently encounters the following problems
(Nadinic 2006):
multiple names for the same entity
missing values
incorrect values
duplicate records for the same customer
These problems affect the model creation and
reporting processes in several ways. Multiple names
for the same entity (usually a name of a customer,
organization or a product type) prevent the
aggregation of data on a customer level, while
giving a false idea about the actual number of
customer or organization/product types.
Predictive model development is affected by
missing values and multiple names in the following
way (Nadinic 2006):
If missing values in relevant fields are
treated as a separate category, they may
affect the accuracy by grouping the
characteristics of high-risk and low-risk
clients in the same category. This is also
valid for multiple names where specific
incorrect values can be treated as a separate
category in the modelling process
Multiple names for education levels,
organization and employment information
about the customer can lead to overtraining
of the model on the training set if the
number of defaults (and therefore the
training set) is low. In this way, when the
created model is used for actual scoring of
the customers, the model will not identify
the correct probability of default (and
therefore rating class) to the customer
Creation of marketing campaigns is mostly
affected by incorrect address fields of the customer
which prevents contracting the customer through
different sale channels, and incorrect and missing
information about demographic and financial data
which prevent segmentation and creation of
customer churn models.
Duplicate records about the same customer may
result in repeated offerings/contacts to the customer
and can prevent identification of the profitable ones.
These data quality issues can reduce the
effectiveness of created internal rating models, while
in the case of CRM initiatives, they cause substantial
financial losses.
3 PROPOSED SOLUTION
The proposed framework for data quality monitoring
and improvement is divided into several distinct
phases:
Data quality analysis through operational
and strategic data quality indicators
Standardization
Data cleaning according to business rules
and constraints
De-duplication and creation of “best
records” for each customer
Data quality assurance on data entry
It has to be noticed that all data quality activities
should be performed on the organizational level by a
group of dedicated employees, thus providing a
framework for total data management.
3.1 Data Quality Analysis
Data quality analysis (data profiling) is the analysis
of fields and tables in search of interesting
information (Ericson 2003). Therefore, we propose
to formalize this approach by creating two types of
information indicators:
Operational data quality indicators
Strategic data quality indicators
ICSOFT 2008 - International Conference on Software and Data Technologies
328